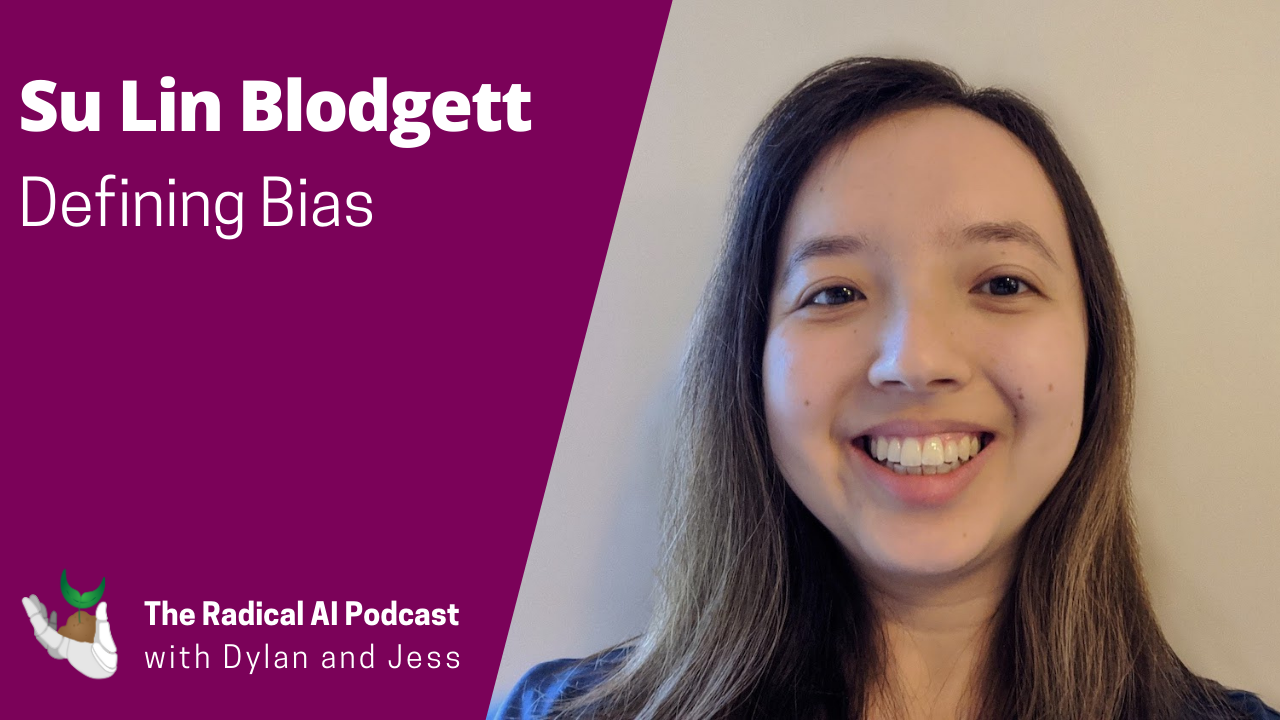
How do we define bias? Is all bias the same? Is it possible to eliminate bias completely in our AI systems? Should we even try?
To answer these questions and more we welcome to the show Su Lin Blodgett.
Su Lin is a postdoctoral researcher in the Fairness, Accountability, Transparency, and Ethics (FATE) group at Microsoft Research Montréal. She is broadly interested in examining the social implications of Natural Language Processing, or NLP technologies, and in using NLP approaches to examine language variation and change. She previously completed her Ph.D. in computer science at the University of Massachusetts Amherst.
Follow Su Lin on Twitter @sulin_blodgett
Email Su Lin at sulin [dot] blodgett [at] microsoft [dot] com
If you enjoy this episode please make sure to subscribe, submit a rating and review, and connect with us on twitter at @radicalaipod.
Transcript
su lin_mixdown.mp3: Audio automatically transcribed by Sonix
su lin_mixdown.mp3: this mp3 audio file was automatically transcribed by Sonix with the best speech-to-text algorithms. This transcript may contain errors.
Welcome to Radical A.I.,
Speaker1:
A podcast about technology, power society and what it means to be human in the age of information, we are your hosts, Dylan and Jess. And in this episode, we interviewed Dr. Sue Lynn Blodgett about definitions of bias in natural language processing systems and beyond. How do we define bias? Is all bias the same? How does bias impact the stakeholders of natural language processing systems? Is it possible to eliminate bias completely in our AI systems?
Speaker2:
Should we sue Lynn Blodgett as a postdoctoral researcher in the fairness, accountability, transparency and ethics, otherwise known as Fate Group at Microsoft Research Montreal, she has broadly interested in examining the social implications of natural language processing or NLP technologies, and in using NLP approaches to examine language variation and change. Sulin previously completed her PhD in computer science at the University of Massachusetts, Amherst.
Speaker1:
This was a really exciting conversation for us to have because last summer's in the summer of 2020, we had the opportunity to interview Dr Emily Bender, who is an amazing scholar in the field of natural language processing, and we had an episode with her on basically the 101 of NLP ethics. And so we see this conversation as a continuation of that dialogue where we dive a little bit deeper into some of those topics that we brought up with Emily, especially on this topic of bias. And there's a lot of really interesting stuff here. So we are so excited to share this conversation with Dr. Sue Lynn Blodgett with all of you. Today, we are on the line with Sue Lynn Blodgett, Sue Lynn, welcome to the show.
Speaker3:
Thank you so much for having me.
Speaker1:
Cause and today our topic of interest is bias and the power of definitions and language. So why don't we kick it off with our first question for you, Sue Lynn, which is, in your own words, how do you define the word bias?
Speaker3:
Oh, man, what an impossible and good question. So for me, there is no single definition of this, and the MLP community has a purpose in a lot of different ways. So maybe it would be helpful for me to illustrate some of those. So. So first, I should say, before I get to thinking about this, a lot of work has emerged in the community thinking about this in the last few years. So even though we have a long way to go, I want to acknowledge all the work that people are putting into this right now and to say it's really exciting to see significant emerging awareness of, quote unquote, bias and NLB. So for me, what I'm seeing is that in many ways, in normal people, life has come to refer really broadly to this undesirable system of behaviors. And what you find when you dig in is that researchers and practitioners actually really mean a whole bunch of different things when they say bias. And I'll give some examples. So in, for example, in sentiment analysis, where the goal is to predict the sentiment of a piece of text, for example, if it's positive or negative or neutral. Researchers have found that systems give different predictions for sentences with names associated with different genders.
Speaker3:
So, for example, Amanda feels anxious because Adam feels anxious and the same, and they get different predictions for how positive they are even when they really shouldn't, because they are basically the same sentence in the same is true for names associated with being African American versus European American. So elsewhere, toxicity detection systems, where the goal is to predict whether a piece of text is toxic or offensive. Yesterday, three sentences I mentioned, disability is toxic even when they shouldn't. So, for example, the sentence I am a deaf person or I'm a person of mental illness gets high toxicity scores or even though they're obviously completely not toxic sentences, automatic captioning systems exhibit higher errors, people speaking and accents or language varieties that aren't mainstream English, for example, speaking speakers from Scotland. And one last example, and these are all awful examples, but this one is particularly awful. Some large state of the art language models actually produce very harmful ideas about different people. So it's been shown that when given prompts referring to Muslims DVD, you almost always refer generates text talking about terrorism and when given prompts, referring to, say, a transgender woman. We've seen a general text talking about how she's not really a woman. So I think all these examples are sort of illustrative because we can see that when we as a field say bias, we really are referring to quite a wide range of different system behaviors.
Speaker3:
And really because language is connected to identity and our social world in so many ways. Right. This comes out and how we think about bias. So sometimes we're talking about issues and how NLB systems treat language about or describing different people, for example, horrifically Islamophobic or transphobia ideas. Sometimes we're thinking about and LP systems treat names associated with different groups. Sometimes we're thinking of a final peace systems, treat language produced by different groups of people. Right. Like with the automatic captioning, sometimes we're concerned. Sometimes bias means a system is exhibiting different error rates for different kinds of language. Sometimes bias seems to mean that we are treating certain kinds of language differently. So, however positive my system thinks, Adam is anxious as we're giving a different prediction. Amanda is anxious. Sometimes it means that systems are just producing certain kinds of language at all that we think are harmful. So. So I think bias has come to kind of encompass a whole wide range of kind of different things, you know, certain behaviours, properties that we find undesirable.
Speaker2:
So I'm curious. Why this matters, right, so we talk about bias, right? I'm not challenging that this matters, but I'm just I'm curious from your perspective. Right. Like how we talk about bias obviously changes how we might implement certain design decisions around that bias. But from your perspective, like why why is this, you know, what you research and why does this really matter out in the world?
Speaker3:
Right. Right. That's a really great that's an important question. So from my perspective, the language that we that we use to describe things really helps is important because it shapes how we think about race and address these things. I think there's two places this happens, right? One, being really precise about what we think is harmful helps us reason about the harms more. Right. So just in terms of what we want, model behavior like what we think is harmful, who might be harmed. Right. The more precise we can be, the more kind of carefully we can reason about these. And it also helps because the more precise we are, the more able to draw on all this work outside. And that has thought about these things for a long time. Right. So language is involved in our social world. Right. And it's deeply like these characters are really deep and people have thought about these for a very long time. So the more careful we are, the more precise we are about these mechanisms, the more we can also draw on all this work. And also the more precise we are, the better we can think about how we are measuring or mitigating these things. Right. I mean, you say racial bias training doesn't really help you think about how good is the way you know is the way that I'm measuring racial bias. Really what I'm after is the mitigation really what I'm after? Are there aspects of this that I'm not capturing effectively through my choice of measurement? And I think being very precise and saying I after stereotyping, I'm after just performance differences, I'm after the erasure of certain topics or perspectives I like that allows you to be quite precise about, like normatively what you think the harm is and also how good of a job you think you're doing at measuring or mitigating.
Speaker1:
You keep using the word measuring, which is something that and I've been focusing on a lot lately. We have a new series called Measure Mentality. And so we were thinking about this in the context of bias before this interview.
Speaker3:
Oh, that's awesome.
Speaker1:
Thank you. Yeah, you should really check it out. Also, other listeners, shameless plug. Yes. Yes. But something that I'm wondering with the measurement of bias, because you are talking about the importance of precision and, you know, I guess somewhat standardization and a common definition. And so I'm wondering, do you think that first it is, is it possible for there to be a common definition for something like Bias and LPI? And if so, is that good or bad?
Speaker3:
That's a good question. And I think there's a couple of things. There's a couple of things I want to draw out from that. So one is that I don't think we need a single way to describe bias or a consensus, because what we're finding right, when you dig into all the way people think about bias, is that what you know? Is that the language we need more precise language or any language that is more precise and biased to describe each of these things because they're about different groups of people, different kinds of language, different kinds of model behavior that we're worried about. You know how to specify. Right. So this variety should encourage us to be more granular and more precise rather than to come up with one single overarching way to describe all of it at the same time, because that's just not possible. Right. Social groups and are not exchangeable by the different kinds of people, in my experience, are not really the same. And so we should have the vocabulary right. The way to think about that that are not just bias, but at the same time, I think it is this measurement aspect.
Speaker3:
Right. Is useful. And there are things that we can draw and kind of unify and how we talk about this. For example, one thing that I do a lot in my work is to draw on kind of framework of measurement modeling from quantitative social sciences. Right. Which offers some language for carefully thinking about. It's really like a framework for thinking about what it is you want to measure. So in this case, we're worried about bias or harms and how good of a job are to begin measuring it. And so this doesn't really say this is the one way to measure bias. This is the one way to think about bias. But it does offer you a language for very carefully thinking through what it is that you're after and how good a job you are capturing what it is that you're after. Right. This kind of vocabulary, I can help us as we're publishing these approaches that are trying to put them in practice, like really compare them against each other and have this kind of shared vocabulary in a useful way.
Speaker2:
There is this idea that was in vogue a few years back and maybe still is that bias is bad. And therefore the goal of our algorithms should be to mitigate that bias. Or if we can just get rid of that bias completely, like let's make our algorithms have zero bias in them. And I'm wondering what you think of that argument, whether it's possible or whether that should be how we're thinking about bias at all.
Speaker3:
Yeah. So first I'll say yeah, that I think it's thinking about bias and what the goal of our work maybe is. Biasing or removing bias is probably not super fruitful because if we see there's so many different harms, the systems can give rise to different issues, this information. Tries to write that thinking about biases like a single thing can't possibly capture them, all right, and maybe lulls us into a kind of a false sense of complacency about how good of a job or doing or because we want these to be sort of iterative process that people always want to be on the lookout for these things. I also think there's like the way we framed bias sometimes is also kind of misses out on some things that we're not capturing really well that we might care about. So I think one thing that's true about bias is that it often kind of locates like problems at the point of decision making. Right. For a system. Right. So all of the examples that I gave are issues of the system at the decision making time, the analysis that looks at how a sentiment analysis system is treating different kinds of language. And sometimes when we say we want to there's bias in the model or bias in the system, we want to remove that bias. We're really not thinking about questions like this is like this mode of analysis doesn't really help you understand how these different decisions actually impact people like who is actually harming the system is deployed. Right. How do people's lives change? So thing you are devising a model in some sense, like almost I think we risk like this. It isn't guaranteed to lead to this, but I think it risks this mode of analysis where you don't really think about what the harms are to people. And I can try to give some examples of those things where I think those, if you're interested, I think the way those give rise to different questions, then just like, you know, questions about at the point of decision making,
Speaker2:
You feel free to to share those examples. I think that would be great.
Speaker3:
Yeah. Yeah. So one fun one is for speech recognition in in cars. So we know that speech recognition systems work less well for people with higher pitched voices. And carmakers have actually acknowledged this. So it's speech recognition and cars don't tend not to work for women as well as for men. And I was looking this up. I found one proposed solution from carmakers. I quote, Many issues of women's voices could be fixed if female drivers were willing to sit through lengthy training. Women could be taught to speak louder and direct their voices towards the microphone, which is both insulting but also could be catastrophic. If, like your voice recognition system in your car doesn't work when it's supposed to go like you think about this, right. Or we've also seen examples. Right, where and systems are increasingly used in, for example, hiring contexts. So you can imagine risibly filtering systems, but also perhaps like interview like situations where your speech perhaps is being evaluated. Right. And I think there are questions of what are the impacts on different groups of people. Right, when these systems are being deployed. And I also think I think one of the example that I like a lot is this example of toxicity detection systems, again, in which we know they train mentions of disability as disproportionately toxic. And we also know that they also these same systems treat minorities varieties of English as more toxic than mainstream U.S. English. So I think, one, if you step away from thinking about just the system output and torse thinking about what the consequences of the system development are, you can think of actually quite a few things might happen right when this is used in content moderation systems.
Speaker3:
Right. When there's like the you know, if you're a person writing about disability or a person writing in a minorities' language of variety. Right. There's like the indignity and frustration of maybe you're like your post got deleted, right. Like very often. Right. So there's an immediate frustration. And there's like the accumulated impacts of these things. Right. Like how does disproportionate removal of the toxicity of disability rate impact public discourse or how does it, a, make it feel like, you know, reproduce ideas that disability is not maybe an appropriate topic for public discourse, maybe on social media platforms? How does it impact people to ability to talk about these kinds of things online in a way, in a free way? Right. If these things are disproportionately removed, how does it affect, uh, efforts towards, for example, like public recognition of like disability rights? Right. And like, you know, efforts towards legislation and so something so there are kind of multiple kinds of harm. That's not just the system doesn't work well for me, but but also there's a dignitary harm of the system doesn't work well for me. There is like the public participation sort of harm that like other people can't see what I write. Right. And we are reproducing ideas about what kinds of language, what kinds of topics are acceptable and available for public discourse. And so and these are important because they reproduce very old hierarchies. Right. So there are very old hierarchies that that do value. Minorities are non-standard varieties of English, for example, and we risk kind of reproducing these exact same, um, hierarchies that that continue to do value these ways of producing language.
Speaker2:
Would you be willing to talk more about toxicity systems? Content moderation has been in the news a lot recently, and I'm just curious about how these toxicity systems work, especially through in an LP and I guess MLP biased perspective.
Speaker3:
Yeah. So I don't I, I should say, just by way of disclaimer, like my my main area I don't work on. Toxicities, I'm something I'm familiar with some of the work on kind of biases or harms kind of possibly arising from toxicity detection systems, but I don't really I don't work with them. But I do think they are super important, um, in part because the waste the toxicity systems that are likely encountered by most consumers, the most users as parts of moderation systems online are actually pretty opaque to researchers. So we have some idea of how it is that they probably work. But, you know, we don't they're they're they're largely black boxes. Right. So we can only kind of examine the outputs. So I guess I'll say a couple of things. One is that these systems, like a lot of other MLP systems, are, it's a supervised task. Right. So they are trained in a way such that you gather a large dataset of language that you think is toxic or abusive or offensive. And these definitions do vary. And there are consequences to these different definitions of what we think is a common language. But largely, you gather this data. It is often it is usually annotated. So perhaps by ground workers, it's like one common paradigm, right, for whether or not this language is hateful or abusive or toxic. And then you train systems on this text. There are a lot of I think one thing that's really important and interesting to me is that there are tons of design decisions. Right. That go into this process that really affect the outcome. For example, if you you know, how do you get the data set that you're trading on in the first? How do you just you know, if you say I need a data set of abusive or toxic or hateful language, how do you get this one way you can imagine? One way to do this is by keyword searches, right? By the sort of like limits what you can train your model on to things that are like very overtly hateful in ways that you already know about.
Speaker3:
You could ask for things that people have previously reported perhaps as hateful. Right. But this might also constrain what you get into something in some way. Right. And it's maybe dependent on what people have reported and what the importance of the larger content moderation system are. And so all of these things kind of have, you know, these things. And also if your annotators are and what their experiences are, will affect this. One other thing that's useful to know is that, um, there are I leaders this when I mentioned definitions of toxicity or hate speech or whatever matter a lot, and they do because harmful language on social media platforms or other places can take a lot of different forms. And so what you consider within the purview of your system. Right. Changes assessment system. And that's important to think about. So most systems consider very overtly hateful language use of slurs. Things like microaggression are really hard, both because it's hard to get a data set of them. It's just there's just subtle language, right? It's much more difficult to just pinpoint them as hateful things. There are other things that are very like sort of forum dependent or location dependent. So very in general, like talking about like like bodies is not harmful, but like some like maybe like forums dedicated to like survivors of eating disorders.
Speaker3:
It is harmful. Right. And that's prohibited. OK, so that's a very specific um. So all of these things contribute to the challenges of developing appropriate and effective toxicity systems. And I think the last thing I want to say is that I think it's really important to think about the systems in the context of the larger context, larger systems that they form a part of. Right. So there's like there's usually like a larger kind of moderation system of which the MLP portion is only like a small portion. Right. There's like some model that does something but that operates as part of a larger system that can look like a lot of different things. Users have many different ways of reporting, hate speech. There are lots of actions user might be able to take. Right. Like there might be the consequences you might choose for, you know, for people are different. Right? Sometimes the decision is I'm going to delete hateful posts. But if the decision is I'm just going to continue for a while or posts, you know, so like there's it's how appropriate or like a good a job, a toxicity system does also has to be considered like kind of in the context of like whatever kind of holistically whatever this larger system is. And I think that's like that's also kind of like a mode of analysis that, um, that maybe we underutilizing MLP, but not, for example, in HCI, in social computing, where folks have been thinking about these things for quite a long time.
Speaker1:
I've been having some conversations recently about A.I. systems that exist in very complex environments and whether or not they're actually useful. And so when you're talking about toxicity, I'm thinking about like ground truth labels and how it's very difficult to make an AI system when there is no ground truth to go off of. And one example that comes to mind for me of this is trying to make an AI system that predicts if people are going to be happy because the the data that goes into making that system is. Labeling whether people are happy or not and happiness is such like an arbitrary and contested, you know, thought a word and also experience and so with toxicity, it's also making me think about that, like how how do we even come up with ground truth labels for toxicity? And if we can't, if there's no way to avoid the inherent bias, should those systems exist in the first place, are they still worth it? What do you think?
Speaker3:
Yeah, totally. That is a fantastic question. And I think it is the case for lots of these complicated and multitasks things like toxicity or sentiment, but also things like, you know, is that much more empathetic. You know, this isn't like an appropriate response even. Is this a good summary of something? I think there are a lot of, um, for I think a lot of these tasks or settings, it is almost always going to be the case that people will disagree. Right. Because this is the nature of language. Right. Language like we we like the way we do language very like a rise in our interpretations of other people's language. Right. What we think language means that comes out of all of our it's our lived experiences. Right. Super subjective and contested sometimes. And it varies a lot based on cultural and geographic context, for example. Right. And so there is there I think there's important questions about, um, trying to make, you know, maximally portable or scalable, um, energy solutions, because it's almost always the case that, you know, people will not agree, um, you know, completely if something is toxic or not really depends a lot on context and on your own life experiences. Right. Um, so I think this raises a lot of questions because the NLP pipeline typically sort of does assume like one right answer, one ground truth like label or prediction or something for everything. And so I think this raises not just questions for, you know, how you entertain right now.
Speaker3:
Maybe it's allowed for answers, but also what the problem formulation is to begin with and also how you model it. Right. Are all, you know, do you treat all answers as equally valid, for example? And I think different settings this will might look different, right. For hate speech. You might decide if at least one person thinks it's harmful, maybe just it's harmful. Like, I don't want to quibble and try to figure out who is, you know, perspective is most valid, whether there are other things like, um, I think one, um, really illustrative example from like the recent kind of U.S. context razor, that is a safe phrase. All lives matter. Do you keep this in a forum or not? Right. And it's like, you know, who you know who might be harmed by having this. Right. And like, who is, you know? And so this is a question that's really important because like, um, the perspectives of who is entertaining for versus like harmful or appropriate or something like that matters a lot. And there may be it may be that there is no decision you cannot make. Right. Whether to keep that in your forum or not. Your social media platform. There's probably no decision that will satisfy everybody. Right. Some people will be upset if it's removed and a lot of people will be harmed if it's kept up. Um, and I think what this illustrates, right. Is that, um, I think heretofore I think we've assumed that there is like a obviously right decision or a neutral decision for a lot of these things.
Speaker3:
I think what this illustrates is very starkly is that a lot of the time there is no neutral decision and but you still have to make one. And so the question is like, what processes do you effectively develop? Right. Who's to bring in many perspectives in a meaningful way. Right. To make that decision? Um, but, yeah, I think this is a fantastic question. And I think it raises tons of questions like, you know, like beyond just toxicity for just like, how do we do an LP at all? Right. If all our models assume there's a right answer and there are there are people thinking about this in other settings, for example, in natural language in France. So this is the task. We're given two sentences, right. Is the first until the second. And a lot of people sort of, you know, like don't disagree on some interpretations. Right at the first sentence mentions a woman in the second act is a girl, right. Does that does the first until the second. Oh. So people will say yes and he will say no. Right. But it depends on your world understanding of woman and girl means. Right. So, yes, I think this is an exciting time because there are people starting to think about, like, OK, these disagreements exist because, you know, we live in the world and language is hard and complicated and contextual. Um, how do we rethink and help to account for this one?
Speaker2:
One thing that you mentioned is this, you know, the mythos, that language is neutral. But, you know, what we know is that language is created in like a real time and real place and like a specific context. And there's a whole I mean, language is political to a certain degree, like there's power behind it, even in how it's created. And I'm wondering from your perspective how we, I guess, contend with that. Because now know people are making decisions about what language means and like you're saying, it's weighted in certain ways. And so I'm wondering if there's a power analysis of all this and how do we make sure that there's, like, either inequality of voices represented or at least a diversity of voices represented in that description or creation of power?
Speaker3:
Yeah. So people have outside of not have thought about this for a long time. So, you know, I think particularly a linguistic anthropologist, but also a lot of discipline, a social linguists, educators. And of course, like folks, you know, who's sort of like existences. Right. Like, you know, sort of by nature, like require this kind of power analysis. So, you know, like indigenous folks or black community, as I have and in thinking about language, have thought about these things and written about them for a long time. And this is where I think l.p, I think could benefit a lot from drawing from all of this thinking. And so I think I think there is considerable evidence that who gets to control languages really is a function of power. And so Jane Hill, who wrote this wonderful book, The Everyday Language of White Racism, one of one of the examples that she brings up is kind of controversies over the naming of a kind of offensive naming of certain landmarks. Right. So there was one landmark. There was like one mountain that had a very like a name that was very offensive. And I won't say it to like the indigenous people living there, but it had been used a long time by like the white residents. And so it's like this controversy erupted.
Speaker3:
And what Jane, you know, what Dr. Hill really showed is that that, uh, there was considerable resistance to relinquishing kind of control over the naming of this thing that like and there are all kinds of arguments were proffered like, oh, you know, we always had this like sort of an offensive name, like, you know, but all these kinds of things. But really what it came down to is right. Like, um, we don't want anybody to tell us that we can't call it this. Right. And and in this case, like, you know, the the choice of like who gets to decide, like, what this feature was called was really a question about who gets to control language. Right. And that has always in the United States history and I think like globally. Right. Place white people at the top. Yeah. And so definitely I think, uh, and like if for an AP systems there is kind of emerging, we're thinking about how help systems also kind of shift power in the sense that they do kind of participate in in several ways. One, that they produce ideas about people write in languages. Right. And those are important because those kind of support, you know, the distributions of power and resources. Right. I can say more about that. I think another is like, for example, if your matri machine translation system or, you know, Google Maps has particular names of particular landmarks.
Speaker3:
Right. In some sense like that almost controls like what those things are called, because this is what a lot of people who go to a new place will see. And so this is like a kind of a stark example of technology and language use that can shift how language is used. Yeah. As far as your question about, um, about inclusion, I think inclusion is tricky. Right. You've probably already thought about this a lot. Right. But there is also a lot of thinking about the politics of inclusion, how inclusion is necessary but not sufficient, and how inclusion that just brings people in but without really meaningfully shift power actually ends up being a thing that looks like change, that doesn't change. And it really is like hides the fact that real meaningful change hasn't happened. So I think, uh, when you think about and in both kind of in both kind of like the the technology space more broadly and also in, you know, like, uh, linguistic anthropology work, for example, there's a lot of evidence that really just because you are you bring in different language varieties. Right. Doesn't mean that you value them anymore. So we can see right in there's great work in linguistic anthropology that shows that we might value different language varieties or you might value African-American English, but we don't actually dismantle larger ideas about what this variety is and who speaks it.
Speaker3:
And you know, what we think good or standard language is, which means that the net result has been that some people would get to borrow African-American English without paying the penalty for it. But people who actually speak this variety. Right. Don't read any of these benefits. Right. So the inclusion, just saying my system will now include this language writing. Right. Doesn't actually meaningfully change anything for the speakers that wasn't in my writing. I think one straight one answer that looks very straightforward for these questions of bias or harm or whatever will look like. Let's include brings some more people to the table and let's also maybe just include this other language varieties and. We've ignored these other communities and there are ways to do that, right, that still don't really they don't really amount to meaningful change and that could incur additional harms like this kind of appropriation. So it is, I think, possible to develop processes that are really meaningfully shift power. But we I think we need to be very, very careful about what those look like.
Speaker1:
Yeah. Let's talk about power, actually, because we love talking about power on this show. And you did mention earlier that a lot of this has to do with the distribution of power and what I'm guessing are probably unequal distribution of power. And one of the great things about an LP is that even though on the show we talk a lot about topics that are highly theoretical and maybe these futuristic ideal concepts, but an LP exists today in the systems that we use all the time. You know, like Facebook, Twitter, social media is all full of A.P. And so taking this from like theoretical to practical, what are the distributions of power that exist in the systems today?
Speaker3:
Yeah, OK, so yeah. So let's talk about how MLP systems participate in different social arrangements. So I think there's there's a couple of ways they can do this. It actually I don't know if your question is about the distributions in my how kind of these things are constructed or in in how they affect these distributions in their outcome. But I guess I'll start with the latter and then maybe we can talk about the format. So I think there's a couple of ways the Alpha Systems can and, you know, none of these are it should be like you are new in the sense that, like, these are in many ways look like how language has always participated in the various unjust social arrangements. Right. This just like new kind of shit. Maybe it just looks shiny or now. So one way is what I and actually folks other folks at NASA who really came up with this, we're talking about these, which is educational homes. So these are homes that might arise when a system kind of went systems kind of distribute resources or opportunities. And so this is a lot of what like the fairness in machine learning. And I has thought about credit hiring this kind of thing. And we think I think it's entirely possible that NLB systems can participate in these kinds of things. Right. So language has often acted as an institutional gatekeeper for allocating resources and opportunities. Right. Who gets who gets citizenship, who can immigrate? Who gets access to good medical care that they understand, who gets penalized in the educational system? Who gets admitted to like universities.
Speaker3:
Right. All of these kinds of things. And so and we know that language. So we know the language is used to these kinds of things. Right. For example, a citizenship test for a lot of countries involved, like a language component. And, you know, hiring has always considered often, you know, implicitly or explicitly considered somebody's communication or language skills. Right. So there's a possibility that, you know, it's I think it's likely that as systems maybe get deployed in some of these contexts, that they will also kind of affect different people's access to these kinds of things differentially. The open question is, how do they do that? Right. We don't actually really know where it is that might be deployed in these settings and what the outcomes are. I think another kind of set of harms we can think about are what we've been calling representational harm. So there really and I think these are maybe more not almost more intuitive, because in many ways language is about, um, like, you know, representing the world. Right. And so representational happens, like it can arise between systems, subordinate different social groups, not by differentially allocating opportunities or resources, but representing them in different kinds of ways. Right. So this can happen when people of different groups or people are stereotyped, but also when people are raised right. When it when different topics or different ways of doing language, different language varieties are erased from kind of public view where people are unable to participate in public discourse.
Speaker3:
Right. When certain language parties are stigmatized. Right. When you say, oh, African-American English is offensive, right? Well, it's not. But but it's but it's been viewed throughout U.S. history as a less valid form of language. Right. As wrong or deficient. So and I think it's very straightforward to see how systems can participate in these kinds of things, like the accumulation of a toxicity system, treating mentions of disability as toxic or treating African-American English language as toxic. Right. Does can very easily result in these kinds of things. I also know, too, that it's not a system output. Right. That we can think about, but also just energy systems and practices more generally. So the fact that resources for developing energy systems just don't exist, right. For most of the world's languages, languages and language languages just just means that whatever harms that these technologies can give rise to. There's also tons of people who just can't get the benefits and those benefits are unequally distributed. Um. So, yes, I think I think one thing that I think a lot about right is to think about how do people how do these and obviously things participate in language in these in these social arrangements by perhaps distributing helping to distribute these resources unequally? And then how do they represent people in a harmful way or kind of recirculate or reproduce, kind of very old and a very pernicious, very persistent ideas about different kinds of language and the kinds of speakers.
Speaker2:
So in 2020, we had on Dr. Emily Bender and she's going to give us a 101 of NLP.
Speaker3:
Oh, she's wonderful.
Speaker2:
She's great. But one thing that I've been thinking about since that interview is to what degree are these just like problems of language and politics, of language? And to what degree are these new issues with NLP technology? And I'm wondering from your perspective, like what what's different now? Is there anything different now, now in this technological space than what's happened for thousands of years and the language space?
Speaker3:
That's a great question. Definitely. I think the root of all these things lies outside of an LP, right. The idea is that, you know, judicial systems like don't require an LP to treat like defendants to speak or know witnesses who speak African-American language as less valid. Like these things have existed for a very long time. And in many ways they arise at a process like colonization in this kind of thing. So they're old. And I think it is both helpful to recognize that sometimes frustrating because you do feel like in many ways when you're trying to address for systems. Right. If only it feels in some ways like stopgap measures, like, you know, Band-Aid solutions for a problem that lives outside of NLB. Right. So we cannot really make equitable or just little pieces of dismantle any larger language ideologies. Right. It's not possible. So I think, one, I think things that are maybe I want to say unique or maybe different about technologies is, you know, kind of, I think scale and perhaps an opaqueness right. To the scale which they operate and the difficulty of challenging them, in part because I think it's the case that most of the systems that that we touch on a regular basis that really users interact with are black boxes. Sometimes we're not even aware that they're operating like, you know, how many Alpay systems do you think, you know, affect what you see in your affecting maybe the ads that you see or your search results or, you know, like whether or not your post made it to social media or whatever.
Speaker3:
Right. Like, some houses are not really visible to you. And I think this means that they likely have significant impact. But we cannot see that neither researchers nor the people who actually interact with them. It's really not tangible. You can't and there's no recourse. Right. And you can't really refuse to participate effectively. So I don't think these are in some ways, they're not brand new. Right. Like the education system. Right. Has long kind of like participated in these kinds of things. And it's also very hard. Right, to challenge the education system. Right. So the fact that these things that these things like persist, these language ideologies persist. Right. Because like, you know, they're institutionalized because institutions reproduce them. It has meant that there's always been some degree of opaqueness in scale, that they're very difficult to challenge. But I think technology takes it to perhaps a new level. But that is a great question, and I'll probably be mulling it over this whole afternoon after this.
Speaker1:
Um, so to wrap up soon, for those of us who are either Alpay researchers or just an LP consumers, whether we realize it or not, because this is such a complex issue that is largely hidden from all of us, like you were just saying. Do you have any advice for how we can keep a healthy dose of optimistic skepticism when it comes to NLP systems? What do you do?
Speaker3:
But I think I mean, I think one thing is to be kind of attentive, like just, you know, people are talking about this. Right? So one thing I've seen this actually like this is brand new license, like, you know, kind of recently is people are talking about this and like the these issues specifically related to large language models and of the things have actually made it right into public discourse because, for example, of, you know, like the wonderful work by Tim Newton and Margaret Mitchell and also their treatment by Google. Right. And all these things, these things made it into the news. And I think this represents like kind of an important like a significant moment. Right, that the fact that, like these these things, these language models exist at all with the fact that they actually underpin a lot of products, like across a lot of companies. The like, the kind of so the fact of their existence and the harms they can give rise to the fact they made it into public discourse. For me, I think represents like a really important moment. And I think it is possible for people to put pressure on these companies, particularly folks working for these companies. Right. It is possible to put pressure on them. But I think, like broadly, the the landscape needs to change in a way that makes it possible both for us to dramatically change who is at the table and also kind of what we know about these systems operating in the first place. Right. And I think it is really impossible to make sense of the social implications of these systems to even be appropriately skeptical, skeptical or optimistic or whatever. Right. Without even knowing what the landscape looks like. And I think what we need to do, both as technologies and as consumers. Right. Is to push for, um, like push for much better accounting of this landscape and where these systems like where they touch us. And in order to think about alternatives,
Speaker2:
Insulin for folks who want to follow up on some of these ideas or join this conversation or just reach out to you, how can folks do that?
Speaker3:
Yeah, please email me. Please get in touch with me. Lindop and Microsoft Dotcom. I also have a website and papers are there. But I, as you can, probably still love talking about this and would love to talk about it with anybody who wants to get in touch. So thank you, Susan.
Speaker1:
Thank you so much again for coming on this show. And we will be sure to include all those links and many more in our show notes. But for now, it's been a pleasure.
Speaker3:
Thank you so much.
Speaker1:
We want to thank Dr. Sulin bludge again so much for joining us today for this wonderful conversation. And Dylan, let's start with you. What is your immediate reaction?
Speaker2:
Yeah, I really loved this conversation because although we were talking about bias specifically in natural language processing systems, we were also talking about what it might mean to create a universal language or a universal definition for a phenomenon that might be experienced in such subjective ways. And for me, again, coming from a philosophy background and also in like human computer interaction, it's like this is one of those thorny things that's like how do you take this concept of bias and then actually do something with it? Because I think it's so easy to say, oh, yeah, there's bias over there. You know, let's let's just get rid of that thing. Like, let's not look at our social anything. Let's just say, OK, biases in our technology, let's get rid of it, which we've talked about before on the show, why that isn't necessarily the way to go. And in this conversation, I think Sulin really passed out some of the difficulties in how you actually design for bias and for a diversity of definitions around bias. But what about you? Just what did you come out of this interview thinking about?
Speaker1:
Honestly, pretty similar thoughts to you. I think this is like
Speaker2:
Great minds think alike.
Speaker1:
Some say this is a recurring theme that I think we've seen quite a bit on this show. And just in the field of AI ethics and responsible technology, is this issue with trying to take something that is qualitative and societally based in nature, like something that is so subjectively human and then attempting to quantify it so that we can feed it into models and make predictions with it and do whatever we will with it computationally. And I think that this is just such a perfect example of how something so subjective, like even defining if a word is toxic or not, or stating whether a human is happy or not. Things that are so obviously subjective are being fed into models. And these models are assuming that there is a right answer like Sulin was saying, or these models are assuming that there is a neutral answer or a quote, true answer. And sometimes in life, because humans are so ridiculously complex that that's just not possible. And so now we're dealing with the impacts in the aftermath of attempting to turn something that is inherently unnatural and subjective into something that is seemingly neutral and objective. And there's clearly a lot of harm and unintentional consequences that come with this.
Speaker2:
And let's talk about toxicity, because for me, that was one of the most interesting parts of the conversation, especially in terms of machine learning algorithms, categorizing micro aggressions. So like, how can a LP system really categorize toxicity in the first place? And especially when there are certain words like death or certain elements around like maybe mental illness, and those immediately put this like toxic red flag up for the system when maybe within the context it's like the most healing thing, nontoxic thing possible for the users. And again, this goes back into like human centered design. What does it actually mean to have algorithms that respond to, you know, real context in real time? So there isn't this damage done by saying, OK, here's this, the sweeping statement. But again, then we get to this microaggression part where you're like, OK, but even within like even if you take the machine out of it, like, I would not say that society, quote unquote, society, as if it could be one single thing, has come to terms with what, like a common definition around micro aggressions, or at the very least, there are different camps around what a microaggression consists of, depending on identity, depending on context, et cetera. And so now we're asking this machine algorithm to categorize based off of that. And Sulin, I think, did an awesome job unpacking why that is so difficult. Just what what do you think about toxicity? I think it's really toxic. Yeah, yeah, well,
Speaker1:
Just the same amount of toxicity.
Speaker2:
That's a hot except a
Speaker1:
Hot shot, actually. So I, I completely agree with you. And I think this is kind of like the crux of the issue that we're that we were getting out with Sulan today was that these things are incredibly contextual. And so what I was saying before with like the human nature of subjectivity, there's certain things that humans just disagree on. And that's because they're super subjective. And I think toxicity is definitely one of those things. I mean, I don't think that I'm pretty sure that we've proven that toxicity is one of these weird, subjective, sticky areas. And it's not just that maybe me and you, Dylan, might differ on what we agree a word to be like the level of toxicity that a word might be. It's also like me and myself as a human. I might look at a word and think that it's not toxic. And then I might look at that same word 10 years later and based off of my lived experiences, I might think it's toxic again. And so that was like the heart of the question that I was asking Sulin about toxicity and ground truth labels is that like if we are building systems to guess when something is going to be toxic or to guess when something is a microaggression or hate speech or whatever it is, assuming that there's no human in the loop here.
Speaker1:
This is just like a purely automated decision. There really is no ground truth. There is no way that we can label this word as being actually toxic or this word as being actually not toxic. We can't even make a sliding scale of the level of toxicity that it might feel like, whichever range it might fall into, because it is so inherently subjective. And so it's just getting back at the same issue that we were talking about before where we don't really have a ground truth or a neutral or a quantifiable label that we can give these things that need to be labeled to work in these algorithms. And I come back to this quote that that Sulin said during this interview that I thought was just like spot on. She said a lot of the time there is no neutral decision, but you still have to make one. And so that's where I'm kind of sitting now after this conversation is thinking like, OK, this feels a little bit hopeless to try to quantify all of these things that are so subjective and to deal with all the unintended consequences and the negative impacts. But we have to make a decision somewhere. So what do we do?
Speaker2:
Everything you just said just brings us back to some of the main research questions of the radical podcasts, especially around ethics and morality. So we have the subjective space and then we have this possibly more objective space and then like, what do we what do we do with it? And then what do designers do with it? Because we can't have this, like, just radical subjectivity, because that, as Sulin said, you have to actually do something right. Like we have these systems, they're out there. And so now we actually need to stake our claim on what is going to be embedded in them. But we also can't say that, OK, this is toxic. And this is not because what ends up happening is that the people in positions of privilege and power end up embedding, you know, the status quo concepts of what is toxic, which makes it so that people who are already either marginalized or decentralized in that space or in that system get further decentered. And so the question is right, like what to do. And I think Sullins work brings us to to the brink of how we do those things, which I think is the next step. But I mean, is this different? Right. Is this different than what we deal with on an everyday basis in terms of language? And that's a question I still have, is like, is there something that makes an LP like the fact that it's a machine and not a human? Like it is still language? Right. There's still this processing of language and the way that humans process language, it's still like capsules of metaphor and images and all of this stuff. So I'm still curious about whether these are the same issues at the societal level, like the human societal level and then the NLP level, or is there something unique about this and systems that we need to think differently about than we do about language in our general context?
Speaker1:
I'm not going to speak for everyone because I'm not an expert, but I have written MLP algorithms before. And so I will say from my subjective experience that I definitely stand in the camp that natural language processing algorithms and any algorithm for that matter that uses data, historical data of any kind to make its decisions or to do whatever it's going to do. It has the same problems that humans have because the data is human data. And so I don't think that just because we're automating these decisions or we're plugging them into a machine, that all of a sudden all of our subjectivity can now be taken away. All of our bias can be mitigated and zeroed out. Like, I just think that's not possible. And so I just I really appreciate Sullins work and Emily Bunder and all the other people in this space who are like really focusing in on the issues with an ALP bias and ethics in general, because I think a lot of people do assume that these systems are neutral and they do assume that they have no political or vested interest when in reality that's impossible given the data that's fed into them. Neutrality is a myth. That is our tagline. Radical A.I. neutrality is a myth.
Speaker2:
We should we should put the as the title for this episode. We'll see. We'll see what the data points. You know, I think I think your points are really well taken, though, just. And I think that even if, like, the problem of maybe, say, bias or language and the human social world versus the robotic and l.p world, even if they're the same, then my next question is like, well, then are the solutions to that? The same, which I I don't know I don't know what this I don't even know what solution looks. Maybe solution isn't even the framework that we should look at, because that's what got us in this, like, rabbit hole of where we need to get rid of bias completely. And so maybe Sullins more nuanced view of this is can be a beacon of hope and light for us and for developers and designers out there who are working with these immensely complex systems of language and NLB.
Speaker1:
And hopefully that beacon of light is enough for us to call it for this episode. Maybe we'll have a Part three on an LP Vieth. We'll see if we can. How deep can we dive on this issue? But for more information on today's show, please visit the episode page at Radical Eye Dog.
Speaker2:
And as always, if you enjoyed this episode, we invite you yes. You to subscribe rate and review the show on iTunes or your favorite podcast to catch our new episodes every other week on Wednesdays. Join our conversation on Twitter at radical iPod and as always, a radical.
Sonix is the world’s most advanced automated transcription, translation, and subtitling platform. Fast, accurate, and affordable.
Automatically convert your mp3 files to text (txt file), Microsoft Word (docx file), and SubRip Subtitle (srt file) in minutes.
Sonix has many features that you'd love including world-class support, secure transcription and file storage, automated transcription, share transcripts, and easily transcribe your Zoom meetings. Try Sonix for free today.