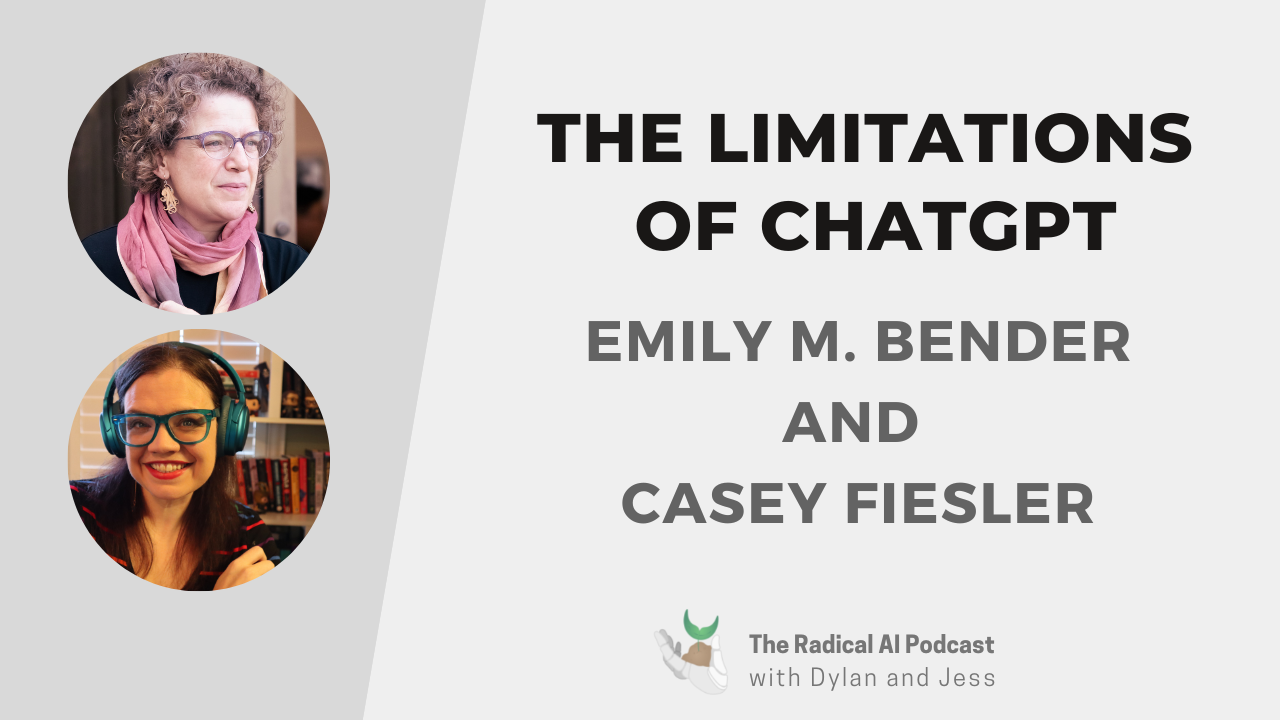
In this episode, we unpack the limitations of ChatGPT.
We interview Dr. Emily M. Bender and Dr. Casey Fiesler about the ethical considerations of ChatGPT, bias and discrimination, and the importance of algorithmic literacy in the face of chatbots.
Emily M. Bender is a Professor of Linguistics and an Adjunct Professor in the School of Computer Science and the Information School at the University of Washington, where she has been on the faculty since 2003. Her research interests include multilingual grammar engineering, computational semantics, and the societal impacts of language technology. Emily was also recently nominated as a Fellow of the American Association for the Advancement of Science (AAAS).
Casey Fiesler is an associate professor in Information Science at University of Colorado Boulder. She researches and teaches in the areas of technology ethics, internet law and policy, and online communities. Also a public scholar, she is a frequent commentator and speaker on topics of technology ethics and policy, and her research has been covered everywhere from The New York Times to Teen Vogue.
Follow Emily on Twitter @emilymbender or emilymbender@dair-community.social on Mastodon
Follow Casey on Twitter @cfiesler or cfiesler@hci.social on Mastodon or @professorcasey on TikTok
If you enjoyed this episode please make sure to subscribe, submit a rating and review, and connect with us on twitter at @radicalaipod.
Relevant Resources Related to This Episode:
Try Out ChatGPT (if you can get through the queue)
Situating Search (Emily’s paper with Chirag Shah) + An OpEd based on that paper
On the Dangers of Stochastic Parrots: Can Language Models Be Too Big? 🦜
ChatGPT Is a Stochastic Parrot (TikTok video by Casey Fiesler)
Vanderbilt University apologizes for using ChatGPT to write mass-shooting email
Microsoft’s Bing is an emotionally manipulative liar, and people love it
Documenting large webtext corpora: A case study on the colossal clean crawled corpus
Exclusive: OpenAI Used Kenyan Workers on Less Than $2 Per Hour to Make ChatGPT Less Toxic
Transcript
chatgpt-limitations.mp3: Audio automatically transcribed by Sonix
chatgpt-limitations.mp3: this mp3 audio file was automatically transcribed by Sonix with the best speech-to-text algorithms. This transcript may contain errors.
Speaker1:
Welcome to Radical AI, a podcast about technology, power, society and what it means to be human in the age of Information. We are your hosts. Dylan and Jess were two PhD students with different backgrounds researching AI and technology ethics.
Speaker2:
And our last episode we covered some of the basics of chat. Gpt three. In this episode, we dig a bit deeper. We interview Dr. Emily M Bender and Dr. Casey Fassler about the limitations of chat. Gpt three We cover ethical considerations, bias and discrimination, and the importance of algorithmic literacy in the face of chat bots.
Speaker1:
Emily M Bender is a professor of linguistics and an adjunct professor in the School of Computer Science and the Information School at the University of Washington, where she's been on the faculty since 2003. Her research interests include multilingual grammar, engineering, computational semantics and the societal impacts of language technology. Emily was also recently nominated as a fellow of the American Association for the Advancement of Science.
Speaker2:
Casey FESSLER is an associate professor in information science at University of Colorado Boulder. She researches and teaches in the areas of technology, ethics, Internet law and policy and online communities. Also a public scholar, she is a frequent commentator and speaker on topics of technology, ethics and policy. And her research has been covered everywhere from The New York Times to Teen Vogue.
Speaker1:
And on a more personal note, we just wanted to shout out that we are so excited to share this interview with Emily and Casey with all of you, because we have known Emily and Casey for quite a while now. Emily, we first met when she was on the show, I think it was almost three years ago now. She was one of our our first community members, and she has been an avid supporter of the podcast and of this project basically from its beginning. And Casey is my one of my PhD advisors at the University of Colorado. So I've known her for quite a few years now too. And both of them come from totally different angles and areas of expertise. When it comes to chat bots and chat specifically, we have Casey, who has the law and policy background, and then we have Emily, who has the natural language processing background. And so we were just super excited to bring them together in conversation for this interview and we're really excited to share the outcome with all of you. We are on the line today with Casey Kessler and Emily Bender. Casey and Emily, welcome to the show and welcome back to the show.
Speaker3:
Super, super excited to be joining you for this.
Speaker4:
Thank you. This is Casey, and I'm very excited to be on the podcast for the first time.
Speaker1:
Yay! And today we are talking about a topic that has been receiving a lot of news attention as of late. Chat. We're going to begin with the basics here. So, Emily, our first question is for you. Could you briefly summarize how GPT works and what makes this technology so novel in the first place?
Speaker3:
Yeah. So. So what is chat GPT? Chat GPT at its core is what we call a large language model, so that the internals of it, the sort of guts are an enormous number of what's called parameters in this very large neural network that have been set through a training procedure where the system sees just piles and piles and piles of text and computer code. I think in the case of chat GPT. The text isn't just English and it's training task is predict what word comes next, predict what word comes next, and then compare that prediction to what was actually in the text. And the training algorithm then adjusts those parameters based on how right or wrong it was. And it keeps doing that over and over and over again, over just an absolutely enormous amount of text. That's the first pass. Once you've got that large language model, what they've done, I have to say it's not entirely known outside of open AI. They have not been open about this. But the blog post basically said we've done some further training and some of it seem to have to do with dialogue to make it be more like a dialogue system, because most of the text you can find on the web is not dialogue. And so there's some training about dialogue, and then there's this phase called reinforcement learning from human feedback, where human raters were given output of the system and asked to rate it as as good or bad or helpful or unhelpful or whatever their rating system was. And so now the system is not only trained to predict what the next word should be, but what the next word should be so that it would be well rated by humans, such as those who are doing the ratings.
Speaker3:
There's another layer in there which has to do with trying to suppress toxic or hateful or otherwise problematic output. And we learned from Billy Perrigo reporting in Time magazine that that was outsourced to Kenya to poorly paid workers and who had to do this very traumatic work of looking at these terrible outputs. So that that's sort of how it's built. So you also asked what's novel about this tech? And I would say not much. What's novel is the way it's been promoted. So we already had, well, internal to Google. There was already LAMDA, for example, that became big news when Blake Lemoine decided that Lambda was sentient and needed help, which is a very similar system as far as is known. There was already GPT three from Openai, which is kind of a predecessor. It didn't have this dialogue overlay, but it did have the ability to generate coherent seeming text that would be somewhat pleasing to humans. But what's new here with GPT is that open? And I set it up with this interface that allowed people all over the world to play with it. And so you went from a world where it was basically people in tech who were playing with this stuff to people from all walks of life, playing with it and being exposed to it for the first time. And I think that's the real novelty.
Speaker2:
And that novelty we've seen a lot of hot takes on in the media and beyond. And as our, I guess, social media star on this episode, Casey, the Tik Tok star, I know that you're well embedded into a lot of these communities, a lot of these conversations. And I'm mostly wondering, what are you hearing and what's your reaction to it?
Speaker4:
I think that a lot of people are very impressed by what can be by what can be accomplished with with chat or what it what it can do. And I think it's reasonable that they're impressed. It's it's impressive. But in in a way that like I feel like the shine is going to wear off kind of quickly because, you know, the first time that you ask chat to write fanfiction of Sherlock Holmes on the Star Trek Enterprise, you are incredibly impressed that it can do that at all. Right. But then as you as you keep going, you're like, well, it can do that, but it's not very good at it. And my friend, the fan fiction writer, could have written something much better. So I do think that there is some extent to which this sort of like, Oh, this feels like magic is going to wear away a little bit. But I do think that one of the challenges here is that everything that Emily just explained, which I think makes a lot of a lot of sense to a lot of our listeners, is still kind of challenging to wrap your head around, like what this actually means. Like, you know, people are saying like, oh, it's just fancy autocomplete and like kind of but also when you actually then see it do something, you're like, Well, that seems much more magical than than autocomplete.
Speaker4:
And I was actually reminded, like when everyone started talking about chat. Gpt, which, you know, is a chat bot, I was thinking back to Eliza so the, the, the chat bot that that Joseph Weizenbaum created many, many, many years ago and I think wrote an article about it in the 1960s and. It was you know, it was procedural. It was mimicking a psychoanalyst. And so the way that it worked was just a set of rules. Like if you say, you know, my mother makes me sad. Well, tell me more about your mother. And that makes a lot of sense, right? But even then, he pointed out that, like this was sufficient to really dazzle people at the time. But as soon as you explained how it worked, like as soon as you could use language understandable enough to explain, like the inner workings of it, the magic crumbled away. And so I think that's part of the challenge here, is that it actually is kind of difficult to explain how this works at a level that the magic crumbles away for people.
Speaker3:
Yeah, I think that that's spot on, Casey. Part of the reason for it, as far as I can tell, is the unfathomable size of these training data sets. So we just don't have lived experience with working with that much text in working memory or frankly at all. And so it's really hard to have an intuitive sense of just what you can get from distribution patterns over enormous collections of text. And so people can say, Yeah, yeah, yeah, I know it's fancy autocomplete or I know it's a stochastic parrot, but still, how did it do that? And especially it's very good at anything to do with mimicking the form of language. So, you know, write instructions for operating a VCR in the language of a Shakespearean sonnet. It will do that in a very impressive way. And I think that some of the magic there is that that's a difficult trick for humans. There are some humans who are very good at it, but it's also something that we find impressive because of what kinds of mastery it would require a human to have. But that doesn't mean that Chadwick is doing it in the same way. Ted has no understanding, no communicative intent, no sense of what the sort of social meaning or value of VCR instructions and Shakespearean sonnets are Just it's got a lot of information about linguistic form.
Speaker1:
Emily You used the expression stochastic parrot, and this is an expression that has also received a lot of news attention for various reasons in, in our broader community. Would you mind briefly describing what you mean by, by that expression and how that relates to chat?
Speaker3:
Gpt Yeah, and so this, this expression comes from the title of a paper that I co-authored with Timnit Gebru and Meg Mitchell and Angelina McMillen Major and several others who had to take their names off because their employer was displeased. And it was a paper written in late 2020 looking at the risks associated with building ever larger language models. And we are now in the very unpleasant position of seeing a lot of the things we've warned about happening. Just no fun at all. The phrase stochastic parrots was meant to cut through some of the hype with these things because it is impressive because, as Casey says, it seems magical because they seem to be speaking our language. It's really hard not to imagine a mind behind it. And so we use this phrase to describe stochastic means happening randomly, but according to a probability distribution and parrot by parrot we don't mean the actual animal because actual parrots are animals that probably have some kind of internal life and intelligence and whatnot. But the sort of expression of just parroting back information. So parrots are used metaphorically to refer to something that something or sometimes someone who is repeating things back without understanding or intent and just, you know, word to the wise. If you're going to write a research paper that gets a lot of media attention because a company decides to fire people over it and make sure to have a catch phrase in the title, it's really effective.
Speaker2:
Well, And, Emily, you've used the term cutting through the hype. Casey, you were talking about how impressive this technology has come across and also that there's a need to break through the the magic. If I if I got that right or that there's an understanding that maybe this is untouchable or something that we don't entirely know what to do with. And I'm wondering what is the danger of hype here? So as we move into the social world, what are the downstream impacts of us just giving our full hype to new technologies such as chat, GPT or maybe chat GPT in particular?
Speaker4:
Well, I do think that beyond just this example in general, it is very helpful for people to have an understanding of how types of technology work so that they can understand their limitations and also when they are working on them. Right. So, you know, one of the one of the issues with the Facebook emotional contagion study in 2014 is that a whole lot of people had no idea that there was an algorithm at all. And so hearing like, oh, my gosh, Facebook is is manipulating me was a was a big deal, you know, regardless of the experiment at the at the bottom of it, it was just that people didn't understand that algorithms were a thing on social media. Now they do. And now I think more people have an understanding that, oh, the content that I'm seeing on social media might be personalized to me or it might be based on these different kinds of signals or that kind of thing. And that's important. And similarly, I think that knowing that chat GPT is. More autocomplete than search engine is really important for people to understand the information that they're getting, getting from it. And so I think hyping it too much like, oh my gosh, this is so amazing. It's going to it's going to change everything sometimes sort of clouds those limitations. And in particular, I think how much bad information it provides right now is a really, really important part of that, because if you think that everything that comes out of it is truth, because it's a search engine and it must be getting this credible information from somewhere, then people might believe it blindly, which I think is one of the risks right now. Just one of the risk right now.
Speaker3:
I've actually been tracking passively cases where that's happening. So I've now seen on social media reports from archivists, archivists and medical librarians and open source code writers. And then also a friend of mine who's a math professor who participates in math, Stack Exchange, and all of these separate people are saying they've dealt with queries from people who want them to find information that told them about. And so is this interesting thing where you have the initial chat user who is being misinformed and then you have this extra work that's being put on on just bystanders. Right. So that that is, I think, a really stark illustration of not just that it is bad for search, but that people are using it anyway and don't understand that it's bad for search. So we need people to cut through the hype. And it's especially key, I think, with these language interfaces, because it puts on a good show of understanding what we're asking for. It gives us things that look like answers and that sound authoritative. And so there's this big risk of misinformation. And then the misinformation doesn't just stop with the person who asks the question, right? So it becomes a problem for these other experts that they're talking to, or it can get posted places. So Stack Exchange in particular, banned chat real fast because they didn't want that information basically polluting their information in space, which is the whole value of stack exchange. And then another really important kind of misinformation is the bias and discrimination and reflections of system of oppression that come out through these systems. And I think that that is sometimes seen as a separate issue, but better understood actually as fundamentally a kind of misinformation and one that is very, very damaging.
Speaker4:
And I would I would add to this, actually. I think that one of the issues with with people believing in information or like thinking that GPT is a search engine, is that in a search engine you have the ability to evaluate the source of the information, right? So you Google a question and actually an example of of bad information that I got from chat as I was asking it to compare car models for me. And it told me that a Volkswagen Taos did not have all wheel drive. So I Google does a Volkswagen Taos have all wheel drive, and then I'll get an answer and I can be like, Oh, this is from the official Volkswagen website. It must be true. But when Chalkbeat told me that it does not have all wheel drive, I did not know what the source of that information is, because there is no source, because it's not a search engine. And so I think that a huge challenge here is actually exactly what Emily was just describing, which is that you can't evaluate the source of the information, which means that every piece of information you get from it, you have to evaluate independently. You have to go find a source of that information.
Speaker4:
And actually, I was just talking to someone recently who said that they had gotten some information from Chalkbeat that they suspected that if it was true, might have come from some kind of internal documents that were not public from companies. And so she was contacting companies to ask them if this thing was true, because there's there would be no other way to evaluate this information. And so I think that is a real challenge when we know that some of the information is wrong. For example, I had Chalkbeat write a PhD statement of purpose for a biology PhD program at my university and gave it a list of biology professors, but plus myself to include in the statement of purpose for why you want to work with them. And. Whereas, it had done this very well for information science, it then was like, I am so excited about Professor Kay's research on plant genetics because again, it's very it sounds good, you know, it's the right words to come next. And you can imagine in the innards of what words come next here. What's more likely that, you know, you're lying about the name of a professor or like, Oh no, they probably are really a biologist. Let's just keep.
Speaker2:
Going.
Speaker3:
Yeah, absolutely. I think you're touching their case on the really important issues of information literacy and the way that that has to be a lifelong project. So there's a paper that I wrote with Cheryl Shaw for a conference called Cheer, which stands for Computer Human Interaction and Information Retrieval. And that came out in in early 2022, where we were talking very much about this proposal to use chat bots as a replacement for search because Google was already talking about it. It came up in Sundar Pichai CES 2021 Google I o presentation. And then there was a paper by Metzler et al proposing using large language models in place of search engines. I think we we weren't talking about in terms of chat bots so much as large language models. And my reaction to that was linguistically, this is a terrible idea because I understand that these things don't understand, but they're going to make it sound plausible. But I would like to talk to someone who understands things about information, science and information behavior. And so I teamed up with my colleague Schrag, and we looked into how how the chat interface, even if it's giving you accurate information, cuts off your ability to do the sort of sense making and exploration and location of the information sources in their underlying system.
Speaker3:
So to pick up on your Volkswagen example there, maybe Google would take you to or another search engine, DuckDuckGo would take you to some like car reviewer site that gave you some information and you think, well, I don't know that I trust them. But the next link down is Volkswagen's own website. And hey, it matches in this case. And then you've learned something about that car reviewer, a little bit of evidence that maybe they know what they're talking about and so on. And so over time, you get a sense of what are reliable sources, what they look like, how they relate themselves to other kinds of information. And if we start using a chat interface, even an accurate one, which I don't think these things could ever actually evolve into, we're not on a pathway to that. But even if we were, we're going to be doing worse in terms of people's information literacy.
Speaker4:
I'll just add one more thing to this, actually, because I think this is really interesting. So there are some things that there are some tasks you might use chat for where the information, if it's wrong, does it really matter that much? Right? So like my fanfiction example, like if there is some piece of inaccurate information about the canon of Star Trek and the fan fiction that is written for me, like that's probably fine. Or, you know, task that you're using it to like help you write an email or something like that. And so I think there's there's also this really important piece in thinking about like what are appropriate ways to use this versus not. And this is why I get really concerned about students wanting to use chatbots to like write essays for them and that sort of thing. I think that helping you write an essay is one thing. If you're thinking about like communication styles and that sort of thing. But I, for one, would not trust any information that came out of chat. Gpt without verifying it from some other source and then I get students telling me, Well, that's what I would do. And I'm like, Excellent. It sounds like you know how to do an assignment, like, Let's do it.
Speaker1:
Let's take this thought to the next step, because this is actually where I was planning on, on going with my question anyway in terms of like when we can trust chat. Gpt when is it more important that we're able to trust chat? Gpt If we're not able to trust chat? Gpt should we even use it for certain kinds of contexts? And so I'm wondering maybe just at a high level, what are people using chat GPT for in the first place right now? What, what are the current normal uses? What are the potential abuses of chat GPT? How are people using this?
Speaker3:
So this is Emily. The things that I'm seeing that are maybe less problematic, although there's there's no use case that I want to say. Yes, this is good. Go for it. Like even the ones that seem relatively safe, I sort of feel like, okay, it's overkill. And like, is that really a sort of resource efficient way to to do this? And every time you use it, you're also giving data to Openai. And there's there's lots of reasons not to, but sort of the less frightening uses are things like getting boilerplate code doing rephrasing from like bullet points to an email and maybe getting unstuck. If you have writer's block and you're starting a writing project or a writing assignment, I can give you my reasons that I dislike all of those use cases too, but I think those are sort of the less problematic ones. And then you've got people using it for search, as we were already talking about. You've got people using it to answer other people's questions. So search it. One remove. This is the StackOverflow use case and potentially you could have people using it to create misinformation, to create harassing articles about people. And like, it's pretty easy to think of of lots of really nefarious use cases. I think about Yuki, what have you seen?
Speaker4:
Yeah, I think that things like writing boilerplate emails and like boilerplate kind of copyrighting is a fairly innocuous use case. So I will say that an example that I saw yesterday where headlines were like Vanderbilt apologizes for using Chat GPT to write an email about a mass shooting. And in this case it was, you know, they had at the bottom of the email like this was paraphrased from Chat GPT or something like that, you know, and actually along those lines in theory it's good that they said that that's how they wrote it, I guess because I think that a lot of the poor use cases of chat have to do with deception. Right. So, you know, maybe the issue isn't using GPT three to help you with your communication for your homework. It's about passing it off as your own, which in theory is also against openai's terms of use like it does. Say do not pass off. I created output as human created and I think that's if you want to compare using chat GPT to plagiarism, which I think is something slightly different. But that's the issue, right? It's the deception. If a if a teacher told you, you know, write this all by yourself, don't use any tools to help you and then you use GPT for it, that's deception. It's not about necessarily using it as a tool, it's about using it as a tool when you're not supposed to. Or, you know, something else that I've heard a lot about in the past couple of weeks are self published books all over Kindle being written by Chalkbeat. And one of the articles that I read about it said, You know, there's probably a lot more because this is just people that we know are using Chalkbeat to write it. And so I think that that is going to be a very interesting normative conflict in the coming days is how much obligation people have to disclose that something was created from A.I..
Speaker3:
That's reminding me of the whole thing going on. I think it's called Class World, the science fiction magazine that basically had to close its submission portal because they were getting overwhelmed by synthetic text. And my reaction to that was, what are people thinking? Like, nobody wants to read this chat. Gpt generated drivel. Like why? And like, did you want to have your name on a publication? Is that the thing? Well, no, apparently what it's about is that it's actually one of the places where you as a as a speculative fiction writer can get paid to publish. So people are trying to just like get through and get money out of this. And some folks on Twitter were speculating that some YouTuber suggested that this would be a good way to use chat GPT to make a quick buck and like down goes this wonderful community resource where writers can get their stuff published.
Speaker4:
As someone who has submitted to Clerks World before and been promptly rejected, I'm very upset by this. I would I think this is actually coming up in the fanfiction community as well. There are a lot of. People who are very unhappy about seeing. I authored fan fiction on Archive of Our Own, for example, and I also saw someone say like, Oh my gosh, you know, I'm never going to have to go to Archive of our Own again because I can just ask GPT to write me whatever fan fiction I want to read. And I'm like, Yeah, if you want to read bad fan fiction, like I, I do think again that that sort of shine is going to wear off, but I absolutely cannot imagine someone thinking that one of these stories could possibly be accepted by Clark's world, which has like a 1% acceptance rate or something, is forcing an NSFW proposal.
Speaker2:
So I have a reaction to this conversation, Right. Like as a poet, etc.. Like I'm like, Oh my God, deception. That's bad. Also bad for me individually, but also genuinely like the process of art. And I think my question is, what do you think is behind that reaction like that, that guttural feeling of like, Oh my God, this is wrong.
Speaker3:
So my impression not as a poet myself, but actually as the child of a poet, is that art is really about sharing human experience. And we like consuming art because it allows us to understand the experience of the artist or to have an experience that is prompted by the experience of the artist and the process of creating art, and especially poetry. From what I understand and this is my mom, Sheila Bender is a poet and she does a lot on sort of writing from personal experience is that that is extremely valuable to us as a way to understand our world and process emotions. And so similarly to what Casey was saying about the chat, GPT generated email in a moment where what you really needed was authentic caring from the authors. Synthetic art is going to be hollow and this isn't unique to GPT because we saw this with visual art already with stable diffusion and mid journey and things like that. And I think the open question is, is there a way to use these tools as an artist so that you are creating art with the tool that expresses your own human experience that someone else can then connect to, which is different to someone saying, Write me a poem about and then just see I have a poem which is just not what poetry is for.
Speaker4:
I actually think that this is sort of at the heart of a lot of the conversations about generative AI that have been happening in artists communities and actually more related to visual art generation than chatbot. So just a couple of days ago, for example, the Copyright Office in the US partially rescinded a copyright registration that they had given to a comic book that had art generated by my journey in it. Because we have a human authorship requirement for a copyright in this country. And I, I mean, the the law around this is going to. It's going to be evolving for a while, and it's fascinating. But we're going to end up with a whole lot of like both, I think, ethical and normative discussions and like legal sort of edge cases to try to work out what constitutes authorship. Like, how much do you have to edit something or how much do you have to be working with the prompt? You know, if you just type girl in a red dress into Dali, I think most people would agree that that was not human authorship. But if you spend 100 prompts tweaking something in mid journey that where the prompts are like paragraphs long and you keep changing them to get exactly what you need, maybe at that point it is enough sort of human creativity that we would feel differently about that, both legally and ethically. But I think that a major component here is like, again, just not being deceptive about it, because then people can make a decision about what kind of art they want to consume and how they want to feel about it. There was also a big controversy in August in Colorado, and someone won a digital art prize at a show for art that had been generated via imagery. And so, you know, people ask, oh, you know, is this really different than like Photoshop? And yes, it is. But the way that you can use it is very different. And right now, I guarantee you that the judges in that art contest absolutely did not understand what it meant to generate a piece of art with my journey.
Speaker3:
I'm reminded in this discussion of Sasha Costanza, Chuck's notion, or rather I have it from them, it might be more broad of contentful tech. And so if you are being subjected to synthetic text without being told that that's what you're going to read, then that's a violation of consent. I think that's a lens that we can use to think about deception. And when we talk about consent in tech, there's the other end of it, too, right? What went into it were did people consent to having their creative expression, either visual or linguistic used as fodder for this thing? And I think at the moment we're seeing a lot more uproar about that on the visual art side. And that might be because any given piece of visual art takes a lot more effort than most pieces of writing do. I mean, poems take a lot of effort. You know, it's not all the same, but but you can write an essay, I think, with far less effort than it would take. Even a very skilled artist to paint a painting. So I think that that we need to think about and view the people at all levels of this and at the very basis of it. In most cases, there are people who have generated what becomes the input for the machine learning system. The reason I say in most cases is that we now have this problem of, especially with text, the output of the machines becoming the input for the next iteration of training data. And that's unpleasant and possibly quite problematic as it sort of pushes these spirals of discrimination and things like that. What comes out of the system on one level is already amplifying the discrimination in the training data. And if that becomes a training data, again, I see problems down that road.
Speaker4:
Yeah, that is the other side of the copyright discussion is training data. And I think it's very reasonable that a lot of people are upset about this. I do. I think it's a slightly separate ethical and legal issue. Like I think there is a pretty good chance that using artwork in training data could be considered fair use depending on some of the specifics. But I think ethically it feels a little different. I will say that in the context of text as well. A lot of actually back to the fan fiction example, a lot of fan fiction writers were very upset to discover that their work was clearly included in the training data for Pseudo Right, which is a GPT three based creative writing tool because of like some extremely specific kind of characters and circumstances that were coming out of it. But they were actually partially upset from a privacy standpoint in the sense that like there are very strong privacy norms in this community and like, who is reading your fan fiction? Like you sort of understand that it's like within the community and not like random people outside. And so I think there are actually some interesting privacy issues with training data to.
Speaker1:
Speaking of training data, whenever I think of training data and chat bots. My brain goes to Tabart from back in 2016 when like within 24 hours this bot that was connected to data from the internet was just like super racist and sexist and misogynistic and was just a reflection of like the worst parts of the internet and chat. Gpt is trained from GPT or it's using GPT three, which is trained on just a ton of internet data. And so obviously the internet is full of some not so great things and reflects a lot of biases in the real world and in the digital world. So Emily, I would love to hear you speak to maybe some of the more pressing concerns surrounding the bias and discrimination that comes from the training data.
Speaker3:
Yeah, absolutely. So the first thing is so Talbot and it's fun to think that like Galactica lasted three Talbot's That was, you know, Metas language model trained on scientific texts that was promoted as this way to access the world scientific knowledge. But of course it's a language model, so it's just making stuff up. And that got taken down after three days for different reasons. In the case of today, what happened was it was set up to specifically learn from the people who interacted with it, and so it got deliberately co-opted and corrupted by far right extremists who who worked in concert to turn it into something that was racist and sexist and all the things. So I don't know why in 2016 this was a surprise to anybody. But like the sort of that level of, well, we'll just take in whatever you give us is, you know, we're past that by now, at least I'd say the first and very fundamental problem with chat GPT is that we don't know what its training data is. And in 2017 there was a bunch of projects that started that were about how do you go about documenting training data and models? And so for natural language in particular, I was involved in something called data statements. At the same time, Timnit, Gebru and others were working on data sheets which were inspired by like the component specifications that you get with electric components if you're if you're building something physical. And related to that, Mike Mitchell and others did model cards.
Speaker3:
There's a whole bunch of these that are out there. And what's shared across them is this idea that if you don't know what's in the training data, then you are not positioned to decide if you can safely deploy the thing. And so that's sort of a big red flag to me that we don't know what's in this training data. Another thing to know about it is that these data sets are actually too big to effectively filter. And so you see this with open AI, you see it with the reports of the what's coming out of Bing. So the new Bing, I've been calling it Bing GPT, the the chat functionality in Bing where there are guardrails that are clearly after the fact guardrails because you just the training sets are too big to go pull this stuff out underlying Li and that's the guardrails are always going to be too flimsy if they're done after the fact like that. And I'm sort of imagining it as, let's say you live in a city that has a really primitive sewer system where you basically have open sewer lines running through the city and, you know, you build some walls next to them because you don't want the sewage overflowing into the non sewer parts of the city. But then a storm comes and there's too much water. And like those walls necessarily fall. And it's kind of like, well, why do we want the sewage running through the city in the first place? Like, isn't there a better design decision we can make underlying Li here? And another sort of part of this is that you have both overt toxic hate speech and sort of more subtle systems of oppression and.
Speaker3:
In trying to filter out those more overtly toxic sources, you can increase the sort of skew of the more subtle systems of oppression. And this was documented by Willie Agnew and others where they found that the strategies that were being used in, I think, the colossal cleaned common crawl. So that's a big sample of the Internet where it's, you know, there's effort being made to make sure that you don't have Web pages that are just there as search engine optimization spam, and you don't have lots and lots of duplicates and you don't have computer generated text and you don't have porn. Right. And the way this is done is basically keyword filtering. And those keywords will include things that are actually identity terms, for example, for LGBTQ people. And when you do the filtering that way, you lose not only sort of maybe some of the porn sites that you're hoping to keep out of your training data that are focused on specific kinds of pornography, but also articles and discussion boards where people are speaking in positive terms about their own identities. That skews what is said about LGBTQ people in the training data and skews the kind of statements that can then come out of the model.
Speaker2:
When we're talking about the training data and how the data that these models, this model, these models are trained on comes to be. One thing I'm trying to still wrap my head around is the question of labor and how this came into being in maybe the more material side. And I'm wondering if either of you could speak to the labor of who has been doing this human in the loop work to construct this model in the first place? Or do we know?
Speaker4:
Well, there was a story in time about the underpaid workers in Kenya, and that was actually related to what Emily was just talking about, which is that a lot of the safeguards for things like toxicity are based on knowing what's what's in this data that's bad, that then you then shouldn't be in the output. And so very similar to like social media content moderation, we had these folks labor labeling data that was in chat as, Oh, this is really bad, which and I know that at some point you've had Mary Gray on this podcast, so probably have talked about this before, but that kind of work can be incredibly. Distressing, harmful in particular. But it's another example of that kind of ghost work of like this is one of the reasons that chatbot seems smarter than it is, or at some point they're being human laborers in the loop. But beyond that article, I'm not sure if we know a lot more about how Openai has been doing this.
Speaker3:
Yeah, there's really minimal information. So again, open I not being open. And when you're thinking about do I want to use this thing for generating fan. Like why when you go read fan fiction written by good fan fiction writers but for these purposes or to help me start with my homework or to help me with this corporate email, it's worth keeping in mind those people and thinking about how do I feel about the ethics of how this was produced and how do I feel about using it, even if it's free to me right now to use? What am I benefiting from as I use this tool?
Speaker2:
For folks who are listening to the show, whether they're a consumer or whether they're a maybe developer or designer who is trying to bring chatbots into their app. I'm wondering if you had if there was one thing that you each wanted each of those groups to know what it would be. So the consumer, what's one thing you would want a consumer of chat or I guess a user of chat app to know? And then one thing that you would want a I guess, developer or designer who's looking to use chat and one of their products to know.
Speaker5:
Well.
Speaker4:
Let me start with the second one. So what are some things that developers who are thinking of integrating this should know? Well, there already is an excellent case to learn from, which is Bing. And I think we all saw that what happened there was a case of moving a little too fast and breaking something. I think that it's important that I mean, I think that this is true for the development of any kind of technology. But I think that it's important to be thinking very strongly about adversarial use cases as well as things that might unintentionally go wrong. But like the fact that the fact that a lot of this stuff happened, like even just the cases of very simple misinformation that came from both Bard and being during their demos, like the fact that that could happen during a demo that like adequate testing had not taken place to avoid That just absolutely blows my mind. And I think that that suggests that there is there has not been a lot of testing taking place yet. And so I think that it's just incredibly important that technology designers who are who are even considering, you know, using this kind of technology has a very strong understanding of the limitations and what could go wrong and what could go wrong when awful people are awful, which happens every time, and what kinds of safeguards can be put in place for it. I mean, I think the genie is out of the bottle at this point. We're not we're not we're not going to get to a no one's going to use this kind of a kind of place. So I think we're going to have to be thinking about harm mitigation. And unfortunately, that is very often reactive instead of proactive. And so I would really like to see some learning from mistakes that are already happening.
Speaker3:
So I think I'll start by speaking to the consumer perspective. And the main thing that I would want a consumer of this technology to know is that the only thing that you can say that a language model knows is information about the distribution of words. It doesn't have information about the world. It doesn't have information about ideas. It doesn't have feelings. All right. And so keeping that in mind when we're interacting with it is really, really important. And it certainly is not an artificial intelligence. That's a ridiculous way to talk about these things that just muddies the waters to the people who might be developing with this tech. I think what I would like them to keep in mind is that the apparent fluency of the language models puts us in a place where our reach exceeds our grasp. And so it seems like we can build a system that can provide answers to medical questions. It seems like we can build a system that can produce legal documents. It seems like we can build a system that is an effective way to create new recipes or whatever it is, because in all that training data, there are the linguistic forms to talk about all these topics. And so these systems that would in reality take a lot of very specialized effort to build all of a sudden seem cheap or free and they're not. And I think that ties exactly to what Casey was saying about the importance of evaluation, that just because it looks like it's doing those things, you shouldn't put it out into the world without doing really rigorous testing. And that really rigorous testing is going to find that it's not going to work. So I want to say one more thing to the developers. I can't do just one. Are you kidding? Which is that it is really, really important to set up your processes so that, no, we're not going to do this anymore. Let's stop is actually a viable answer at the point that you're doing evaluation.
Speaker4:
Um, I, you know, I think I'll I'll add one more thing, which is maybe like a point for consumers. You know, I'm we have seen a lot of, I think, fear about job loss and obsolescence. So I do think it's important to remember a lot of the limitations that Emily have been talking about. So, again, I don't I don't think we're replacing creative writers any time soon. The other thing is that I think there was a lot of concern when, you know, for example, Chalkbeat passed the bar exam and a medical licensing exam. I mean, I got to tell you, I'm 100% not surprised that a chat bot could pass the bar exam like I took it. It's like talk about regurgitating information in terms of where words go. But I think there's been some concerns about this, like, oh, we're going to get like robot doctors, right? And that that is not even in the remote near future. The best case scenario out of this right now, I think, is, you know, that we get tools that can help people. And, you know, in the medical context, A.I. has been greatly helpful in terms of like certain types of diagnosis and imaging and that sort of thing. And as long as you understand the limitations and the kinds of mistakes that it has, that it has, you know, I think that doctors having access to tools is is great. But we're going to need we're going to need to have a very good understanding of the kinds of mistakes that this particular tool is, is is making before, you know, doctors have have AI in their pocket. But I'm not worried about the vast majority of of jobs right now.
Speaker1:
Well, as always, we could discuss these topics for so much longer and I'm sure that there will be many more topics surrounding chat to discuss in the future. But for right now, Casey, Emily, thank you both so much for helping us gain some clarity on the limitations of this technology and to set the record straight about chat. Gpt So thank you so much both for being here, for coming on the show.
Speaker3:
Thank you for having us and thank you for all the work you do on this show. I think it really is a wonderful place to go to learn more about what gets called AI and how it interacts with the world.
Speaker4:
Yes, thank you very much for having this. And it was great chatting with you, Emily.
Speaker2:
We want to again thank Emily and Casey for joining us today. It's really cool for us to be able to talk to people who have both been supporters of the podcast for a long time, but also supporters of us individually for a long time. And it was great to be able to hear them discuss this really interesting and sometimes thorny issue that I think, Jesse, you and I and maybe the rest of the world are so wrapping our heads around. And as always, we'll do a quick debrief. Just what are you thinking after that interview?
Speaker1:
So many things. I feel like we covered like 20 different ethics topics in this interview. So I was like, where do I even begin? But what I think I took the most notes on during this interview was when we were talking about the topic of chat GPT versus like search engines. So how like chat GPT differs from Google Search, for example. And I think coincidentally it might have been from either a tweet or a retweet from Emily or Casey on Twitter that I originally got this idea from. But I, I heard this concept a few weeks ago and I thought it was really useful regarding this like tension between search and chat bots. And basically the tweet said that you can think of chat GPT as sort of like a blurry JPEG image of the Internet or like a blurry JPEG image of like search results. If you were to Google search a question as opposed to like the actual clear, crisp, concise, first primary source responses that you'd see on like a Google search, for example. So like people assume that the response to a question that they get that they prompt GPT with is like this truthful answer. But in reality it's actually just sort of like this aggregate summation of all the potential truthful and untruthful answers that there could be because it's just scraping this data from the web. And as Emily described a bunch of times in this podcast interview, it's just trying to to statistically determine a probability of what words are going to come next. So it doesn't actually know what it is saying, Like it's not actually trying to. The purpose of the technology is not to answer your question. The purpose of the technology is to try to create an answer to a question that seems like it is the correct answer to your question, and it sort of resembles this like, blurry image. So I just wanted to call that out that I was thinking about that as they were talking about that topic. And that was the first thing that came to my mind. So what about you?
Speaker2:
Dylan Yeah, I was really taken by the conversation around deception, and I'm just again, as as an artist, but also as an academic and someone studying technology and is interested in how people make meaning around technology, the anthropomorphic that God, I can't say that word. Yes, I can't say the word anthropomorphic. So I was so close. It's a mouthful. The putting of human characteristics onto technologies. It's just as much of a mouthful. It really is. But I can I can say all the words so that's I can enunciate it that that process that I think we're seeing through this this chat program of like saying, okay, you know, I'm chatting with something and I have some level of trust with this thing because I'm not seeing it as just this probabilistic system of just taking best guesses and then putting words in sequence. But that we're thinking, oh, you know, this. This bot has created something akin to maybe an artist that we would be in communication with or akin to someone that that we're speaking to, you know, a therapist or what have you.
Speaker2:
And so this idea that we have given so much importance, so much hype to this new technology, I think is in part to when you know that we're chatting with this thing and that we're able to put all of these human characteristics on this very not human thing. And yet there are these whole like systems of humanness behind it, like how we're doing the data scraping, how, you know, Casey was mentioning the fan fiction writers have had their data scraped in order for this chat. Gpt to appear as if it is also a fan fiction writer. And so that concept of deception, I'm still, as I'm talking out loud even and still playing around with my mind of like, who's doing the deceiving to what end. Is there use value in that deception? And if there is use value, then to whom is there use value? And you know, for us, for you and I as researchers, you know, how do we even use this thing, especially if we agree that parts of it are based in basic psychological deception?
Speaker1:
Hmm. You know, as we were talking about this, the concept of anthropomorphizing or whatever, this this chat bot.
Speaker2:
I just show off. Sorry, I just had to say that word.
Speaker1:
I had to make sure I got it in there one more time. It did remind me, though, of an older episode that we have with I believe it was Miriam Sweeney on. It was like chat bots, but also just like voice assistants generally and how we anthropomorphize those. I got it in there like a fourth time. That's great.
Speaker2:
Yeah, that's right. You're really just rubbing in. It's fine.
Speaker1:
There's a lot of ethical implications when we do think of these things as more human than they are, or even just human, like in general when they really aren't, even though they resemble being human so much like you were saying. Right. Like this training data is is built off of humanity. It's built off of the digital world. And these outputs are just reflections of the digital world and the discourse that happens on the digital world. And then these the the prompts that are being improved upon are also being labeled and tagged by real human laborers and annotators. And so that's also adding a human element as well. So it's almost like this, this human in the loop technology that that resembles humanity so closely, but also is its own thing entirely that is separate from from humans and is separate from language, but also is language. And yeah, it's it's fascinating how much we trust this thing. Like I've used chat GPT myself and I've, I've put different prompts in and ask different questions and the responses are scarily accurate. Like I totally trust the responses. This would have been a great gimmick when I was a kid to show somebody like, Well, look, you can ask it any question and it gives you the answer. Like this is like Peter answers, but like it's actually real.
Speaker2:
If you haven't done that within the past week, I definitely.
Speaker1:
Have done.
Speaker2:
It.
Speaker1:
But yeah, there's, there's, you're totally right. Like there's, there's so much trust that we place in this technology because it appears to be so human and so trustworthy. And then we have experts like Emily and Casey telling us, like, be wary of that trust, you know?
Speaker2:
Yeah, I don't know what to do with this intellectual property idea and like the legal element to and how much lag that the legality has. I mean, maybe we need to do a follow up episode with Casey to talk about this, because I just it's hard for me to wrap my head around as someone who's not a legal scholar of like, well, what do you what do you do to set precedent around this and copyright and all of that? And so that that also just like worries me in kind of a fundamental like societal level, but that's I don't know, that's above my pay grade, I guess, a podcast this week. But just any any last comments or thoughts?
Speaker1:
I think my last comment is just going to be an invitation for all of you to check out the show notes for this episode. If you don't usually check out the show notes or if you do usually check out the show notes for these episodes, we always curate a bunch of links that are mentioned during the interview and also ones that are just related to topics we discussed in the interview. And there was a lot of really awesome, useful resources that were brought up by both Emily and Casey. So there's there's a whole big list of resources for you all to interrogate some of these topics even further and to really educate yourselves. And in the spirit of algorithmic literacy and improving our information literacy online, this is just really a great starting point to facilitate the conversation around Chat GPT and to try to get to more responsible discourse around this topic.
Speaker2:
For more information on today's show, please visit the episode. Age at radical a reorg as just just said. That's where you'll find all the show notes that you could ever possibly need.
Speaker1:
If you enjoyed this episode, we invite you to subscribe, rate and review the show on iTunes or your favorite pod catcher. You can catch our regularly scheduled episodes the last Wednesday of every month, and sometimes there are bonus episodes in between. Otherwise, you can join our conversation on Twitter at Radical I Pod. You can find us on LinkedIn at the Radical AI podcast. And as always, stay radical.
Sonix is the world’s most advanced automated transcription, translation, and subtitling platform. Fast, accurate, and affordable.
Automatically convert your mp3 files to text (txt file), Microsoft Word (docx file), and SubRip Subtitle (srt file) in minutes.
Sonix has many features that you'd love including secure transcription and file storage, collaboration tools, enterprise-grade admin tools, automated translation, and easily transcribe your Zoom meetings. Try Sonix for free today.