Episode 23: Designing for Intelligibility: Building Responsible AI with Jenn Wortman Vaughan
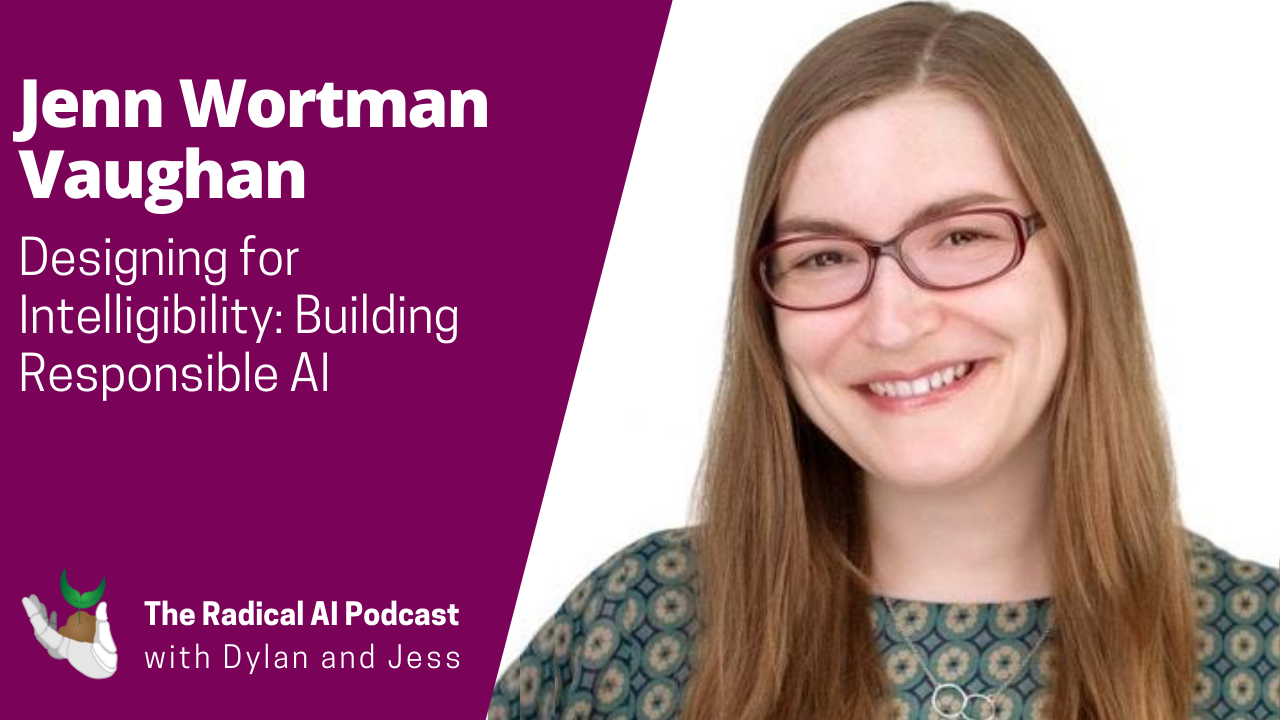
What are the differences between explainability, intelligibility, interpretability, and transparency in Responsible AI? What is human-centered machine learning? Should we be regulating machine learning transparency?
To answer these questions and more we welcome Dr. Jenn Wortman Vaughan to the show. Jenn is a Senior Principal Researcher at Microsoft Research. She has been leading efforts at Microsoft around transparency, intelligibility, and explanation under the umbrella of Aether, their company-wide initiative focused on responsible AI. Jenn’s research focuses broadly on the interaction between people and AI, with a passion for AI that augments, rather than replaces, human abilities.
Follow Jenn Wortman Vaughan on Twitter @jennwvaughan
If you enjoy this episode please make sure to subscribe, submit a rating and review, and connect with us on twitter at @radicalaipod.
Relevant Resources from the Episode:
Book chapter on “A Human-Centered Agenda for Intelligible Machine Learning”
Tutorial on Transparency and Intelligibility Throughout the ML Life Cycle
Datasheets for Datasets by Timnit Gebru, Jamie Morgenstern, Briana Vecchione, Jennifer Wortman Vaughan, Hanna Wallach, Hal Daumé III, Kate Crawford
Microsoft’s AI, Ethics, and Effects in Engineering and Research (AETHER) Committee
Women in Machine Learning Workshop, on Twitter @WiMLworkshop
Women in Machine Learning and Data Science Community, on Twitter @wimlds
Women in AI Ethics Community, on Twitter @Women_AI_Ethics
Transcript
JWV_mixdown.mp3 transcript powered by Sonix—easily convert your audio to text with Sonix.
JWV_mixdown.mp3 was automatically transcribed by Sonix with the latest audio-to-text algorithms. This transcript may contain errors. Sonix is the best audio automated transcription service in 2020. Our automated transcription algorithms works with many of the popular audio file formats.
Welcome to Radical A.I., a podcast about radical ideas, radical people and radical stories at the intersection of ethics and artificial intelligence. We are your hosts, Dylan and Jess. In this episode, we interviewed Dr. Jenn Wortman Vaughan, senior principal researcher at Microsoft Research, and has been leading efforts at Microsoft around transparency, intelligibility and explanation under the umbrella of Aether, their company wide initiative focused on responsible I. Jenn's research focuses broadly on the interaction between people and A.I. with a passion for A.I. that augments rather than replaces human abilities.
Just a few of the many topics that we cover in this interview include what are the differences between explain, ability, intelligibility, interprete ability and transparency? What role does pessimism play in the creation of responsible machine learning models? What is human centered machine learning? Should we be regulating machine learning transparency? And what is the difference between ethics and responsible?
This episode was very special for Jess and I because Jen, along with some of the other guests that we've had, is one of those people who are the people that have been publishing in this responsible AI field for a number of years and are some of the reasons why we started this project in the first place and some of the first names that came up on our list. So Jen is just a huge hero of ours and we are just really excited to share her wisdom with all of you.
And we both learned so much, especially around how to take the theory around responsible AI and really make it operationalized. We are so excited to share this interview with Jen Wortman. Bond with all of you.
And we are on the line today with Jen Wortman, Vaun, Jen, welcome to the show. Thank you. So excited to be here. Yeah, absolutely. It's great to have you. And if you could just start us off today by telling us a little bit about you, we would love to hear what motivates you as a researcher in life and your work in general.
Ok, sure. So broadly speaking, I'm motivated these days by the idea that A.I. systems are really, truly popping up everywhere. They're impacting people's day to day lives in ways that are big, in ways that are small. And as someone who's a natural pessimist, I'm just completely terrified of all of the ways that we are going to mess this up. Right. So we've all seen the hype. We know that A.I. systems can lead to denigration. That can lead to people being unfairly denied resources. And even more commonly than all of that, they just don't work as well for some people as they do for others. Right. So speech recognition systems don't work as well for people with particular accents or people with disabilities that affect their speech. But there are tons of more subtle examples of this to. So I'm actually a machine learning theorist by training. My training is improving algorithms, proving theorems, essentially, and this means that I'm trained to poke holes in arguments. And when I hear about A.I. systems, kind of that part of my brain that just picks holes and things really starts going to work. And so I guess one way I would put this is that I'm motivated to teach other people to try to be just as skeptical as I am so that we can build products that work well for everybody.
That's a that's a question that I have about pessimism in general. What do you think is the role of pessimism, maybe in research in general, but also specifically about machine learning right now? Is it just in separating the hype from the fact or is there more to it?
That's a good question. And as a natural pessimist, I like to think that pessimism has a large role to play in machine learning these days. Part of it is what you were saying about separating hype from fact. And there is so much hype these days around add Bradley, but it also comes up just in the day to day practices of data scientists and machine learning practitioners in their work. And I think we'll probably get into this more later as we really start digging into some of the work that I and others have been doing around intelligibility in machine learning. But part of how I think about tools for model intelligibility is that we want these tools to be kind of uncovering places in the data or places in the model where people should be pessimistic and should take another look.
And before we dive deep into the research that you're doing, unintelligibility and explain ability for our listeners who are a little bit new to this space, could you give us a bit of a 101 on what intelligibility is, what explain abilities and what transparency is, maybe the differences between them, the contentions of those definitions and the small community? You know, just easy, easy definition, dictionary stuff. I'm sure it's not difficult at all.
So that is a great question. And this is something that's really a constant source of frustration for me. So I'm somebody that cares a huge amount about terminology and about consistency and about getting all of these things right. And there's essentially no consistency in the literature right now around all of this terminology. There's a huge amount of debate among researchers and policymakers and machine learning practitioners. And I also have really strong opinions about all of this. So I will tell you kind of my view of the breakdown here, which is based really heavily on the European Union's High-Level Expert Groups few. So there's this high level expert group that broke down transparency into three components. I find this really useful. So the first component of transparency by this breakdown is traceability. And this means that the people who develop or deploy machine learning systems should clearly document their goals, their definitions, their design choices, assumptions and all of this. So I kind of think of traceability as being transparent with yourself and with your own team about what you're doing. And this is kind of foundational because you can't be transparent if you haven't documented what you've done. Right. So an example of what I'm talking about here would be something like the this project on data set documentation that we have going on at Microsoft, the data sheets for data sets project this. The idea here is that we want to encourage data set creators to capture all sorts of information about the motivation behind their data sets and about how these data sets were collected and cleaned and labeled and preprocessed and all of that other information that tends to get lost and forgotten after a couple of months.
There's kind of two goals here. So one is to help data set creators catch unintentional assumptions that they're making and potential biases in their data before it's too late to do something about this. And the other goal is to help data set consumers. So the people who are taking this data and building models off of it understand whether a particular data set is right for their needs. So that's number one. The second component of transparency is communication. So people who develop and deploy machine learning systems should be open about the ways that they use machine learning technology and also about the limitations of their technology. Now, something like data sheets could be used for communication. If they're exposed to end users, though, that wasn't our original intention, but other examples that you hear a lot about are things like Google's model cards or the fact sheets that IBM has put out. And for some of our products at Microsoft, we've put out transparency notes, which are kind of the same idea, basically documentation getting into characteristics and limitations of our products so that end users and others can understand.
So that's to the third component of transparency is what I personally like to refer to as intelligibility. The idea here is that stakeholder's of machine learning systems should be able to understand and monitor the behavior of those systems to whatever extent is necessary in order to achieve their own goals. So this is where there's a little bit of disagreement. I would say there's disagreement in all of us, but this is where my view differs the most from the High-Level Expert Group. So they call this explain ability. But I tend to find the term explain ability a little bit to limiting, because I think that there are sometimes ways that we can achieve intelligibility that are not necessarily just giving explanations. So I don't really like that term. Also, sometimes in the machine learning community, this is referred to as interprete ability and I've used that in some of my own work. But lately I just prefer the term intelligibility because I think this really emphasizes the human component. And in particular, you know, if you think about it, you can't really be intelligible without being intelligible to someone. Right. So it kind of emphasizes that there's somebody out there who needs to be understanding what you're doing. And if you've heard of tools like shop or like line or Microsoft's interpretive multitool, these all kind of fall into this intelligibility bucket.
So we envision this podcast as talking about the ethics of some of these things. And I'm wondering if you could talk about kind of what's at stake here, either ethically or morally. Why does it matter what we call these things? And maybe in general, why does transparency matter in these systems?
Yeah, so I tend to try to avoid the word ethics. But I think a lot about this in terms of just responsible A.I. and this includes things like, you know, building systems that are more fair, are more safe or more reliable and so on. And for this, I really do think that transparency is key. And the way that I think about it is that, you know, this is kind of common view of machine learning as this fully automated process. So you think of machine learning model taking some data and kind of extracting predictions from this data. But if you look at what actually happens, I would argue that people are really at the heart of every stage of the machine learning lifecycle. Right. So there are people who are defining the tasks that we're using machine learning to solve in the first place. There are people generating data sets in this data. These data sets are often about people capturing information from people. There are people who are making the decisions about how to preprocessed and clean and label this data, making decisions about which models to use, what to optimize when you're training these models. And in the end, once you have a system, there are often people who are using that systems to make decisions based on your models output. These decisions, of course, impacts more people. Right. So you kind of think about the machine learning lifecycle from this perspective, then. I think it's just clear that thinking about responsible requires a human centered perspective. And to me, that means thinking about responsibility. I mean thinking about stakeholders like the developers, the users and ultimately the people who are going to be affected by these systems. And so once you kind of frame everything this way, I would argue that it's just clear that building machine learning systems that are going to be reliable or trustworthy or fair or any of these things requires that all of these relevant stakeholders have at least a basic understanding of how they work. Right. So this is kind of where transparency comes into the picture in my mind.
So when I hear about multistakeholder systems and transparency especially, it reminds me of this sort of catch 22 that exists in the email community where people like to game the algorithms. And the example that comes to my mind is the Twitter algorithm, the trending algorithm for topics that are trending and how a lot of politicians especially and really important people in the world will try to utilize figuring out these algorithms to game them and become trending, even though that might not be reflective of what's actually happening in the world. Same is true for, you know, YouTube video recommendations and social media recommendations, things like that. And so I'm curious what your take is on how we can be transparent and open to stakeholders and users in systems that. You know, it might be a little bit harmful to be overly transparent and open about what's actually going on behind the scenes.
Yeah, that's a really good question. So there are certainly trade offs, as there are with all facets of responsible A.I. And there are cases where just being fully transparent can be harmful. So this could be because of the type of situation you're bringing up where people are trying to game the system. It could be because a model is developed using proprietary techniques and companies are just worried about giving away too much information about their model. So there are some potential downsides here. But I would say for the most part, considering the, you know, getting around these downsides typically would just involve considering the trade offs and weighing them and finding some form of transparency that is appropriate for the situation, keeping in mind the whole picture and kind of balancing benefits and harms. So in a particular case, if you're worried, say, about a model being proprietary and not wanting to give away too much information, you might choose to be transparent about the particular features or classes of features that we're used to train a model but not be, you know, fully exposing weights or fully exposing the details of the model or fully exposing the data that you use to train the model there. Just all sorts of balances that can be made here.
I wanted to go back to this concept of a human centered approach, and sometimes we talk about making sure there's a human in the loop and those kind of models. And I'm wondering if you could say more about when you say human centered approach, what that looks like in practice.
Yep. So that's a good question. And I have something a little bit different in mind. I guess what I'm talking about human centered approaches here, although I think humans in the loop have their place as well. So when I talk about taking a human centered approach to transparency or to responsibly AI more broadly, what I'm really thinking about is the fact that when we're developing or evaluating tools, we need to be doing this with the particular stakeholders that we have in mind, front and center, and in context for those particular stakeholders so that we can make sure that they're achieving their goals. And this is something that I think there hasn't been enough of it in the machine learning community. If you look at the literature uninterpretable or unintelligible machine learning models or techniques that have come out, especially those that were coming out several years ago, there is kind of this this common way of writing papers where people would come up with some way of generating explanations or some sort of simple model and just kind of declare it to be interpretable without ever stopping to define what interprete ability means, who it's interpretable to, without actually testing this on real users or any of this. And this is kind of the perspective that I'm pushing back on when I'm arguing for human centered approaches. So let's get a little bit more concrete about this. One example of where we tried to do this is on some work that I have. That was Linky this year on interpreting interoperability in this work, we focused specifically on data scientists and we wanted to look at data scientist in the context of day to day tasks that they are trying to achieve.
So things like debugging a model. And we asked how these data scientists understand and use existing interoperability tools, which seems like a simple enough question and something that you would think there would be a lot of work on. But this is actually something that is really challenging to look at for a number of reasons. So first of all, it requires expertise both in the mathematics underlying machine learning models and in HDI. So human computer interaction or other kind of human centered approaches. It also requires knowledge of both the academic literature on interoperability and on day to day engineering practices and data scientists. We think that. To do this well and get inside, you want to have kind of a mix of qualitative methods so that you can understand the nuances of how these tools are used in context, but you also want to mix these with quantitative methods to achieve scale. And we wanted our study to mimic a realistic setting that a data scientist would face. But we wanted to do this without it being too burdensome or time intensive. So we wanted it to take no more than an hour of someone's time. So it was kind of a combination of factors, makes this really challenging to do. And the first thing that we did in trying to work on this project is to gather an interdisciplinary team where we had of researchers, machine learning researchers, researchers and data scientists working together.
And then what we did in our study is to give data scientists a data set and model to play with where we had inserted inserted some kind of common data science traps into the data. And we tried to see whether these data scientists would become suspicious about these traps when they were given interoperability tools that they could use to kind of see what the model is doing. So this is what going back to what we were talking about earlier around, you know, pessimism and suspicion. We're trying to see if we can make these data scientists suspicious. And at a high level, what we found was that while these tools were sometimes helpful, both of the tools we looked at generalized idea models and shocked both of these resulted in over trust and misuse. We found that the data scientists who participated in our study weren't able to accurately describe the visualizations that these tools were showing them, even after we gave them a kind of standard tutorial. And we also found that just the very fact that they had an explanation made them more confident about the underlying machine learning model, even when it should have been making them suspicious. So the explanation was showing something that should raise suspicion. But just the fact that they kind of had this explanation was causing them to justify what they were seeing and actually trust the model more.
Well, yeah, that's really interesting is I feel like there's several sides of the coin here as we're talking about multiple stakeholders. And so one of the stakeholders is the engineers. And you just kind of explained how we explain things to engineers and to the data scientists. And then what I'm also curious about is how we explain things and make interpretable machine learning models for the end users, especially those who don't have a coding background and might not even understand how machine learning works at all. And so I'm wondering if you have maybe a concrete example of how we might try to explain something to a user that's understandable and interpretable and authentic so that they trust the system and the platform, but then also without pushing an agenda or pushing a user towards something. Yeah.
So that is a good question. It's I don't think that this is something that is a solved problem for sure.
It's something that people are still thinking about a lot in my own work. So actually, the first project that I worked on in the space of interprete ability was this project that was led by our former postdoc for Subsea Center. And this project was one that was looking specifically kind of at laypeople as the stakeholder of interest as opposed to data scientist in the study I was talking about a few minutes ago. And there we were kind of looking at the impact of factors that were kind of commonly just spot in the literature or just claimed in the literature to influence the interoperability of a model. So we were looking at the number of input features to a model where the idea is that if there's a small number of input features, it should be more interpretable than if there's a large number. And we were looking at the kind of transparency level of the models. Is it clear in the sense that you can see the model internals? So this is sometimes referred to as glass box or is the model a black box where you just can't see what's going on at all? And we found that participants who were shown this class box model with a smaller number of features were better able to simulate the model's predictions. So they did, in some sense have a. Better understanding of what the model is doing in the bay could kind of simulator guess what the model is going to predict, which was reassuring. But really surprisingly to us, we found that increasing the level of transparency. So going from blackbox to clear and going from a larger number of features to a smaller number actually reduced people's ability to detect and correct for cases in which the model made a mistake.
And this was kind of surprising to us, because the sort of common thought is that if you make the model more transparent, more interpretable to to people in these ways, then they should be better able to reason about the model and kind of correct for it when it's doing something that's clearly wrong. But this is not what we are seeing. And we have some evidence from our studies that this was due to some amount of information overload like we were just showing, even though it's kind of a small model, the amount of information that we were showing to people was possibly overwhelming them to the point that they just weren't stopping to look at the details and see where the model was making a mistake. So this study does not answer your question about what it is that we need to be showing laypeople in order to get them to understand models. But I think points out the complexity of this question and the importance of running studies, in any case, to see whether any proposal helps the stakeholders in mind to achieve their goals. Because it's not always the case that our intuition about what makes something interpretable is right. And it can be the case that, you know, we think that we're providing people more information or some sort of explanation or something that will help them. And actually we're not helping them at all. We're having kind of the opposite effect.
So what do we do, John? How do we solve transparency, I guess more specifically?
So you're you're coming from being a Microsoft Research, which has a very particular role to play, along with other research groups in an industry and also in the academy. But then we also have government stakeholders. We also have end user end users as stakeholders and we have a lot of folks.
So who I guess who is responsible and who is accountable for these algorithms in terms of transparency?
I think this is still something that is being sorted out and being debated and, you know, I think I should emphasize here that I'm completely speaking on behalf of myself here and not on behalf of Microsoft. So this is all just my opinion. But, you know, the question that you're raising seems to be getting at the issue of whether we should be trying to, you know, regulate transparency or whether we should be thinking about standards for transparency or things like that. If I'm understanding and, you know, I think personally this is really complicated because of the fact that there's just never going to be any sort of one size fits all solution to transparency. So it's always going to be the case that the correct approach depends on the people involved. It depends on the domain and it depends on all sorts of context. And because of this, it kind of worries me a little bit to think of regulators kind of jumping in and trying to propose something that's a bit too stringent. I do think that this could make sense in certain critical domains. So if you think about something like housing or if you think about machine learning for loans or one of these, these are domains that are already heavily regulated. So in that sense, I think it might be a smaller and more natural step to try to kind of standardize what's going on there. But just more generally, I think that we're going to need a lot of flexibility in this space.
So when we talk about this space and we talk about your research specifically, Gen, what are some of the goals here? Do you think it's more along the lines of educating the end user, explaining things to the end user, or educating and creating more interpretable tools for the data scientist, more for regulation, standardization? What is your hope for the future?
Kind of all of the above, right? So there are so many ways that I think that transparency and intelligibility can help in creating responsible AI systems. So I think the big one that I think a lot about in the near term is what I already brought up before about how being data scientist debug models and just catch problems in models and in data because, you know, essentially every data set out there has some problems in it. And it's really important to be able to find these and figure them out. So I think that this is a place where, you know, intelligibility, tools in particular have a huge role. But beyond that, you know, these types of tools and techniques can be useful to domain experts like doctors or scientists who are trying to build machine learning models in order to understand some phenomenon in the world. They're used frequently. In these cases. They can be useful for proving that you have met some particular regulation, although I think this area is still a little bit fuzzier and nobody knows exactly what this is going to look like yet. They can be used for helping end users of systems gain trust in the system or figure out that they should not gain trust in the system.
They can be used in other places within the machine learning development lifecycle. So an example I've seen come up is you can use these model interoperability tools to explain to a designer who's involved in a system what a model is doing so that this designer can better communicate it to the end users of the system, something like this. I think there's kind of endless possibilities here. And this, again, just emphasizes why there is no one size fits all solution and why there is going to be like years more work in the space that's kind of wide open and just gaining more importance all the time as machine learning becomes more common and as it becomes the case that the barrier is being lowered to being able to build more. Machine learning models, so as we're kind of democratizing machine learning, making it be the case that you don't need any formal training in machine learning in order to develop your own models. This is just making it like more and more important that we're giving people good tools that they need to understand what's going on.
That's something that's really exciting for us both as students and as folks. Doing the radical AI project is how wide open some of these spaces are, even down to the some of the language that we're using, which you've broken down here. And I want to circle back to something you said at the beginning of the interview when I asked about about ethics. Right. And this concept of ethics versus responsibility versus all these other terms that are kind of in conversation with each other but have particular baggages and connotations and things like that for us. We're still trying to figure out like what what do we call this this thing? And I'm wondering if you could say more about how you see the difference or why you you shy away from ethics versus responsible.
Yeah. So I think a big part of why I like to avoid ethics is that, first of all, this term has a lot of baggage associated with it. And, you know, when you say ethics, some people start getting deep into philosophy and kind of want to understand the tradition that you're coming from and all of that. And I feel like most of the time when we're just talking at a high level about ethics, that's not really what we're getting at, although that's a part of the picture. But I think if we're talking to the people, say, in companies or in government, but people who are actually out there, building systems like this view is not going to resonate with them as much. And I also feel that there's kind of an associated value judgment that comes with talking about ethics. And people tend to get very defensive once you get into the territory of value judgments. And, you know, they either claim that, of course, what they're doing is ethical or, you know, it's not their problem to be thinking about ethics or any of these common reactions where I find that if you frame this in the context of responsibility responsibly, I it's like. Of course, we think that the tools we're being that we're building should be used responsibly.
This just seems like a much kind of more natural and agreeable goal.
And it's also something that I feel we can get a little bit more concrete on.
When we talk about ethics, it's not super clear, like exactly what you're getting at there when we talk about kind of specific responsible principles of things like transparency or fairness or safety, reliability, any of these types of things. I mean, they're still very fuzzy, like we could debate for hours what fairness means and not get anywhere.
But I think we're getting at least a little bit more concrete and actionable as we talk about this term, responsible to and as responsible researchers in this space. I'm curious a little bit about your journey, Jen, and how you went from a theory, an algorithmic economics background to I think you said it in your own language, in an email to Dylan and I, to someone who preaches about human centered approaches to machine learning. So could you tell us a bit about what what your journey was in the space and how you landed where you are?
Definitely. So I can ask this question a lot. So I'm just going to ramble at you for a while now.
But I do get asked this question all of the time because I think that my research director has been a little bit nontraditional.
So let's see. So I guess going way back, I wanted to be a computer scientist all the way back since I was in high school. So that that part has been very traditional. I have been computer science all the way partially. You know, I think this is because I just loved, like, doing little logic puzzles when I was little. But partially, I also credit this to the fact that I had a female teacher for my first programming class in high school who really went out of her way to encourage me. And I think that this probably had a really huge impact on my career. When I started undergrad, I knew I wanted to do computer science and I spent a little bit of time kind of working part time in industry and had some fun with it. But when I was actually really passionate about then was computer graphics. So I basically, you know, made a fancy ray tracer for a class that I was taking and thought it was the coolest thing ever. And I was like hooked from there. That was what I wanted to do. So I applied to a master's program so that I could learn more about computer graphics. And the only program that I got into was at Stanford. So that is where I went. And when I arrived there, you know, I knew basically nothing at all about research, but all of my friends there were doing research and I was really curious about it.
So I went to talk to one of my graphics professors about research options, and I was, let's just say, harshly rejected on the spot to the extent of, you know, you're a nobody. Why are you talking to me? Get out of my office type of thing. I was a bit fragile at the time, so maybe this is a little bit scarier in my memory than it was in reality. But that was basically my takeaway from the exchange. And I came out of this like really questioning whether computer graphics was right for me or not. So meanwhile, in a couple of close friends who were working in algorithmic economics, which is the term I used, other people say economics and computation or algorithmic game theory or multi-channel systems, other terminology debate here. And they encouraged me to take a class in this area with you have Sean. So I did. And I just loved that class. So first, it was my first exposure to game theory, which I loved. It also included a bunch of formal logic, which, as I said, I loved. So I was just kind of hooked on this right away. And at the end of the class, you have asked me if I want to research position in this group. So I kind of jumped on it and started getting into research from there.
So I did that. I ended up switching my master's concentration to A.I. because that's where algorithmic economics was lumped at Stanford. And this meant that I kind of spent the second year of my master's taking all these classes and machine learning and Bayesian networks and other A.I. courses and learning all about that, which I also loved. So I guess the outcome here was I discovered that I really like doing research. I also, maybe more importantly, really didn't want to get a real job. So I decided to go for these programs from there. So when I started my PhD, I was interested in these two. So algorithmic economics and machine learning, and there are only a couple of people who are active in both areas at the time, and Michael Kearns was one of them. So I went to work with him at Penn for my Ph.D. I briefly started out working on applied machine learning problems, but it became clear really quickly that applied machine learning was way too messy for me and too ad hoc. And I just found that completely unsatisfying and distasteful and wanted nothing to do with it. So I was trying instead to learning theory where everything is really like clean and precise and seemingly objective. Right. So just to give a little bit of background for people who aren't familiar on these areas. So in both learning theory and an algorithmic economics, every paper starts by defining some model of the world.
So in learning theory, a model usually captures where your data is coming from. So, for example, is it ID from a fixed distribution? Sometimes it captures things like what actions your learner is allowed to take and algorithmic economics. The model will often include something about the behavior of agents in a system. So agents may be people, they may be other entities. As an example, a model might say that agents are bidding in an auction and they have some budget and some sort of well-defined preferences or beliefs, and they take actions to maximize their expected utility. So this is kind of a model. And in both of these fields, defining the model is kind of the artistic part of the research process. So different researchers and the community of sort of different aesthetics for the types of models that they prefer. And this is the part where you have artistic control for lack of a better term. We know that these models never capture the complexity of the real world, but ideally they should be defined to capture key elements or key parameters of interest. So you can reason about the impact of these things. So, for example, how many data points or how does the number of data points that you have impact the error or the function that you're trying to learn this type of thing? And once you define a model, everything else is kind of seen to be objective, right.
So in other words, all of your assumptions are explicitly laid out in this model and everything else, all of the theorems you prove, everything else just mathematically follows from those assumptions and about sort of the kind of fundamental of what my training in theory was about. OK, so I am getting to your question, so let's jump forward a few years. So I guess from early in my PhD, I was interested in the interaction between people and systems. But since my training was in theory, the people that I was talking about were usually mathematically ideal people with well defined preferences and beliefs who behaved in well-defined ways. One of the areas that I did a lot of work on over the years was in crowdsourcing. So if I if I lump all the work I did and prediction markets into this crowdsourcing buckets, I spent about a decade working on crowdsourcing. And on the learning theory side, I was interested in things like how to aggregate information or beliefs from groups of people on the algorithmic economic side. I was interested in how to incentivize these people to report their beliefs truthfully and produce higher quality data. All of this sort of stuff. And so back sometime around 2014, I had been at Microsoft for a couple of years and I just written a paper on incentives for crowdsourcing.
And I was discussing it with my colleagues, Didsbury, who is now in Redmond, but at the time was in New York and sat close by to me in the lab. So it is an expert in designing behavioral experiments and did a lot of experimental work with cloud workers in particular. So when I told them about my model, he immediately dismissed it and pointed out that it totally went against how real proud workers behave. So I kept kind of pushing him on this because I wanted to know how to refine my model to make it more realistic. So one thing led to another here, and I ended up collaborating with Sid on an experimental project in which we dug into how proud workers actually respond to incentives. And I was able to use this to define my model. So I was happy in the end. And I ended up kind of collaborating with set on a couple of these projects and just generally getting more into the sort of experimental work. So fast forward one more time to run 2016. So in 2006. I went to D.C. for one of these panels and society that are happening all over the place now, we see these all the time, but one of the panelists at this particular event made this claim that really stuck with me. So he said approximately soon all of our A.I.
systems are going to be so good that all of the uncertainty will be taken out of our decision making. And I was just completely horrified by this claim. The world is just inherently full of uncertainty and all of our systems and machine learning models have uncertainty baked into them, whether it's explicit or not. So it just seems like completely irresponsible to me to tell people that I could take uncertainty away. So I came back to New York and I was fuming about this, and I immediately ranted about this to my close friend, Hannah Wallach, who agreed with me on this. And we spent the next couple of months just dissecting this claim and trying to figure out, like, why it was that this claim bothered us so much. So for context, you know, this was right around the time that there started to be all of this talk of democratizing machine learning that we were talking about. And it's also around the time that Hillary Clinton's chance of winning the US election was hovering around 80 percent. And the general public was kind of treating this as a done deal and watching all of this play out and just kind of replaying this panelist's quote over and over in my mind, I became really obsessed with this question of how well people actually understand the predictions that are coming out of our models.
And, you know, as I said before, I was a theorist, so I was trained to always state my assumptions really clearly and explicitly. And this whole stating of assumptions was super core to everything that I did. And I was afraid that people may not always understand all of the assumptions that go into machine learning models or the implications of these assumptions or things like the uncertainty behind any prediction. So these worries just led me to kind of discover this literature that was just starting to come out in the machine learning community on unintelligible or interpretable machine learning. And, you know, I got really hung up on the fact that I mentioned earlier that people were designing all these methods without stopping to define exactly what they meant by interoperability or intelligibility. So they were basically proposing all of these solutions without first defining the problem that they were trying to solve. Right. So thinking about this great experience I've had working with said on experiments and crowdsourcing, I started talking about this problem with colleagues, with backgrounds in psychology and other human centered fields. So colleagues of mine who knew a lot about behavioral experiments and user studies in general, and I started working with them to run some of these experiments to see how interoperability plays out in practice. So we started with this project I mentioned earlier with laypeople on Mechanical Turk.
And more recently, I've gotten into all of this work around data scientists. And that's kind of how I got started on what's now one of the major themes of my research agenda.
I'll finish my long ramble by just mentioning that also right around the time that I was getting passionate about transparency, a few of the researchers in my lab started up a reading group on topics to do with fate. So fairness, accountability, transparency and ethics. And I and I also started around the same time dabbling more in the space of fairness. So, you know, fairness, which is an area that immediately appealed to me because like many women in computer science, I already spent a good deal of my time thinking about diversity and inclusion in computer science and in machine learning more broadly. You know, in my incoming cohort of 20, I was the only woman when I was briefly faculty at UCLA. There are three women in my Department of 30 something faculty. When I started at MSR, my lab was 13 men and me.
So I felt like I kind of in some ways spent my entire career thinking about inclusion or possibly exclusion, depending on how you think about it. So this was kind of also just a natural fit.
So I got into that as well. My first few projects in the fairness space were also theoretical projects. So I remember I mentioned that there are all of these aesthetics that go into defining a model. And I think those are really super important in an area like fairness, because creating an overly stylized model can actually result in real harms. When you're talking about something as important as this. And I kind of made a conscious decision that I was comfortable using models to illuminate what can go wrong in machine learning systems. So my pessimism again, but I was not comfortable using these models to try to define these kind of so-called provably fair algorithms. So that was kind of my gateway into fairness. But this is, of course, is another area where human centered approaches are really crucial. And the more I got involved in this line of work, the more it seems to be the case that the problems I'm most excited about are not really problems that can be addressed with theoretical models or with new algorithms, but are problems where we really need, again, these human centered techniques. So once again, in the fairness space, like with transparency, in terms of collaborating with people who have backgrounds in areas like HCI, I think you're actually going to be talking with my collaborator, Michael Medair, about some of our work on a fairness checklist in the next couple of weeks. And, you know, I basically just got into this weird point in my career where almost all of the research I do is through these types of collaborations. And I'm having this kind of identity crisis where I don't even know if I identify as a theorist anymore. But at the same time, I feel like the work that I'm doing is having more impact than anything they've done in the past. And it's just a really satisfying space to work in. So I think I'm OK with that.
Yeah.
Thank you for for sharing your story, especially all the twists and turns along the way and also naming the identity crisis that I feel like you're not the only one right now who is having in this in this space, especially in this A.I. ethics or responsibility space. And part of how we envision the radically AI podcast is naming some of those identity crises that are happening out in the world and then also trying to put some some language to it. And the word that we came up with was this word or I should say came up with the word that we have to continue the tradition of is this word radical? And we are curious for you whether in all these twists and turns, you have come up with a definition for yourself of what radical could mean in the space and then whether you situate yourself in that definition.
Yeah. So I yeah, I have a little bit of trouble with the word radical just because I grew up in the U.S. in the 80s and I feel like I have just too strong of an association with 1980s slang like Teenage Mutant Ninja Turtles era radical here. I also feel like what often when people claim to be doing something radical, you know, if people are claiming too hard that what they're doing is radical, I think it often isn't. It's one of those words with big hype, like disruptive, right? Like in industry, everything is disruptive all the time to the point where I feel like it's sort of lost its meaning.
But I do, you know.
I sympathize strongly with what you're trying to get out here, and for me, if I kind of replace it in my head with non-traditional and I'm more comfortable and in that sense, you know, if I think about it, the non-traditional sense and I think of radical meaning, something like ignoring the norms, even when there's societal pressure to think the way that everybody else is thinking. And that's something that I certainly try to embrace in my work, although I don't know if I always I don't know if I would actually classify anything that I do as radical, but I do try to ignore the norms. And, you know, I I do think that, you know, going back to some of what I said before, a lot of people get into machine learning because they don't want to have to think about people or how their work impacts people. And I think personally that the most important and impactful open problems and machine learning are not technical problems, but they're actually people problems or in some cases even process problems. So thinking about something like data sheets are like the A.I. fairness checklist I mentioned, these are kind of solving process problems. And, you know, that's a little bit outside the norm these days. The second thing is, you know, in academia and I kind of include industry research in that bucket, though, I think it's a little bit more flexible in industry. You do quickly get put into a box in your career and kind of expected to work on one area forever and be an expert in this one small thing.
And I've sort of refused to be put in a box. You know, in some ways this alarms people. So within the last year, I've had people telling me that I'm not doing computer science and questioning if I'm really a computer scientist at all. And, you know, someone else in the community has been kind of trolling the data sheets for data sets project. And, you know, same data documentation is not research and, you know, whatever. There's always going to be this type of attitude if you're doing something nontraditional. But from what I actually see firsthand in industry on a day to day basis, I feel like the types of problems that I'm working on now are more important than anything I've worked on before. And I feel more satisfied than I ever have before. So I think it really just goes down to what your measure of success is. And, you know, it was the case that early in my career, when I was producing more standard machine learning theory results, I was maybe getting more awards and getting more of this kind of formal recognition in my career. And that's kind of valued in academia. But. You know, when you're doing something that's truly different, people don't always know what to make of your work and you don't get the same sort of gold stars. But I still feel like more energized now than I ever did before. And I I love what I'm doing, so I'm okay with that.
So, Jen, as we near the end of this interview, something that we ask of all of our guests is some sort of piece of advice. And for you, when you were telling your story earlier, something that really stood out to me was your experience being the only woman in a room or on a team. And as a young woman in this field, myself and somebody who comes from, you know, software engineering, undergraduate degree, and also being the only woman in a room and on software development teams as well, I really empathize with that. And I'm wondering if you might have a piece of advice for another young woman who's a little bit younger than I am, who's maybe also in her undergraduate degree, either in computer science or related field, who might be the only woman in the room and what you might tell her if she's looking to get maybe into the space.
So I will I will give you the advice that I would give. To two young women, but also to introverts, because I myself am also on top of often being the only woman in the room. I'm an extreme introvert and so, like especially when I'm the only woman in the room, I have a lot of trouble speaking up. And my advice there is that, you know, it sounds a little bit cynical. It is cynical, I guess, but, you know. You might need to you might kind of think that all you need to do in your career is do good work and you'll be recognized and rewarded. And I thought this for a long time. But in reality, this is not true. Much of the time and as you kind of get involved in research, you know, you're going to see people get rewarded for projecting confidence. You're going to see people getting rewarded for talking the loudest. You're going to see people getting rewarded for speaking out first on issues that they don't necessarily understand. And these people are going to be totally mediocre. They're not going to know the material as well as you do. And it will absolutely drive you crazy. But, you know, in some ways, if you want to be successful in this world, then you have to kind of accept this and do what you can to really make yourself heard and make sure that your opinion is coming through and getting value to you.
And this means you need to practice being brave. You need to you know, once you start doing research, practice your research pitch, practice your elevator pitch on people practice projecting this really big vision of what you can accomplish, because you know that when other people are interviewing for the same jobs as you or any of this, they are going to be selling this like huge vision of what they can do. And it's not because they can do more than you. It's just because they're kind of projecting this confidence. And, you know, this is something that it's important to do and just practice speaking up on little things like even when it makes you uncomfortable. So there are a lot of tricks that I use to help me speak up. One of them is that I use a lot of notes. And, you know, I was telling you before the show, I have a massive quantity of notes in front of me so that I can make sure I don't forget all of my important talking points. And even like during meetings, I like to kind of take extra notes and formulate what I want to say so that I can make sure what I'm saying is going to make sense when I actually say it out loud. You can also recruit allies who are in the room with you, find somebody who's sympathetic and who maybe has an easier time speaking up and get them to kind of help you break into the conversation.
There's lots of things like this. And I bring this up because this is something that I definitely did not put enough time into in my own career until honestly, like maybe mid to late 30s, which is way too late. And it's really set me back over the years. And I guess my advice is just don't be like me. Don't let all of these mediocre people who don't know as much as you like, claim success, go in and speak up and, you know, be brave and make people listen to you. Oh, and I I would feel bad if I got through all of this without throwing in a pitch for attending events like women and machine learning to make sure that your you know, to make sure that you are meeting people and learning about all of the other women doing awesome things in the fields and, you know, just making a network there. Because the people that you start networking with, like even if you do this when you're really junior, are going to be the people who are you're kind of going through your career with over the years. And they're going to be kind of some of your best friends and allies as you move forward in your career.
As Jess and I were beginning this radically I podcast, project and organization and all of that, we sat down to talk about like what what we wanted to do. And in that we talked about, you know, who are the people that we that have really brought us into this field. And you were one of the first names that they came to that, among others, to that list. And so just while you're still on the line, we just want to thank you so much for the trailblazing work that that you have done that have allowed us as doctoral students coming into this field to be able to kind of continue that that work that you have started. So on behalf of just myself, thank you so much.
And if folks want to find out more about you or your research, where can they go to find out more about.
Oh, thank you. That's so inspiring to hear. And I mean, I'm very impressed with everything that you're doing with this podcast as well. You've had an amazing lineup of guests, and it's just been really fascinating in terms of finding out about my work. I do have a website that's easy to find and it's reasonably up to date in terms of publications and things like that. But I'm also happy for people to, you know, reach out and be happy to talk to people. You can also find me on Twitter, although I am a little bit resistant to it, but I've kind of been pulled in. Now, if you search for me, you will find me easily.
Well, John, thank you again so much for joining us today. Great. Thank you.
We again want to thank Jen so much for joining us today and for this wonderful conversation in which, again, we covered so much ground.
And I think the thing that is sticking with me the most after this conversation is that comparison between when we call something ethical versus when we call something responsable I or responsible tech and what we do with those labels and what those labels signify. Because I think and I think Jen pointed this out to like what we call things. Matters like what we do with those things matters even more now we design our tech and how we design our algorithms right in ethical ways and responsible ways that matters. But it also matters when we call something ethical versus when we call something responsible, even in terms of like who will listen to it. Right. So, like, just if you and I brand our podcast as an ethical I podcast, which we, you know, originally did like, that's going to bring a certain flavor to it, or at the very least, it's going to bring a particular audience. Maybe, you know, you listener out there clicked on this podcast because you knew it was an ethical EHI podcast. And so I'm curious, would it be the same audience if we had originally started calling it a responsible EHI podcast or a responsible tech podcast or those two different things? And again, like my question to you, random listener out there, like, would you have clicked this? Would you have clicked on this episode if or this podcast in general, if we had branded, as, you know, responsibly I podcast versus an ethical I podcast.
And when I start thinking about that for myself, like when I click on something that says ethics versus moral versus responsible, there are some of those that I would click on more than others because of my background, because of my discipline, because of where I enter this conversation, I think is just really important for us to root ourselves back into, you know, what are the politics of our language. And obviously, I don't mean politics in terms of like what, you know, Republican or Democrat, that kind of thing. Really, what are the politics of, like what we signify and what we signal through what we what we call things like? It was a big deal for Microsoft to say, no, we're responsible. And they didn't say ethically. I for for a bunch of reasons John brought up. But I'm just I'm really sitting with that and obviously wrestling with that a little bit as I try to work some of these things into my own doctoral research as well.
Just what stood out to you from this interview with Jim Bell just to kind of tag along to what you were just saying there? I mean, I totally agree. And I think it's interesting for us, too, because we've been in this dialogue of trying to understand and recognize and reconcile with the narrative that we've chosen to create with this project and also trying to understand the language that's being used in this community in general. And it's interesting because for me, I mean, I feel like when I see the word ethics, I immediately I'm like, oh, I want to look at that. I want to listen to that. I want to read that. And clearly, I'm super biased because I'm doing my PhD research in tech ethics. But if I was to see a podcast episode that was titled Responsible, I then I feel like I'd be less likely to click on that. And maybe that's just because, like, the word responsible is less sexy to me. But but then I don't know. I see a word like radical and like that sticks out to me a lot. And that's a word that we chose for many reasons and reasons that we're still continuing to learn. And so you're right. It is it is really interesting that some people have such I mean, we all have such visceral reactions to the language that we choose to use in this space.
And that language really plays such a big role in who feels welcome to the conversation as well. And and that actually, I think is a good Segway, too. To what my immediate reaction was in this interview with Jen was kind of who is a part of this conversation and who does feel welcome to sit at the table and who feels comfortable to be a part of this discipline and this space and this dialogue. And obviously, the thing that me and Jen kind of started talking about towards the end there was how women fit into this space. And by this space, I mean the computer science and machine learning and AI community more broadly. And obviously, this isn't a new concern. I think that the topic of inclusion and accessibility and welcoming in the computer science community for women is something that continues to unfortunately come up time and time again. But I really appreciated Jen's call towards community basically at the end of of our discussion here, in the end of her piece of advice and really inviting women to join groups like Women and Machine Learning. And another group that came to my mind is Women and A.I.
Ethics, which was created by Mia Dand and is a blossoming community of really incredible women in this space and also with a great mentorship program. And so we'll include links to get involved in that in the show notes. But I really think that there are amazing spaces where everyone in this AI ethics community, but especially people who feel like they might not belong or they're having a hard time finding that sense of belonging. There are communities for you out here to join in this space and to feel welcomed and to find people who have similar lived experiences to you and to. Fawned over those experiences and to be there for each other and to support each other and maybe even do work and research together, and so I like Jen heavily encourage any people who feel like they are really looking for that sense of belonging and want so badly to feel welcome in this space to find those communities. And if you're having a hard time finding them, then reach out to Dylan and I and we would love to help you find them or maybe even just start with our community and see where we can go from there.
Yeah, I think that's a great point, Jess. And I think the only other thing that I wanted to lift up from Jen's interview that's related to that is, you know, the process of finding your voice out in the field. And we come into the field, whatever the field means to you from from different places, whether that's industry, whatever different identities we might be embodying. But Jen's invitation for us to practice speaking up on little things and also her naming just how difficult that can be, because there can be some, you know, some real consequences sometimes. So knowing where you're at, but also knowing where you might be able to stretch yourself and where you might be able to speak up. And especially for those of us who might have some level of, you know, leeway or people look to us as leaders in the groups that we're in or the companies that we're into to really maybe there's extra space then for us to speak up on things that matter. And I also hear it as an invitation to not just let things slide for the sake of things being easier. Right. But how can we say, OK, no.
All right, OK, there is something off here, like even if we don't exactly name or we don't exactly know what is off here, to be able to even begin that conversation in the groups that were so just it's like what you're saying in terms of finding those places of support in systems that we're in or in finding places of support and new systems and taking that risk.
I think Jen's also asking us to not just find support, but then when we do feel supported or feel like we have the space in our home institutions to speak up and to speak out, that we really take that risk to do that. Speaking up and speaking out, just how important that is, even if it may be uncomfortable, because that's a large part of how this changes has happened towards creating responsible systems. And it's a large part of how this change is going to need to continue to happen is through that a little bit of being uncomfortable and speaking up and speaking out when it's, you know, safe and strategic for you to do so.
Exactly. This community is created by us and it can be whatever we want it to be. And by us, I mean everyone, the people who are listening to this episode right now, you whoever's ears we are currently speaking into, the people who are a part of the larger online community, people who are doing research in this space and anyone who interacts with technology at all, we're all in this community and we all are building this space together. So let's make it inclusive, welcoming and warm. And for more information on today's show, please visit the episode page at Radical Eye Dog.
And if you enjoyed this episode, we invite you to subscribe rates and review the show on iTunes or your favorite podcast to join our conversation on Twitter. Apte radical iPod. And as always, stay radical.
Automatically convert your audio files to text with Sonix. Sonix is the best online, automated transcription service.
Sonix uses cutting-edge artificial intelligence to convert your mp3 files to text.
Create better transcripts with online automated transcription. Here are five reasons you should transcribe your podcast with Sonix. Automated transcription is getting more accurate with each passing day. Lawyers need to transcribe their interviews, phone calls, and video recordings. Most choose Sonix as their speech-to-text technology. Manual audio transcription is tedious and expensive. Are you a podcaster looking for automated transcription? Sonix can help you better transcribe your podcast episodes. Rapid advancements in speech-to-text technology has made transcription a whole lot easier. Automated transcription is much more accurate if you upload high quality audio. Here's how to capture high quality audio.
Sonix uses cutting-edge artificial intelligence to convert your mp3 files to text.
Sonix is the best online audio transcription software in 2020—it's fast, easy, and affordable.
If you are looking for a great way to convert your audio to text, try Sonix today.