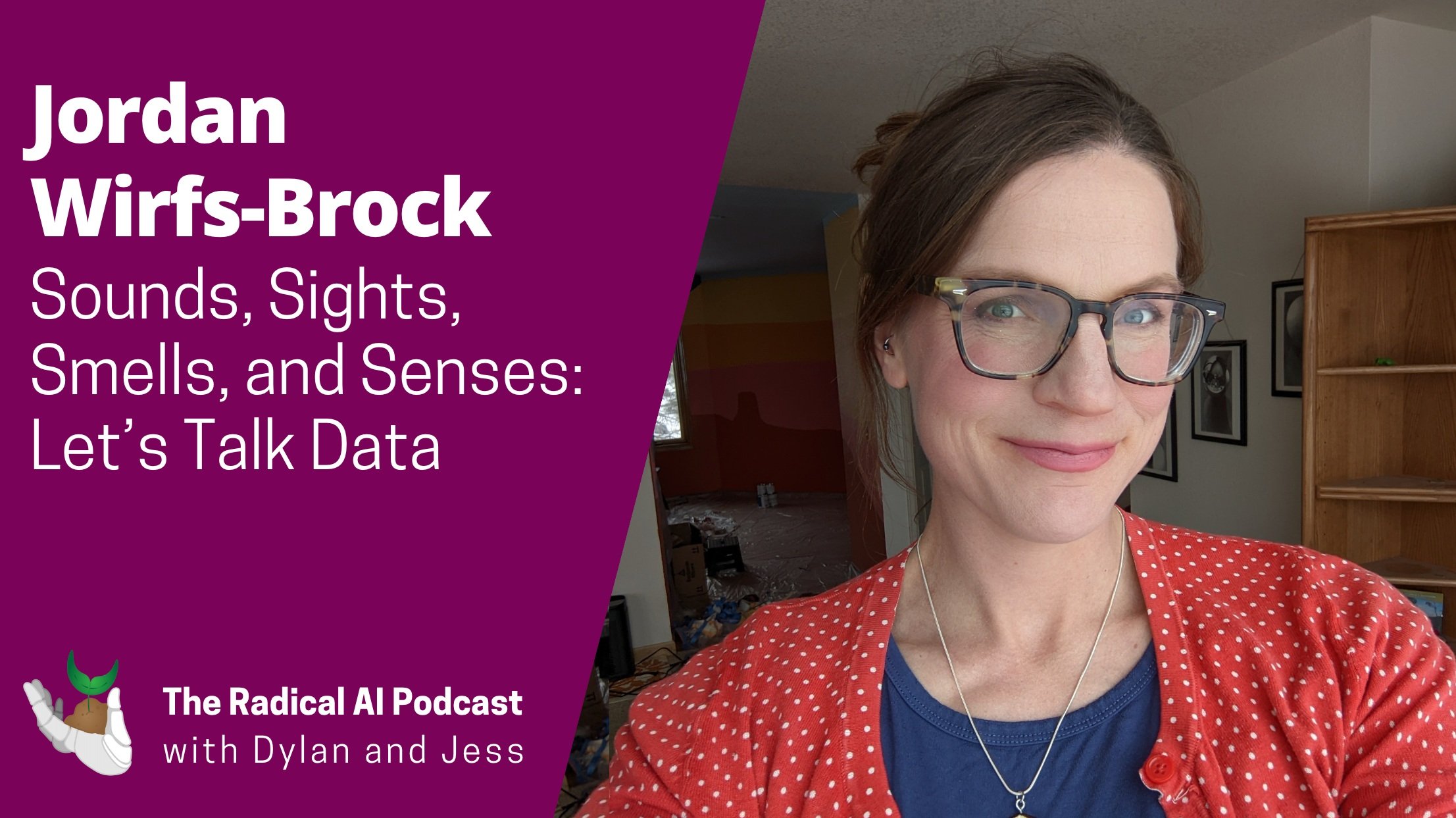
What can our senses teach us about data?
What can data teach us about our senses?
In this episode, we interview Jordan Wirfs-Brock about how we can explore data through all of our senses, especially through the sense of sound.
Jordan Wirfs-Brock recently completed a PhD in Information Science at the University of Colorado Boulder and will be joining Whitman College as an assistant professor in Computer Science in January. Her research explores how to bring data into our everyday lives as a creative material by developing data representations that are participatory and engage all of our senses, especially sound. In the past, she has worked as a data journalist covering the energy industry and as a civic technologist helping non-profit organizations understand their communities through data.
Follow Jordan on Twitter @jordanwb
If you enjoyed this episode please make sure to subscribe, submit a rating and review, and connect with us on twitter at @radicalaipod.
Relevant Resources Related to This Episode:
Yield curve sonification and animation
How to do sonification without coding
An Immense World: How Animal Senses Reveal The Hidden Realms Around Us (Book)
Transcript
data-senses.mp3: Audio automatically transcribed by Sonix
data-senses.mp3: this mp3 audio file was automatically transcribed by Sonix with the best speech-to-text algorithms. This transcript may contain errors.
Speaker1:
Welcome to Radical Eye, a podcast about technology, power, society and what it means to be human in the age of information. We are your hosts, Dylan and Jess. We're two PhD students with different backgrounds researching AI and technology ethics.
Speaker2:
In this episode, we interviewed Jordan Wirfs-brock about how we can explore data through all of our senses, especially through the sense of sound.
Speaker1:
Jordan recently completed a PhD in Information Science at the University of Colorado, Boulder and will be joining Whitman College as an assistant professor in computer science in January. Her research explores how to bring data into our everyday lives as a creative material by developing data representations that are participatory and engage all of our senses, and especially sound.
Speaker2:
And the university that Jordan just graduated from might sound familiar to all of you because it is the same university that Dylan and I are currently attending. The university of Colorado, Boulder. And it's not just the same university, but actually the same Department of Information Science that both Dylan and are currently in, which is how we connected with Jordan several years ago and why we've also known about Jordan's research for a few years now. So although this research is not new to us, it is very inspiring to us. And because she recently graduated, we wanted to take the opportunity to share this awesome and interesting work with all of you. So without further ado, here's to the interview.
Speaker1:
We're on the line today with Jordan Brock. Jordan, how are you doing today?
Speaker3:
I'm doing great. Thank you so much for having me.
Speaker1:
Yeah, no, it's great to have you. Obviously, Justin, I know you from being from literally being in your dissertation defense fairly recently. And so we know a little bit about your research. But besides the title and the blurb, folks out there may not. And so I was wondering if we could just start by you describing what your research is.
Speaker3:
Yeah. So I generally think about it as expanding the things that we can do with data. And I talk about that in terms of using data as a creative material, and that means often doing weird and wonderful things with data. Doing participatory activities with it, using all of our senses to communicate data and really just sort of trying to expand our ideas of who gets to use data, what we include as data, what data is, and then also what you can do with it. A lot of that in the past has been using sensory representations or using sound and physical objects and social interactions to represent data. But yeah, that's, that's kind of the, the high level overview of it in the sort of the most broadest way that I could probably describe it.
Speaker4:
I want to latch on.
Speaker2:
To one of the things that you described in your research, which is what is data itself? Because data is this buzzword that we hear what we're talking about, big data or data in the cloud or personal data informatics, things like that. So how would you describe what data is? Or also is data plural?
Speaker3:
I go back and forth. It depends on the context. I tend to use data is rather than data, but it definitely depends. But I love that you posed this question because I realized that I hadn't actually been doing a lot of reflecting on what data even is. Um, and so yeah, I love, like kind of thinking about this and like taking a step back before kind of diving into what we can do with it. And I don't know, I tend to think like pretty much anything in the world can be data. So like, for example, it, it snowed here last night and I went on a walk this morning and I was like looking at all the tracks in the snow like rabbits and and things like that. And I was like, That is data. Like those tracks in the snow are data. Anything that you can observe out in the world. But then when you ask that question, it was like, okay, so then when does it go from just being a phenomena in the world to being data, Right? Because data is something we have to we have to transform it in some way.
Speaker3:
We have to transform the world into data. And so I don't have like a solid answer, but some of the things that I was thinking about, like, is it the collecting that makes it data? Is it that you're adding structure to it? Right. So you're asking some kind of question in terms of, you know, where are those tracks in the snow? When were they when did they show up? Right. So is it adding some kind of structure so that you can collect it? Um, although that structure can be super loose. Um, yeah. So maybe it's in terms of putting it into some sort of structure that allows you to compare data across dimensions, right? Whether that's time or space or a category. But I don't know, I'm kind of curious, like what your definitions of data are because I just, I love this question of like, what is data? Um, I don't know. So I want to put that back on you. Although I know you're the host, so you're supposed to be asking me the questions, but.
Speaker2:
This is great. No one ever asks us questions. I feel like a fish out of water. How do we answer questions? That's. That's fun. I did not come prepared to this interview to answer these questions. But I will say, when I was hearing you describe what you thought data was, the word that came to my mind was information. I feel like data is any kind of information about anything which sort of aligns with what you were saying. Like it can literally be anything. As long as we can derive something from it, some sort of information, although maybe some data just exists to exist and we don't actually have to derive any meaning or anything from it. It just is. So maybe everything is just data and in some way or another and yeah, don't know. Dylan Feel free to like push back on that too, because that's like a very, like deterministic kind of perspective.
Speaker3:
Data and nothing is data. So you need to have some sort of definition. But I don't know. Yeah.
Speaker1:
I wonder. I appreciated you talking about the, the structure and like the structure and then like, does, does data have to be used Like, does there have to be a user of the data or someone who's interpreting it in order to make it like to render it as data as opposed to just a phenomenon? But on that question of structure, I'm wondering if you can talk about some of the ways that you've structured data, especially when you're talking about like the sensual aspect of it, of like using the senses of bringing that in tactile, Like what does that look like?
Speaker3:
Yeah. And oh man, does someone have to use it like so often? Sorry, I will get to the structure question, but so often, like the way data is used or it's used by someone, that's not the person that collected it. And so I think that that's a separate thing, right? So there's like the creation of the data that like turning the world into data, and then there's the how it gets used. And so often it's used in ways that, like the people that transformed the world into the data never imagined. And so there's so much data out there like waiting for someone to. Go grab it and use it. So in terms of how to structure data, like, yeah, there are so many ways to do it. And as you mentioned, I work with sensory data, right? So like the things that you smell, the things that you hear, the things that you touch, the things that you taste. And one of the reasons I was drawn to those types of data is because they are so hard to structure. Like, you know, if I were to ask you to, you know, today you're going to collect data on everything that you smell, right? How would you do that? We don't have we don't have a good way to use to collect quantitative data on smell, although there are people that that do this.
Speaker3:
But if you were to do this in your daily life. Right, would you come up with some sort of intensity scale? Right. 0 to 10. I'm like, how intense the smell is. You could describe the smell, right? And there we have to then fall back on language and words, and often our language falls short. You could come up with your own system. And so by by posing kind of these challenges of data collection, you start to then explore what structures you might use. And it's through the collection. You then realize which structures fall short and which are which kind of don't do the data justice. And so then you can then rework them. So that's one, that's one example. Um, but yeah, it's, I like to focus on these questions because there is no perfect way to structure data, there is no perfect way to collect data and I encourage people to reflect on that process and that that process is subjective and it is emergent and it really is then imbued in the data itself. Right? You can't you can't you can't distinguish data from the process in which it was collected, but we can reflect on it.
Speaker2:
Okay. Totally unfair follow up question. But if we're speaking about the structures that we prescribe to data being inherently subjective and and imperfect. Do you think that there is any way to represent data that is objective or is data inherently subjective?
Speaker3:
Okay. So so in your initial question of like, what is data? One of the things I brought up was like, in order to you impose a structure so that you can compare it, right, so that you can compare one thing to another. So I can impair, compare like the tracks I see in the snow today to the tracks I might see tomorrow if there's another snow or so I could compare the smells that I smell today with the smells I'm smelling tomorrow. And I think some structures are better than others in terms of meeting the goal of comparing or finding relationships. So they're all subjective, but some are better suited to the goal that you're trying to achieve. And that sounded really I don't know, that sounds really fuzzy and like maybe you can try to try to follow up with some like get some examples out of me. But I think there are some it's all about how well the structure matches the purpose of what you're trying to do with it, right? So if like your goal of collecting smell data is to understand your own. Relationship to the world. Then maybe you describing it in words that only you understand, or maybe you, you know, only make sense to you. That's totally fine. But if your goal of collecting that data is to say, okay, just how is what you're smelling compared to what I'm smelling, then you need to, to collect it in a different way that lets you then make those comparisons. So yeah, I'm not sure if I answered your question. It's deeply philosophical and I think that these are questions that are really hard to answer but that we should all be asking all the time.
Speaker1:
Yeah, yeah. It's really interesting in the sensory world too. I'm thinking about, say, color blindness, where some folks, if you're born colorblind in many different ways, right? You don't have that maybe even the same language or that same reference point. And so there's a translation that probably has to happen, especially in a world that is very much built upon the the assumption of certain ableism. And but I'm curious, you know, do you work with with sound like how does how does that translation work across these spaces where everyone has perhaps a really different reference point that they're starting from?
Speaker3:
Yeah. Yeah. And you, you you're right you bring up a really great point is like we are our sensory worlds are unique to us like we are we have detectors, right? So we have, you know, our eyes and our nose and the little hairs in our ears, which all serve to detect things happening out in the world. But then we interpret them, right? Our brains process them and our brains all process them in different ways that are dependent on all kinds of things biology, cultural experiences, environmental contexts, all kinds of things that influence how you process that sensory information. And I would just make a kind of a plug for like a really great kind of book about this. Ed Yong recently had a book about the sensory worlds of different animals, Um, that it's called What's it called? It's Near Me. It's called An Immense World. And yeah, and it uses this philosophical, philosophical concept of an umwelt, which is basically like the world that you experience. And it's just this idea that, like, the world I experience is unique to me. The world you experience is unique to you. We can never like have a 1 to 1 translation of our our umwelt. But by but by acknowledging the fact that we have a we have a unique sensory experience, we can start to then maybe form some bridges or form some connections across each of our own unique experiences. Um, so then to bring that back to design, right? If you're designing a data representation, it would be impossible to design for everyone's individual context, but you can try to understand some of those contexts and bring those considerations into any design that you do. So I think it's important to yeah, to, to kind of know who you're designing for and what you're designing for, even though, you know, you can never design for every single context.
Speaker2:
Well, it's fascinating because when when you speak about how our sensory worlds are unique to us, I love I love that, that that way to describe our unique experiences. It reminds me of the concept of qualia, which I think you you have a podcast that you host or that you hosted that's literally titled Qualia. Is that that's is that correct?
Speaker3:
I did, yeah. Um, and yeah, it's kind of the, the philosophical concept of experience, right? And sensory experience and what you can observe and experience in the world as a phenomena, um, which is such a slippery concept. And um, yeah, yeah, that was just a fun project I did with a friend of mine who's a sound designer and just loves to bring like complex ideas to life through really insane sound design. That's like way beyond anything that, that I could do. Um, so yeah, that was a super, super fun.
Speaker2:
That's great. Well, I remember when I was in my undergrad, I think it was the last year of my degree, I took a philosophy of the mind course, which was the first time I'd ever heard of Qualia. And the way they described it to me was, um. That there's no way to know if, if I am looking at a red shirt and, and both of you are also looking at at this same red shirt. There's no way to know if my red is the same as your red. Maybe we are all seeing red, but maybe your red looks like green and my brown looks like blue and there's really no way to know. And so I'm wondering in in this world where we all have these unique sensory experiences, but but we are attempting to design for something universal. Do you have an example of some of the design decisions? That might afford our ability to to design for everyone's unique sensory experiences. Even if maybe we're experiencing something different, we can still get the same benefit from a system that we are perceiving data from.
Speaker3:
Yeah. Yeah, that's a that's a really great question. So yeah, I think of this kind of this fact that we all have our own sensory worlds, not as a, as a deterrent or it's like a, it's like a fun challenge, right? And it's an invitation to like talk about those sensory differences, but to get into like, real kind of specific examples. So I used to work in radio as a data journalist, and one of the challenges there is which, you know, as podcasters, you don't know the context in which someone is listening, right? Are they listening while they're driving, which is very often the case? Are they listening while they're maybe out running, you know, with one earbud in so that they can hear the environment around them? Are they listening like, you know, with headphones on and their eyes closed? That's not often the case, but it might be for like super audio nerds and you have to think about all of those contexts and then figure out which one you're going to kind of have as your primary example. And so if you're doing like radio news, right, you have to realize that you have people whose attention is divided. They may tune in and then tune back out. And so you have to then do things like, you know, repeating things multiple times, using signposts to like remind people whose attention might be divided.
Speaker3:
Whereas with the Qualia podcast you mentioned, like we knew we wanted it to be so sound rich and we wanted the sound to tell the story. So we actually said like, you know, a message at the beginning that's like, Hey, you should listen to this with headphones on. And, you know, without distractions, we don't know if people will do that. But that's kind of the way that it was intended to listen. So that's kind of one way where you can sort of you can sort of give some guidance to your audience and they might not listen to it, they might not take it. But you can always kind of kind of communicate your intentions and then know that people will do whatever they want with what you're putting out into the world. But you can but you can be deliberate about kind of, you know, how someone is listening, but also like embracing the fact that people are going to, you know, as your your artists and creators who are putting things out into the world, embrace the fact that people are going to do their own thing with what you put out there. So you can't control people out in the world. But and that's okay. But you can you can take their different contexts into consideration and think about them.
Speaker1:
It's it's fun interviewing a colleague who, you know, just and I have known for for a while and knowing your research for a while but like so we get to ask personal questions about different areas of your of your professional life. But it sounds like you've worn many hats, right? It sounds like you started out as a data journalist that you then moved into both like maybe you were always an artist, but there's this artist component and then also you as a researcher. And I'm wondering for you how the way that you've engaged with data maybe thinking about like sound data, how that's changed depending on what your goal was or your role was in different systems.
Speaker3:
Yeah, definitely different goals of working with data. So, um, so yeah, I used to be a journalist and before that I actually worked in the nonprofit world essentially doing data journalism, but for community organizations and nonprofit groups, basically helping them understand data about their communities. And then as a journalist, I focused on energy data. So like reporting on energy systems, you know, like the electric grid and oil and gas industry and, you know, solar panels and all that stuff. And with both of those roles, the goal with communicating data was almost always to like, get to a point and or to get to an idea and to get to an idea quickly and succinctly and clearly and for as many people as possible. So the goal there was kind of to make data approachable and to have data tell a very specific story. That wasn't always the case, but often say you're doing a, you know, a radio piece about how coal production has gone up and down over time. Right? You need to get that point across clearly. And that was actually the first time I ever used sound to communicate data. That's a technique called data sonification. And so I made a story that was basically it turned coal production weekly coal production into musical notes.
Speaker3:
So when it went up, the notes went up higher tones. And when it went down, that went right. And so and that was a piece where, like I produced it, I produced this cool sound and I like played it for one of my colleagues. And he was like, This is cool, but people aren't going to understand it, you know, Like it needs to have context and scaffolding around it. And so there because my. All was to tell the story about how the how US coal production has gone up and down according to various different historical events. I kind of then told this narration around it and brought the music in and out at those key points. Um, and so yeah, there's a lot of cases where it's like, okay, I need to tell a specific story. Whereas now as a researcher, I use, I use data a lot more to like muddle things or to confuse people or to get people to ask questions or to rethink things. And in those cases, it's not it's not about telling a specific story, but maybe encouraging people to question whether there is a single interpretation. And so that was a project I did with another student at the University of Colorado, Mikaela Frisky.
Speaker3:
Like we collected data about our own lives. So our commutes to get to school every day, how busy we were, and we kind of made data representations of those. But the goal was not to have these representations be understandable. The goal was actually to have them be kind of obtuse and kind of confusing because we exchange them with each other without much guidance. And the goal there was to see like, what other interpretations would this person come up with? Right. So it was to surface these multiple narratives and multiple ways of interpreting data. So, um, yeah, so those are kind of two modes that like, let's tell a specific story and you have to, you have to do that in, in, you know, there's techniques to do that. And then if your goal is to kind of get people to question and explore and wonder, you use different techniques to accomplish that, that type of goal. And there's other there's other ways to other reasons you might be communicating data as well, right? There's you might be doing exploratory analysis, you might be doing prediction like and those all of those different purposes have their own set of techniques and there's a lot of overlap between the techniques.
Speaker2:
But so I'm curious, regardless of the goal of working with data, whether it's storytelling predictions or wonder analysis, the things you were just describing, I'm curious how we can perceive data using all of our senses. And you just described one of the senses with with our our ears and hearing we can you use data sonification and you can hear how data changes through different pitches and tones. And so I'm curious at least, could you describe maybe some other examples of the, gosh, I almost said six senses, the five senses and the other ways that we.
Speaker3:
Know how many there are, because there, there's like, um, like we tend to think about the five senses, but then there's also like your spatial awareness, right? Like how you're oriented, your body is oriented. That's like maybe another sense. And then there's additional other ones. Um, so yeah, it isn't, it isn't like there's a set five, but that's how we traditionally talk about them. Um, yeah. So how can we use all of our senses to perceive data, like to answer this question, like, I mean encourage you to think about how you're already doing that all the time in your life. So because people are like sound, that's so novel. And I'm like, But you listen to things all the time, right? So you're walking down the street, you can hear when a car is coming and then you can hear when it's, you know, coming up behind you and then when it's going away. Or you can hear footsteps and know who's walking towards you. Right? So like, you can probably tell if you live with different people, like based on the or you live with animals, right? Whether it's your dog walking up to you. I have a ten month old. Right? I can tell when she's crawling.
Speaker3:
I can tell when she's, you know, uh, what's it called? Cruising. When she's cruising around? She doesn't quite walk yet. And so you're already using that. And that's data, right? That's data about the position of the people and objects around you. And you're already interpreting sonic data all the time. Um, same thing with, with tactile data or smell data, right? So like, I don't know, you know, when it's time to do laundry, right? When there's visual cues like the, your laundry getting full. Right? That's a visualization or a physicalization I guess it's three dimensional. But then there's also the smell, right? You know, when it's time to like, okay, I've worn that shirt long enough, I'm going to throw it in the laundry pile. Right? So that's using smell data. So, so, so yeah, I always start by saying like, you're already doing this, so how can we do that to then represent abstract data? So let's take something else and then translate it into a sense. So. To to kind of tap into our ability to use all of our senses all the time. And then so but I think you wanted an example of this. I don't know the one that keeps coming to, to my mind is this, this sonification, um, from the Financial Times, I believe.
Speaker3:
And they took something called the yield curve, which is like this really abstract economic concept of like how bonds in the future, whether their value is going up or down, which is basically a way to understand whether the economy is like going strong or whether we're maybe going into a recession anyway. It's this really esoteric concept. Um, and there's this beautiful sonification that also comes with an animated video that one of the journalists did there where he turned it into music. And you can hear like, is it doo doo doo doo doo doo doo doo. And like it basically is turning this super esoteric concept into something that you can hear and learn to listen to very quickly. And he did it through both visuals and animations. Um, oh man, I wish I had like a better one, but that's for some reason, that's the one that keeps coming to my mind. But basically you're tapping into your sensory, um, wiring which can interpret sounds and images to, to analyze something that otherwise would be abstract and esoteric and hard to interpret in, in another form. Um. Yeah. See if I come up with the other ones.
Speaker1:
Well, yeah, the scale of that is what's really interesting to me, because I can imagine, say me making a sound throughout my say like my day, right? It's like when I eat, I make like an eating sound or something. When I wake up, I make a different sound and like, I can record that. And I would not, you know, put it out into the world necessarily for people to listen to the sounds of my day. But I can I can visualize that for myself of like how I would do that. But something that you were just mentioning with, say, like the Financial Times or markets is you have all of these different data points from all these different either people or projects or anything like that. And you're using sound specifically in that case to represent it. And I'm wondering, I guess there's many ways you could represent that data. Like you could just give a graph with a bunch of data points. Is there I don't want to be too utilitarian, but is there like a reason to use sound? For example, or is there a way that this allows us to rethink some of these data points that is particularly useful or different than the norm?
Speaker3:
Yeah. And you bring up a great point. Like sound is not good for every kind of data. And I think that's, that's kind of a something that often get that question is people are like, but why would you do this? And I'm like, Well, you wouldn't always do this. And that's why you kind of it's always important to think about like, what is my purpose? Who is my audience? What is my goal? Um, but sound is good for something. So sound is good for changes over time. So we're really good at hearing something. A tone that evolves or changes. Sound is also really good for spatial things, right? For for, um, you can't, your listeners won't see what I'm doing, but whether something is coming from behind you or in front of you or from the sides. Sound is also really good for rhythm, right? So there's if you have kind of two rhythms that are close but slightly different, your ear is really good at distinguishing those. So. So those are things that sound is good at. So you can think about also engaging ambience, right? So maybe there's something where you want an ambience signal, but then you want to know when something changes.
Speaker3:
That's why we have a lot of audio alarms. So those are some of the things that sound does well. There's other things that sound does not do well, right? So many, many, many channels of information. So you have like ten different sounds. Um, we tend to listen to those holistically. So if you think about listening to a symphony, if you're not trained right as a as a musician or a musicologist, you're probably listening to it as this whole beautiful piece of music. But whereas if you train your ear, you can then listen to like, okay, this is what the trumpet is doing, or you know, this is what the bassoon is doing. But that takes training and that takes like a special kind of attentive listening. So, um, so yeah, there's ways where we can turn multiple channels into a holistic thing or we can kind of break them apart. But that is something that you would, you would need. Yeah. Training and guidance to be able to do that. Oh, and also, I mean accessibility also there are people, right, who who are blind or low vision. And so that's a huge, huge, huge area of, um, sonification applications as well.
Speaker2:
So as you were speaking about the, you know, the sensory journey that we're going on, I was envisioning using the technologies that I use daily that involve a lot of data and number crunching and the the technologies that were coming to mind first were social media apps where I'm sitting on my phone, I'm scrolling, and I was thinking about how most of the the data that I perceive or that I, I intake is, I think through my eyes, I feel like the vast majority of the data that I'm seeing is like visual representation. But then I was also thinking, well, when my phone is not on silent mode, sometimes, like if I like a post, I'll get like a ding ding or like if somebody sends me a message, I'll get like a doo doo doo or whatever the sounds are that come up. And then if my phone was like also on the like the vibrate mode, if I get like a notification, I might also like physically feel it. So I have that tangible sense too. And so I usually keep my phone on silent. So the vast majority of the time the data that I'm getting is just visual and I'm not getting those other senses in. But I'm wondering if do you think that tech platforms could benefit from incorporating more of the senses into our data consumption, and if so, how?
Speaker3:
Yeah, and I definitely think that we could consume more things through sound, and that's something that I always did. I've always been an audio file, so I always liked listening to things. But now as a new parent, I'm doing it more and more. I can't look at screens like my eyes have to be on her and what she's doing as she's exploring the world and, you know, climbing on. Things and about to fall over or pull open some drawer. And so I am finding myself more and more using audio input, whether that's a, um, a voice assistant, which I use a lot, whether that is listening to audio content. Um, but you're right, a lot of times it's not, um, it's not data in terms of as we think about most data, right? So a lot of that audio content is narrative based or it's, um, it's language based. Is there music or it's like language and there's like this whole world in between music and language, um, where I think we can start to explore and use more. And so, um, yeah, I think there are all kinds of situations where your eyes are otherwise occupied. Another one for me, another big, um, context for that is when I'm running, right? When I'm running, my eyes need to be looking at the trail. And so I can't look at things. And there's definitely been times where I'm like looking at my watch and I'm like, Oh crap. And I'm like, you know, tripping. Um, I once fell off a treadmill because I was like, fiddling with my iPod.
Speaker3:
So. So I think there are all kinds of cases where your eyes are otherwise occupied, and there are so many more kinds of audio information that we could be using than we're not. So like, yeah, I totally agree with you. And so just think about all the different ways that you could perceive things. Like one, I like how you mentioned like your own personal data and your like whether it's your social media data or your biometric data or whatever it is you're tracking. Like one idea I've been really taken by is. You could develop your own personal. Audio system. Write your own personal audio representation that only you would know, right? So like you don't know what the doodling means, like or other people wouldn't. So like, you would know, like the doodling means, oh, someone liked your post, but someone who walks in the room, they might not know what it means. So, like, I don't know. I really love this idea of, like, developing your own private sound. Um, I don't know world where you can interpret that information because so much of this is kind of learned over time. So I don't know. I think maybe, maybe that could be like a research area in the future is like developing our own audio information ecosystems that work for us. Right? Because it's not it's not universal. And because because so much of the sounds that exist are we end up turning them off because they don't work for us. But if they did work for you, you would use them more.
Speaker1:
So there's this idea that I've been reading about recently about how like we don't just use technology, but that to some degree technology uses us. Or if I'm thinking about like silicon Valley psychology, right, of like making sure that our devices probably including sounds, are training us in some way to like get that adrenaline rush, get that endorphin rush when we get a notification and things like that. I'm wondering if there are spaces in which sound I don't want to say can be used for nefarious purposes. But whether there's the flip side of what we were just discussing.
Speaker3:
Oh, totally. Yeah, think it can. I don't think of it as nefarious. Think of it as yeah, like fighting back, right? Against. Against kind of like systems that may be using data about you. Um, and that's, that's something that I think is really intriguing is how sound because it is a little bit inscrutable, right? It's, um, you know, so it has that characteristic where it is harder to draw meaning from it or to extract meaning from it. Although I don't know, the field of like computational sound analysis is like. Taking off and soon we'll be able to extract all kinds of data from audio files. But there still is this extent that like sound lets you mask certain things too. And so I think, yeah, using that, I love that idea of like using it to like take ownership over over your data or to do things with your data that other people can't understand and to like embrace that.
Speaker1:
Jordan One question that I have is we know that your next space of employment is at a college and a teaching college. And I'm just curious for because you I think you do think about data and you are employing your research to think about data in a way that is a little not mainstream. I'm wondering how you're thinking about employing this research or what you've learned from it within the classroom setting and whether there's like an academic application as you're leading a classroom and helping people learn.
Speaker3:
Yeah. So I'm going to be teaching in a computer science department and I'm also going to be teaching data science. So one of the classes that I'm going to be teaching in my first semester is Intro to Data Science. And I'm for sure thinking about how to bring in these ideas of like, okay, we always need to think about how data is collected. We always need to think about expanding the ways we represent it. So I'm definitely bringing in those ideas into the classroom and I hope to eventually develop like a multisensory data representation course that does really focus on both collecting data about sensory experiences, but then representing data through sensory experiences. And yeah, I have goals of kind of like bringing more people into to doing data science and into doing computer science as well. And I think that some of these, some of these, some of the work I do like personally, I feel I definitely always have. Like I always get a little nervous. I'm like, are people going to think that this is computer science? Like, is this just like fun activities? And it's like, no, this is computer science. Like anything where we are engaging with data, we're using it. We're thinking about it, we're making things with data like that is computer science. And I'm hoping that the kind of activities I do can bring more people who maybe have thought like, I could never see myself doing data science or I could never see myself doing computer science.
Speaker3:
So to bring more people into the classroom, because I really think that, yeah, it's not I don't know. There's so working in journalism, you know, I encountered a lot of colleagues who were like, Well, the reason that I became a journalist because, well, I love it, but also I'm not good at math and like, yeah, fighting. So it was like fighting back against this idea, you know, that, that there's like people that are good at math and then people that aren't good at math. And I think that's just not at all true. And there's all kinds of different ways of engaging with data. And so, yeah, those are some of my goals. Um, yeah, I would love to teach a course all about sound as well. A course on creative coding that would be, I think really fun or on live coding, basically doing programming as an expression of art and as a performance. Like I think there's lots of really interesting things to come out of that. So yeah, we'll see. We'll see where things will go. And of course I will also get to get to continue doing research. So I'm excited. You know, I'll be working with undergrads as well. And um, yeah, so I'm excited to see where I can take, where I can take this research with kind of this new injection of energy and enthusiasm from, from awesome students. So yeah, it's going to be fun.
Speaker2:
That sounds really exciting. And we'll have to check in in a few years and see where your research has gone and also which classes and creative artistic computer science classes you've incorporated at the university. And as always, this conversation, of course we could continue on, but we are unfortunately at time and I did want to point out, because this took me a second to wrap my head around, so I did figure out why this conversation feels full circle, because we began the conversation by describing how we use our senses to collect data. And then we finish the conversation by describing how we interpret collected data through our senses. So it begins and ends with our senses. There we go. That's a full circle moment. But anyways, thank you so much.
Speaker3:
I love it.
Speaker2:
Yeah, Well, thank you so much, Jordan, for, for coming on and for describing your research to us for the amazing work that you are doing and that you will continue to do at the at Whitman College in January. And, um, congratulations on finishing your dissertation.
Speaker3:
Thank you. It's been fun.
Speaker1:
We want to thank Jordan again for joining us today and for this wonderful conversation. Jess, what what are you thinking about? Sonic's sensory data, creativity and sonification? I just love talking about.
Speaker2:
My my sixth sense. The sense where I can see dead people is what I'm thinking about.
Speaker4:
I.
Speaker1:
I almost I almost referenced the movie after you said that. And then I didn't feel like it was in the movie.
Speaker4:
But it still it came out anyway. So.
Speaker2:
Okay, I'm thinking about something that we did not bring up in the conversation, but that it sort of came to my mind when the conversation was over. That I think is fascinating. So on this show, we talk a lot about AI and machine learning, because The Namesake, the show is called Radical AI. Even though we talk less.
Speaker4:
And less about AI through two years.
Speaker1:
Go on. Every everything you're saying is absolutely true.
Speaker4:
So I try sometimes I try.
Speaker2:
To like bring these topics back towards AI whenever we don't talk about it explicitly in the episode. So after this conversation, I was like, okay, how can I bring AI into this? Like, what does this have to do with AI? And I was thinking something that is fascinating to me about this whole like sensory experience of data is that. One of the things that separates us from the most advanced AI is that we have the ability to take data in from all these different kinds of senses, all 5 or 6 of them, right? But I only has the ability to use one sense to take in data, and that's just text. And it's not even a sense. It's just like all the, all the inputs, all the data inputs that it is processing are just text based. And whether we're processing an entire database of data or whether we're we're feeding in a bunch of images that are then converted to RGB values, that's still text or whether we're feeding in videos that are converted to a bunch of screenshots of images that are converted to RGB values. That's just text or whether it's sounds or smells, it all has to be reduced to text. And that's how AI functions, is it takes in all this data, but it's just text. And then humans are just so much more complex. Like, I wonder if our brains are also like converting it to some textual kind of data type. But either way, like sounds don't feel like text to me. You know, smell doesn't feel like text visuals don't feel like text to me. I just thought that was like a cool separation between us and the robots or us and the AI machines. That doesn't really have.
Speaker4:
Much to do with what we were talking about this conversation, but it's definitely related.
Speaker1:
That was that was the original goal of this podcast, is to separate us from the machines, figure out exactly what what is different between us and machines. I I'm going to I'm going to take I'm going to take issue with one thing you said about the reduction and like the reduction of oh, okay, please do data. And also of I guess everyday life experience into AI things.
Speaker4:
Ai is an official adjective.
Speaker1:
That's right. Um, but I think that that's one perspective and a legitimate perspective. But I also think maybe it's just a matter of like interpretation. Maybe it doesn't have to be a reduction, but maybe it's just a translation. Um, and so I think it to what Jordan was saying, right? I think it kind of depends on what your goal is. And so maybe it's not a reduction of lived experience, but it's just a different way of representing the experience to go then do something which we should be intentional about. But I'm thinking about this image that I saw earlier today in my. Silly academic world about like the history of this particular discipline, but it was all about how it was about the metaphor of the mind and the metaphor of like the human brain using that as a basis for understanding how technology works and how like silicon chips work and how there's all these different senses that are happening and we can think about them as all of these things happening in parallel, these processes happening in parallel, in the same way that you might think about a technological system, whether that's hardware or maybe software. And so I'm thinking about that a lot during like, well, reflecting on this conversation in well, maybe. I is not a reduction in that sense, but is an extended metaphor about how we are playing with data in new ways. That's what I'm thinking about today.
Speaker4:
Interesting.
Speaker2:
Interesting. I think, like, I agree with you in part and I disagree with you in part. I do like the idea of reframing. The idea of collecting data as it doesn't always have to reduce something to a number like data collection is not maybe inherently reductionist, but I do think that at least the way I've experienced it the majority of the time, it feels reductionist. And I was also actually when I was reflecting after the conversation about Jordan's question to us, which was like, how do we define data? Something else came to my mind that I didn't say during the conversation that I think is related to this too, and that is that I think of data as like a snapshot of a moment in time of something in a moment in time. And so like taking like a photo of something that is a form of data. And so like extrapolating that metaphor, that idea of a photo and now thinking of like text and databases or like words written on a page, that's also data too, but it's just a snapshot of, of the full picture. It's not ever actually the full picture. And so because we can never actually fully gather all of the data that is happening in a given moment, in a given place at a given time in space and the space time continuum, then no matter what, these snapshots will always be just a part of the whole. They will never actually be the whole. And so because of that, I do wonder if data is inherently reductionist, because no matter what we are, we are attempting to take something that is much larger and reducing it to a smaller understanding of that larger thing or a smaller description of that larger thing. And maybe, maybe.
Speaker4:
The.
Speaker2:
Next task here is to sort of like reframe our perspective on what it means to reduce something. Like maybe data being reductionist doesn't necessarily have to be bad. Maybe it just is, even if it is sometimes bad.
Speaker1:
Again, I agree with almost everything that you said. I don't know if data has to be. I think you're right about it being reductive of some sort of reality that it's breaking it down so that it can be communicable. So, like in the same way that Jordan was talking about how there she didn't use this word but like there's there's certain ineffable things or certain things out in the world like maybe like love or like sound that are not easily translated into something that has language that there are ways in which. Data can serve as a as a translation into that, even though they're impossible or difficult to describe. In that same way, I think that it doesn't have to be just one moment in time that's being represented by data, but it can be a series of moments or maybe it's a story, or maybe you're talking about just one data point. But even then, through multiple data points, it doesn't have to be a single moment in time, but it can be more of an evolving narrative. And so I'm thinking about Jordan's example of the finances and the markets of how there's all these little like beeps and boops that kind of which was which was actually, I believe the official.
Speaker4:
Terminology is the beeps and boops.
Speaker1:
I believe Jordan's dissertation was was entitled Beeps and Bloops. I believe it.
Speaker4:
Was bleeps and bloops, maybe bleeps and bloops. We'll have to check that. Yeah, that's.
Speaker1:
Right. Put it in the show notes or something. But but the the sound. Right. That are being interpreted or translated, I guess, from these different data points to these different moments in time that then become one sound that is like transforming over like a new amount of time that you're actually hearing it live and maybe it's recorded. And so then it's like interpreted both like during the recording, but then also so I just think that there is just more than data being a reduction into a single moment of time. I think it can be and maybe what you're talking about the collection point, but I don't know if it always has to be seen that way.
Speaker2:
Yeah, I do agree with you. And I also agree that data is like more often than not temporal, like it does exist over time. It's not just a single snapshot or moment in time, but I do still stand strong to it being a reductionist thing. I do think that like life is a soup or what is something that can be reduced, like a simple syrup. Life is like a life is the water and the sugar. And however you make simple syrup and and once we reduce it down to something else, it cannot be brought back to what it once was. And I think if we were just talking about it being translated, then life would be more like, I don't know, data would be more like the the precipitation life cycle where like water can become ice and then it rain and gas and liquid and all the different forms. But it doesn't it's not like a zero sum game. Like once it becomes one, it's not like it can't become another thing. Again, I think this is getting too philosophical.
Speaker4:
But I think that that we.
Speaker2:
Can agree to disagree on this one. Maybe.
Speaker1:
No, I agree that it's a reduction. I don't know how much I agree with the with the cooking reduction metaphor in this, but the.
Speaker4:
Metaphors were a bit of a stretch today.
Speaker1:
No, this is this is this is good content. But but I think that's I think that's we do really appreciate Jordan joining us It's always really nice to have close colleagues on the show. We haven't really done that for a long time, actually. And so it's nice to to go back to our roots of the first few episodes with some with some close colleagues of ours. Anyway, for more information on today's show, please visit the episode page at Radical Talk.
Speaker2:
If you enjoyed this episode, we invite you to subscribe Rate and please review the show on iTunes or your favorite Podcatcher. If you have not yet left us a review, you can catch our regularly scheduled episodes on the last Wednesday of every month with the exception of next month, December 2022. We are taking some time off to enjoy the holiday break, but we will be back to hit the ground running in January of 2023. And until then, you can join our conversation on LinkedIn, the Radical AI Podcast. Or we still have a pretty large community on Twitter at Radical Pod, but we totally understand if you are not on Twitter anymore and who knows, maybe we'll be joining some of the other alternative platforms in the future soon. But you can stay tuned for all of that. And until then, as always, stay radical.
Speaker1:
Oh, and happy holidays and happy holiday years. Stay safe out there.
Yeah, for sure. It.
Sonix is the world’s most advanced automated transcription, translation, and subtitling platform. Fast, accurate, and affordable.
Automatically convert your mp3 files to text (txt file), Microsoft Word (docx file), and SubRip Subtitle (srt file) in minutes.
Sonix has many features that you'd love including automated translation, world-class support, upload many different filetypes, automatic transcription software, and easily transcribe your Zoom meetings. Try Sonix for free today.