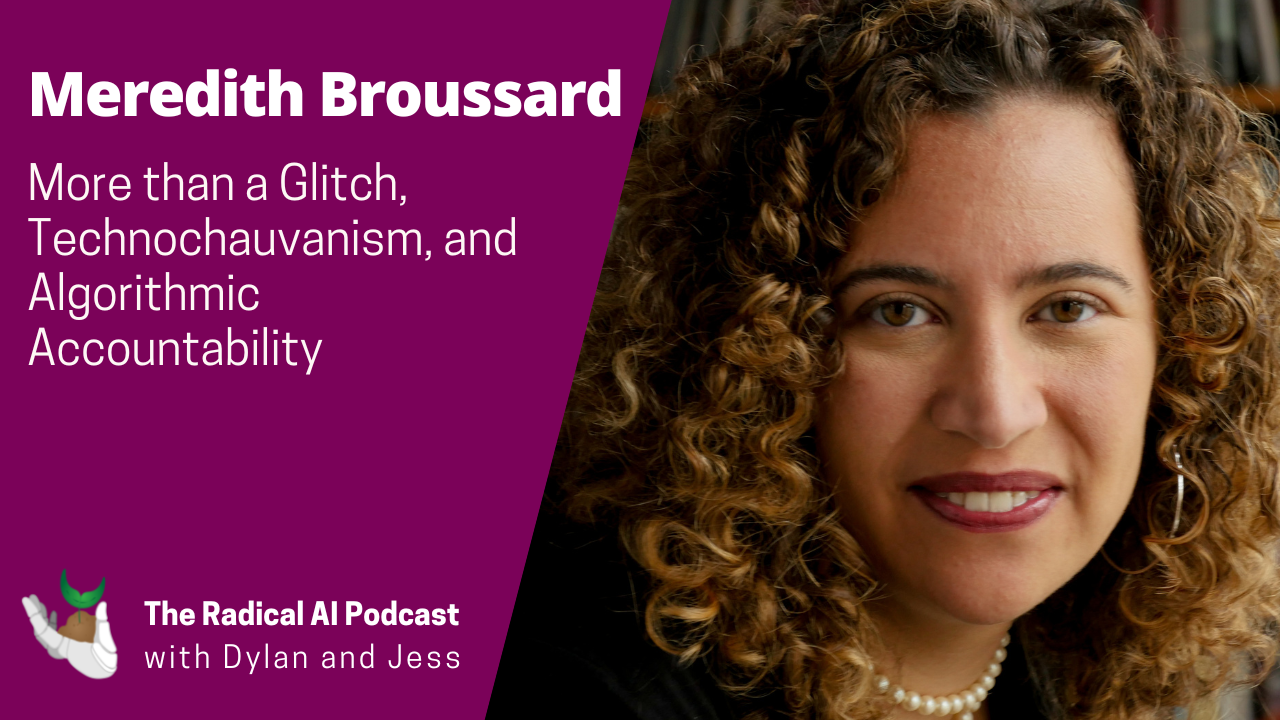
In this episode, we discuss Meredith Broussards influential new book,
More than a Glitch: Confronting Race, Gender, and Ability Bias in Tech – published by MIT Press.
Meredith is a data journalist, an associate professor at the Arthur L. Carter Journalism Institute of New York University, a research director at the NYU Alliance for Public Interest Technology, and the author of several books, including “More Than a Glitch” (which we cover in this episode) and “Artificial Unintelligence: How Computers Misunderstand the World.” Her academic research focuses on artificial intelligence in investigative reporting and ethical AI, with a particular interest in using data analysis for social good.
Follow Meredith on Twitter @merbroussard
If you enjoyed this episode please make sure to subscribe, submit a rating and review, and connect with us on twitter at @radicalaipod.
Relevant Resources Related to This Episode:
Use Code READMIT15 for 15% off the book at checkout if you buy the book from the button below, and ship it to a US address!
Artificial Unintelligence by Meredith Broussard
Google ‘fixed’ its racist algorithm by removing gorillas from its image-labeling tech
Using A.I. to Detect Breast Cancer That Doctors Miss
Industry AI Ethics 101 with Kathy Baxter (Previous Radical AI Episode)
Salesforce AI Ethics Maturity Model
An algorithm that screens for child neglect raises concerns
Inside TikTok’s Algorithm: A WSJ Video Investigation
Mozilla Open Source Audit Tooling (OAT) Project
Responsible Tech Job Board (All Tech is Human)
Coded Bias, AI, and the Future of Civil Rights (Previous RAI Episode with Meredith Broussard) in Partnership with All Tech is Human
Transcript
more-than-a-glitch.mp3: Audio automatically transcribed by Sonix
more-than-a-glitch.mp3: this mp3 audio file was automatically transcribed by Sonix with the best speech-to-text algorithms. This transcript may contain errors.
Speaker1:
Welcome to Radical Eye, a podcast about technology, power society and what it means to be human in the age of information. We are your hosts, Dylan and Jess. We're two PhD students with different backgrounds researching AI and technology ethics.
Speaker2:
In this episode, we interview Meredith Broussard about her newly released book. It's titled More Than a Glitch Confronting Race, Gender and Ability Bias in Tech, published by MIT Press.
Speaker1:
Meredith is a data journalist and associate professor at the Arthur L Carter Journalism Institute of NYU, a research director at the NYU Alliance for Public Interest Technology and the author of several books, including More Than a Glitch, which we cover in this episode and Artificial Unintelligence How Computers Misunderstand the World. Her academic research focuses on artificial intelligence in investigative reporting and ethical AI, with a particular interest in using data analysis for social good.
Speaker2:
Also, for those of you who are looking to read this book and to buy this book, first of all, we highly recommend it. It's amazing. We literally speed read it over the course of like 2 or 3 days before this interview. But second of all, we have a little surprise for you. So for the first time ever on this show, we have a special discount code for you to buy this book. And this was graciously provided by Meredith. So for listeners who have a mailing address in the United States, if you go to W-w-w dot Penguin, Random house.com, you can buy Meredith's book for 15% off if you use the code red MIT 15 at the checkout and that code is in all caps. You can also find all of this information on the show notes page for this episode, which, by the way, if you were not aware, we have show notes for every single episode that we do on this show and you can find them on our website at Radical Eyeborg. On the show notes pages, we have things like discount codes to books now, and we also have relevant links that are brought up maybe during the interview or also just related to topics that we discuss during an interview and during an episode.
Speaker2:
And we also have a summary of the episode and a transcript where you can read the episode and other links to view the episode or to listen to the episode on platforms or view the episode. Actually, we also have a YouTube channel. So yeah, all of this is available for every single episode. And if you're wondering where to find this, if you go to our website, Radical Eyeborg on our homepage, you can scroll down on the homepage and there is one carousel for episodes with guests where we have just one guest that we interviewed. And then if you keep scrolling, there's a carousel for interviews that we've done with multiple guests as well, and you can just click on the image and it'll take you straight to the show notes page. All right. So now that I've waxed poetic about our website for long enough, we've kept you from this interview for far too long. So without further ado, we are so excited to share this interview with Meredith with all of you.
Speaker1:
We are on the line today with Meredith Broussard to discuss her new book, More Than a Glitch Confronting Race, Gender and Ability Bias in Tech, published by the MIT Press. And we are so excited to have Meredith. We've heard so many different things about the book and now we've read it ourselves. And just it's it's so good. It's so good. We won't spend the entire hour saying how good it is, but like, it's so good. And so I think the first question, why this book and why now?
Speaker3:
So thank you so much for having me. And if you did actually want to spend the whole hour talking about how good the book is, that would actually be totally fine with me. Just, you know, for the record. So again, thank you for having me. Hi, listeners. This book came about on the heels of my last book, Artificial Unintelligence How Computers Misunderstand the World. And after Artificial Unintelligence came out, I found myself having a lot of conversations with different audiences all over the world. And the topic that we kept coming back to was the intersection of technology and race. And we also talked a lot about the intersection of technology and gender. And I started thinking more about the intersection of technology and disability, and I realized that I had all of these things to say about the intersection of these three things and technology and I wanted to explore them in a longer format. So here we are. The new book is called More Than a Glitch Confronting Race, Gender and Ability Bias in Tech.
Speaker2:
And speaking of that title, let's cover the first half more than a glitch. I'm curious what you meant by Glitch when you titled the book with this word and how it relates to the world of tech ethics and responsible technology.
Speaker3:
Well, a glitch implies something ephemeral, right? A glitch is something that is a blip. It's a momentary it is easily fixed in the code. Whereas a bug is something that is more kind of more intense. A glitch is something that you think, oh, we'll just fix that. That's not a big deal, whereas a bug is a problem. And what I felt was that people tend to treat bias inside technical systems as a glitch. So when we have a case like Google Images labeling photos of black men as gorillas, that gets treated like a glitch. Oh yeah, something will just fix in the code that's know that's not a big deal. Or when Microsoft bought, I started spewing anti-Semitic rhetoric on Twitter or when Chatgpt does something like generate text that seems like it's grooming a teenager for a child predator. These things get treated like, Oh yeah, that was totally unexpected, but actually there's no problem. We'll just fix it. And I'm arguing that actually we are at a point when we should be more sophisticated in our understanding of technology and our understanding of the way that social problems manifest inside technology. And so I urge readers to use a frame that was given to us by Ruha Benjamin in her book Race After Technology. And that's the idea that technology and automated systems discriminate by default.
Speaker1:
One thing that's coming to mind for me, as you're mentioning Chat GPT specifically, is last month we talked with Emily Bender and Casey Fiesler. And one thing that Casey mentioned is the magic that gets seen, basically gets read into chat GPT. Like, Oh, we have this tool, Oh my God. It's like this thing that we know how it works. We don't know how it works. And it's just like it's really cool and we can just dive into it and use it. And one of the examples towards the end of your book you gave was your computer science teacher. I think maybe back in undergrad talking about the magic of, of technology, the magic of coding. And it, it is neat, right? It's like all this technology, even like the the glitches are kind of this this weird magic. And I'm curious for you in thinking about this framing of maybe how we get away from that framing of magic to see technology more of of how it is in producing injustices, etcetera.
Speaker3:
I mean, I would really love to see the the rhetoric around technology equals magic. Like I would love to see that disappear because it is really a distraction when people think about themselves as magic because they're making technology or they think of themselves as wizards, it contributes to kind of an inflated sense of one's own power when it comes to technology. And I think we need to add nuance to that. We need to understand that our black boxes of automated systems are not actually impenetrable. But they can be understood. But it takes a fair bit of computational literacy to do that. So one of the things I'm really invested in is empowering people around complex technical topics so that people can push back when algorithmic systems are making decisions that are unfair. I. In the book, I tell the story about a time when I was an undergraduate and I was really struggling to understand something in my computer science class. And the professor got really frustrated with me and said, okay, listen, just pretend it's magic. Like, just just do it. Just do the thing that I said. And you don't have to understand why. Just pretend it's magic and it'll work. And so I did. And, you know, it did work because it's a pretty mundane technical concept. Uh, but I felt really dismissed in that moment, and I could tell that the professor just wanted to get rid of me. He wasn't invested in me learning a thing. That definitely happens. But it's not it's not ideal.
Speaker2:
It's interesting hearing you say this. You're reminding me of when I was first getting into the discipline of what will keep calling tech ethics. I guess today there's this concept called the engineering mindset, where an engineer is taught to ask the question, How? How do we build this? How do we create these technologies? And the philosopher instead is taught to ask, why? Why do we create these technologies and what is the impact? And that was something that I was grappling with as like a budding computer scientist at the time was, Oh my gosh, I'm being taught how to do all these things, but I'm never being taught how to ask why? Why are we are doing these things? And I'm curious from your perspective, because one of the things that I loved so much about this book, as you were just saying, is just the the the writing style that you chose was really catered to any kind of audience of any technical background. And I find that so refreshing in this space because for some reason, technologists have this stereotype of like, Oh, we've learned these really complex technologies and now we have to like gatekeep so that only those who have put in the work to learn how these technologies work are allowed to be invited into the conversation. And I'm just curious what you think the role of like critical algorithmic literacy plays in and gatekeeping plays in our ability to ask these questions of why and to critically interrogate our technologies.
Speaker3:
I'm so glad you brought this up because there's so much gatekeeping in tech. I remember that when I was when I was a budding technologist, when I was just learning, I was always made to feel small and I was always made to feel stupid for not understanding something. And nobody ever said to me, Hey, well, listen, this stuff is actually hard to understand and so just keep at it. You're doing fine. Just keep hammering at it. I which would have been a more helpful thing than. Just making me feel dumb because, you know, everybody learns at their own pace. And this, you know, technical stuff is not impossible. It's just a little challenging. Uh, and the, the normative ethic inside tech is also is definitely worth examining. I wrote about this a little bit in artificial intelligence, and I continued in more than a glitch with this idea of techno chauvinism, which is a kind of bias toward technical solutions. And the idea that you would go into a meeting at any big tech company and say like, Oh yeah, I think we should do this without technology. Like, I think there's a better solution that doesn't involve building a computer program. Like nobody would do that, right? You don't. Walk into an engineering school and point out that, oh, hey, I if we are trying to get books to kids or if we're trying to get learning materials to kids in rural areas, like maybe it's not a good idea to like give everybody iPads and ebooks because there's no connectivity. Like maybe it's a better idea to do paper books, right? So techno chauvinism can get in the way of good decision making. What I argue instead is that we should use the right tool for the task, and sometimes the right tool for the task is a computer, and sometimes it's something simple like a book in the hands of a child sitting on its parent's lap.
Speaker3:
Right. One is not inherently better than the other. But you're right, we do get trained in things like the engineering mindset. Or there's another aesthetic that I write about a little bit called Elegant Code. So back in the day, computer programs had to be really small because memory was really expensive and computing was really expensive. And so you would refactor your computer programs down to the smallest, most elegant unit, and that is the dominant aesthetic still. I mean, if I look at code and it just sprawls all over the place, I'm like, Oh yeah, that's not, that's not elegant. Like that is 100% the way I was trained. And. So this kind of mindset leads to making some default decisions that are not necessarily in harmony with the way that society operates now. So a good example of this is the case of gender. When I was taught to write elegant code, I was taught that there were only two genders, male and female, and they should be represented as 0 or 1 as binary values. Now we know now, you know, this is we're now several decades past when I learned to code. And we know now that gender is a spectrum, but programmers are often still representing gender as just a binary. And instead, what we should be doing now is we should be making gender an editable field. We should make it something that the user can change by themselves without talking to customer service. We shouldn't represent it as a binary. We should represent it as text. Maybe there's a dropdown, maybe it's free text entry. There are politics to these kinds of seemingly mundane technical decisions.
Speaker1:
I think we'll get into some more case studies for techno chauvinism and dive even more into that term in a second. But one thing I'm reflecting on right now and also in reading the book was how much of your own story that you told of how much? There were a lot of I statements and really pushing, I guess, a narrative or telling a narrative, I should say. And I'm curious about that decision for you writing that book and almost the power of storytelling maybe for that computer computational literacy or. Yeah, just to talk more about weaving your own story within the book during the process of writing.
Speaker3:
So glad you asked. I don't usually get asked about this, so it's thrilling to talk about, uh, to talk about narrative craft. So I, I do this a lot. I write a lot in first person when I am writing about complex technical topics. And I do that for a couple of reasons. It comes out of a literary tradition called immersion journalism, which is a kind of descendant of a ethnography where the ethnographer is a participant observer, they're participating in a scene, and then they're also observing it and writing about it. Immersion journalists do this and immerse themselves fully in a situation, and what I do often is I build technology in order to explore. A phenomenon, and I take readers along on that journey, and there are some practical reasons for doing this. If I were to just write about the technology without any people in it, it would be really boring. Right. So we we kind of want to see people. We want to see characters moving around in a, you know, in engaging nonfiction. And so I put myself in because, you know, I'm there. I'm a convenient, convenient character and I am building the thing. So one of the stories that I tell in the book is I talk about when I took my own mammograms and ran them through an open source cancer detection AI in order to write about the state of the art in AI based cancer detection.
Speaker2:
And you just perfectly segwayed into what my next question was going to be, which was to ask for a, let's say, maybe sneak peek into what that story was and maybe how techno chauvinism proliferated in the medical industry and the techno industrial medicine complex we can call it, especially as it related to your your personal experience and your attempts to like gain access to data that maybe should have rightfully been yours, for example.
Speaker3:
Well, this whole this whole thing started when I got a mammogram. You know, routine medical care. Everybody get your mammogram. If it's if it's appropriate and appropriate for your age and what have you. Uh, and I saw on the scan a note that said this scan was read by an AI. And I thought, oh, that's weird. Like, I wonder what the I saw. And then I kind of forgot about it a little bit because I got diagnosed with breast cancer and that was terrible. And, you know, I went through the whole treatment and I am now fine. So I should preface it with that. I am fine. I received excellent medical care. I'm really grateful to all the doctors and nurses and medical staff who took care of me. Uh, but I couldn't forget this, this note on the chart. And so I decided to investigate why the AI had read my scans, what it saw, and what was the state of the art in AI based cancer detection. We tend to hear about AI and cancer as being right around the corner. There was an article in the New York Times recently about how people were using AI in Hungary on breast cancer. And reading the article, you got the impression that this was going to be happening everywhere for like next week. And it turns out the truth is way more complicated than that. I looked at some open source software because one of the ways you can understand proprietary software is by looking at open source software, because it's kind of made it's all made the same way, right? Same general principles apply.
Speaker3:
And when you're looking at an algorithmic system in general, we know from algorithmic accountability reporting, which is kind of journalism that I do, we know that you can understand an algorithmic system by looking at the training data and looking at the outputs and also reading the reading the documentation and reading the academic research about how the system is built. So I did this. I looked at some open source software, I looked at the training data, and then I tried to get a hold of my own mammograms in order to run them through. And I thought this was going to be easy because we have electronic medical records and, you know, people make a big deal about how portable data is. And it was not portable and it was not easy. So this is one of the reasons that I'm a little skeptical when people claim that there is like a bright AI enabled future for medical data around the corner. These systems are clunky and are not necessarily interoperable, and they're very, very fragile. Right. So I eventually got the data. I ran my scans through the the cancer detection AI. It's made by one of my colleagues at NYU. And it worked. It was really cool. It identified what I knew was a cancerous area, but I realized. That it didn't work the way I expected. So I expected. That it would be some kind of Grey's Anatomy type scenario.
Speaker3:
Like, I do write a lot about how our Hollywood ideas about AI are really deeply embedded, and I myself have really trained myself not to think about the Terminator when I think about AI. But it turns out that I think about Grey's Anatomy when I think about medical technology. And so I expected this moment to be like a really big reveal, and I expected it to be dramatic. And I thought there would I don't know, it just would be visually exciting. And it's really not like I just like took a flat image and ran it through the program. And then it drew a red box and that was it. So I had unreasonable expectations about the cancer detection system. And I realized, Oh wait, this is an unreasonable expectation that I got from. I you know, from reading about the promise of AI in the popular press. So I think we need to dial it back. I think we need to be more realistic about what AI can and can't do when it comes to cancer. It is primarily a tool that may or may not help doctors, and it is. More in its infancy than you might expect from the marketing literature that's out there. That said, I'm very impressed with a lot of the with a lot of the research being done in this area. And the research that I looked at specifically was a really good example of being being honest about what works well and what doesn't.
Speaker2:
Yeah, it's it's interesting. One of the things that really stood out to me when you were sharing this story in the book towards the end, after you got these results back and you were looking at that image in the red box was the numbers that were associated with the box. And you describe in the book, you get this like decimal 0.2 something, and you were expecting that to be a percentage of like likelihood that this was like malignant tumor, for example. And and then this conversation is sort of started around like, well, why wasn't it a percentage likelihood? Why was it just this like arbitrary decimal that I and now as the end user I'm supposed to interpret and I think this is a really a great opportunity to open us up to a conversation around like what outputs of these really high risk models should be. If, if engineers, computer scientists who are creating these technologies should have the responsibility to to make declarative statements with the outputs of their models, or if interpretation should always be left to the end users. And this could be like high stakes, like medicine. Or we could be talking about things like, you know, fairness and, and quantifying other really high risk theoretical, sticky subjects. What do you think the role of where do you think the role of responsibility lies in interpreting the outputs of these kinds of models?
Speaker3:
You know, I think I'm reluctant to make a sweeping statement about it. Like, I think I'm reluctant to say it should always be this or that because I think it depends on context. I with most of I, I think it depends on the context. So when I got the the results of this, of this AI analysis that I did on my own scans, as I said, it drew a red box and it gave me a it gave me this score and. And because it was a numerical score and because it had a decimal point in it, I immediately assumed, Oh yeah, this is a percentage, like this is a 20% chance that this is a that this is a malignant area that's been identified. And like this was another case where I was wrong. Like, so the way that I was wrong was extremely instructive. We have a lot of misconceptions about how AI works, and I was really interested to discover that this is a because of legal issues. Right. So the I can't output a prediction that, oh, there's a 20% chance that this is malignant, uh, because of the legal environment in which it operates. So that led me down a rabbit hole of kind of trying to understand the the legal and economic environment for AI in hospitals. So something else interesting is that we we do hear a lot about AI replacing radiologists, which is not something that I think is going to happen anytime soon. But you do hear it and people often talk about how AI diagnosis is going to be so much faster and so much cheaper. But hospitals don't get paid if an AI reads a scan and hospitals do get paid if a radiologist reads a scan. So there are these competing economic incentives that I definitely was not aware of until this project. And I think we're going to have to start having conversations that are also about like who's getting paid, who wins, who profits from AI in medicine, and what does this do to like the economic models that make health care.
Speaker1:
Proved effective outside of the health care sector. You also cover several other topics. And I think the way I want to frame this is in terms of the Hollywood idea that you referenced and like the stories that we tell about or that, you know, it depends on who the we is, But we'll use Hollywood as an example that Hollywood tells about about I and I'm curious what we're looking at, either race or gender or ability bias other ways that you're seeing that Hollywood idea play out in technology design and maybe how you're seeing ways that we can combat that or rightsize that to the reality that we're actually living through.
Speaker3:
I think that Hollywood is our default. In part because Hollywood stories are so well told. We feel like I the Star Wars universe, for example, is real. We feel like it exists. And you can go down to Florida or over to California and actually spend time pretending that you're living in it at Disney World. Like it's it's very vivid, right? So we need to make sure to operate in the world of what's real when we're thinking about AI. And we need to not get it confused with science fiction. There has been for a very long time, like a kind of long standing initiative to make science fiction real. And you see this in people who want to go live on other planets or people who want to make ray guns or want to make teleportation devices, which are all incredibly fun to think about. And I completely applaud the creativity of. You know, of wanting to make imaginary stuff real. But I think that we are at a point. Technologically speaking, when we need to be practical about these things as well. And we need to reflect not just on can we do something, can we build it, but should we build it? So I am really delighted that there is a greater conversation happening in corporate America about AI ethics and there is a conversation about responsible AI governance happening.
Speaker3:
One of the things I read about in the book is Salesforce, which has an AI ethicist named Kathy Baxter on staff, and Kathy Baxter has done this diagram that shows exactly where a bias audit can exist inside an existing corporate process. So one of the excuses people often make when they're building technology is, Oh, we don't we don't really have time to audit this technology for bias. And what that actually means is we don't want to do it. But if you integrate it into your regular business processes, there's plenty of time to do it. And if you start looking, you're going to find problems, right? Technology is biased. Technology includes the unconscious biases of its creators. Right? This has been an open secret for a really long time. But it's the open secret that I explore in the book. And we really just we need to talk about it. And we need not be afraid of talking about it because confronting it is really the only way that we're going to make any kind of progress. And it's going to be a tough conversation. Those tough conversations will have to happen over and over again. It's going to require collective solutions. It's going to require a lot of kindness. And, you know, I hope. Like. I hope we're up to the challenge.
Speaker1:
One thing that I think we see in different contexts is that bias, as a word, has some linguistic slippage of people mean different things in different spaces. And the same thing with fairness. I'm stepping on justice toes a little bit as the person who studies fairness, as a as a as a scholar, I do something very different. But I'm curious for you because I know you have a whole chapter in this book also about bias in a machine bias, understanding, machine bias. And I'm wondering for you how you understand bias and perhaps fairness and whether it's the kind of the two sides of the same coin or whether those are two totally different concepts.
Speaker3:
It's a good question. I'm going to go back to the idea of context.
Speaker4:
I.
Speaker3:
I write in the book about fairness and bias in the context of technological systems. And I also tell a story about the difference between mathematical fairness and social fairness. And one of the ways I understand the distinction is by thinking about a cookie. So when I was a kid and there would be one cookie left in the kitchen, my brother and I would fight over who got the cookie. And my brother is younger than I am. And so I you know, there were just well, there was just fighting. So if you were a computer and you were confronted with this as a word problem, you know, two children, one cookie, the computer would say, oh, well, you cut the cookie in half, each child gets 50%. And that solves the problem. And that is absolutely true. It is a mathematically fair way to solve the problem. But in the real world, when you break a cookie in half, there's a big half and a little half. And so if I wanted the big half, I would say to my brother, All right, you give me the big half now and I will let you pick the show that we watch after dinner. And my brother would think for a second, he would say, Oh, yeah, that sounds fair. And it was it was a socially fair decision. And so I think when we are talking about using technology to solve problems, we need to be clear about are we looking for a mathematically fair solution or are we looking for a socially fair solution? And if we're looking for something that's socially fair, we should really be cautious about using computational systems for this, because computers can only. Calculate mathematical fairness. Right. So computers are not the best tool for solving social problems alone, right? We're not going to be able to code our way out of social problems. Computers can be a really good tool for humans to use, but we shouldn't expect that we transfer decision making over to autonomous computational systems, and that will somehow be better than, you know, than human problem solving.
Speaker2:
Totally agreed. And it's interesting, too, in some of the research that I've done on algorithmic fairness, it it seems like a lot of I'll just say like computer scientists as a broad generalization, but the people who who love to audit systems for fairness mathematically more recently have begun to attempt to auditing these systems for like social fairness, for example. But it it is there is this question of like, is it possible to even audit a system for something that is, you know, non-empirical non-observable not not quantifiable, perhaps not reducible to a number. And so I'm just curious about this, this topic of auditing generally. And you mentioned earlier that one of the things that you do in your work is this algorithmic accountability reporting. And I'm curious if you could just perhaps describe to us what that is and how that influences your work.
Speaker3:
So algorithmic algorithmic auditing is, I think, just one of the most exciting, exciting new fields in computer science. It is. I mean, I am just I am thrilled that it exists. And I, I love seeing all of the amazing projects that have that have bloomed in the past couple of years. So let me back up. So algorithmic accountability reporting is a is a subtype of data journalism. In today's world, algorithms are increasingly being used to make decisions on our behalf. And one of the traditional functions of the press is to hold decision makers accountable. So in the modern world, the accountability function of the press transfers onto algorithms and their makers. So we have algorithmic accountability reporting, holding algorithms and their makers accountable. Sometimes that means opening up the black box of an algorithm and examining what's going on. So the journalistic project that kicked this all off is a project called Machine Bias by Julia Angwin, formerly of ProPublica, formerly of the Markup. And what that investigation found was that there was software used across the country to estimate the risk of recidivism, the risk of reoffending, and the software was biased against black people.
Speaker3:
Propublica released the released the data that they use to evaluate the system, and it caused an absolute flourishing of interest in the topic. It facilitated I our new understanding of mathematical dimensions of fairness. And one of the interesting findings was a mathematician, computer scientist went in and discovered that, oh, there's actually no way for this algorithm to be to be fair to both white people and black people. Right? So this was kind of what kicked off algorithmic accountability reporting. Recently, there have been some really amazing investigations that came out. There was one from the markup about an algorithm used in LA to allocate homes to people who are unhoused. And they found found, I believe it was racial bias inside that algorithm. There was a story that came out in Wired recently in collaboration with Lighthouse reports about an algorithm used in the city of Rotterdam to allegedly detect welfare fraud. Well, this was biased based on ethnicity and gender. Uh, there's another investigation of an algorithm used in a.
Speaker5:
What is it in like child.
Speaker3:
Child safety. Something should fact check me on that. Ap investigation looking at an algorithm used in providing some kind of public service that they found bias in AI. So it's great that there are all these investigations happening because it's, you know, bringing clarity to unfair systems. I would really prefer if the unfair systems didn't exist in the first place. But I'm glad that as reporters, we have tools to do this. And so. Sometimes algorithmic accountability. Reporters open up black boxes. Sometimes what we do is we write our own code in order to investigate things. I do this sometimes. There is a Wall Street Journal investigation about the TikTok algorithm. That's another example of this, where they they built a lot of bots and had the bots. Quote unquote. Watch TikTok videos in order to understand how the TikTok algorithm works. And then they did this terrific explainer about how the TikTok algorithm works. So I sometimes we investigate other people's code. Sometimes we read our own code. And it is very much related to. Uh, algorithmic auditing. So algorithmic auditing comes from the world of compliance, which if your eyes are starting to glaze over at this point like that, is, that is not unusual. People never want to talk about compliance. I totally understand. But it's actually sort of interesting. Uh, so the idea behind algorithmic auditing is that we can take the inputs and the outputs of the system. We can look at the model, we can look at the code, and we can apply. You know, common sense reasoning or, you know, definitions of mathematical fairness. And we can evaluate whether the system is biased against different groups. If so, how much and and does it matter? All right. So we have lots of tools for this. Now, there's something new from Mozilla that Deb Raji has put out. It's called OTX, the Open Access Toolkit for algorithmic auditing. There's something that came out from IBM a few years ago called Fair 360, and there are lots of other open source, open source toolkits for auditing systems.
Speaker1:
We have a lot of folks who listen to this show who are researchers in the academic space and the industry space. A lot of students as well who are on board. Right. They're on board with what you're saying. And they're thinking, well, how do I do the work in order to, like operationalize this in a research capacity? So, for example, myself, I do a lot of work with oncology and end of life planning, and I use a lot of ethnography in order to say, okay, well, technology, how can technology be effective? How can it not be effective? And then what are the social needs of people in addition to the tech needs and I'm still thinking like.
Speaker6:
Well, these like.
Speaker1:
Chart notes from the 90s on this 90s interface. I want to do something about it. I want to make some sort of implementation in terms of fairness, in terms of bias, but also in terms of like interface access. But I want to do really effective research to do that. And I think one thing that your book speaks to is whether it's algorithmic auditing or other examples you give are really powerful and effective interdisciplinary research opportunities for like the methods of actually doing this work in the first place. And I was wondering if you were to speak to the the researchers out there who are listening, how do you do this work? Well, how do you research these topics Well, or maybe what success have you found or failures, depending on how you want to frame it in doing this work?
Speaker3:
I'm so glad you asked that. And one of the challenges for me as a researcher has been that there isn't a kind of defined set of methodologies for doing interdisciplinary digital work.
Speaker7:
Right.
Speaker3:
So as a journalist and as a as someone who writes scholarly work in communication journals and as someone who does very interdisciplinary stuff, like I tend to to pick and choose my methodologies from whatever discipline has one that works for me. I have the freedom to do that. I don't think that every researcher has the freedom to do that because some disciplines are really orthodox about what you're allowed to do and what you're not allowed to do. So. I think that we are we are overdue for a. And kind of.
Speaker7:
Lots and lots of methodology.
Speaker3:
Methodology Papers about complex digital research. Uh, one of the resources I would point people to is something I'm involved in called the Center for Critical Race and Digital Studies. And there's a syllabus that is really, really wide ranging and has a lot of work from ethnography of Black Twitter to how do we how do we collect beauty tutorials and use them to understand, uh, you know, to understand cultural identity. Uh. So I would say definitely check out the work of of scholars. Um, and. I have a question for you, actually, which is what are some examples of really good use of technology in end of life situations?
Speaker1:
Well, you're asking the dissertation question and I'm probably going to defer a little bit. I think we're still trying to figure it out. And so my work is basically how do we create a tool as we create more and more data every year in our lives and we have so much that we leave behind to our loved ones after we die. And technology, say Silicon Valley, et cetera are not particularly known for developing for end of life needs and end of life technology for various reasons. How do we design tools that help people first think about it and reflect on what their data is going to be and then also what their wishes are and then how to communicate that to technology and to systems that will actually make those wishes executable or able to be fulfilled after death. And so there's there's many other, many other topics. But I don't want to take away from this particular conversation.
Speaker3:
That's super interesting because I definitely would not have thought about that. Like I immediately flashed to things like care bridge or meal train, which I think are are really, really useful for the, the kind of practical aspects of end of life situations because you do want to, you know, to take a meal over to somebody who is, you know, who's dealing with that and meal train helps you organize it so you don't have like a thousand casseroles showing up one day and then nothing, you know, the next five days. And Cambridge, it seems like is or systems like that seem really useful for keeping the community aware of what's going on with with somebody who's in medical crisis without having to burden the caregivers with like sending a million emails and texts and photos and what have you. So that's interesting. That's something I'll think about. Thank you.
Speaker2:
I think something else that you just highlighted in that response to was just how complex these technologies are When we attempt to interact between technology and a social system or when we attempt to solve I'm going to use air quotes here to solve social problems with technological systems. We we learn time and time again that these things cannot be solved purely through technological means if through any technological means at all. And I think we so often hear this really like negative dystopic rhetoric, at least in the like, AI ethics academic world that sort of just like burn it all down, don't make it in the first place. And I really appreciate it at the end of your book that you you end with this perspective of hope and the things that you're hopeful for in this discipline and surrounding all these themes. So I'm going to ask that question to you now in in this techno chauvinist world that we find ourselves embedded in. What is something that you are currently hopeful about?
Speaker3:
Well, I am really hopeful that researchers like you are going to help us get more insight into fairness and and help us figure out what the heck is going on. Uh, so that's one thing I'm optimistic about. Another thing I'm optimistic about is the field of public interest technology, which is exactly what it sounds like. It's making technology in the public interest. And so sometimes that means working on a government website to strengthen it so it doesn't go down when there's a pandemic and a million people file for unemployment insurance simultaneously. Sometimes public interest technology means doing algorithmic accountability, reporting and uncovering systems that are racially biased or ableist or gender biased or just unjust in some way. I.
Speaker7:
I'm very hopeful that.
Speaker3:
There are going to be more jobs in this sector going forward. One of the things that we did at the NYU Alliance for Public Interest Technology, which is a group I'm part of, is we sponsored a career fair with all tech as human, where we brought together people who.
Speaker7:
Are.
Speaker3:
Offering jobs in this sector. And all of that material is recorded and archived so students can learn more about job opportunities in that field. And.
Speaker7:
I am really I'm really hopeful.
Speaker3:
Based on what I'm seeing in the classroom. My students are just terrific. They really understand these issues and they are interested in in building technology that is.
Speaker7:
That is not biased or that is not.
Speaker3:
Racist, that is not sexist, that is not ableist. And they're really interested in pushing to build technology to make it better.
Speaker1:
Well, Meredith, unfortunately, I think we are at time for this conversation. But thank you so much for joining us today.
Speaker3:
Thank you so much for having me. It was such a pleasure.
Speaker1:
And of course, in the show notes and all of the different links will make sure that you listeners have all the resources that you need both from this conversation and also to buy the book, underlining that the book is so amazing, go buy it now.
Speaker2:
We want to thank Meredith again for joining us today for this wonderful conversation. And as always, now is the time where we debrief our immediate reactions after the conversation. So, Dillon, what is immediately coming up for you right now?
Speaker1:
Yeah, it's just exciting to have Meredith on the show. I don't think we mentioned this in the intro, but years ago we had Meredith on the show as part of a collaboration with Alltech as Human, and it was really cool for us to now be the ones interviewing her and being able to chat and get to know her better and everything. So it's kind of a full circle like three years later kind of thing. That was that was really cool. I think the thing I'm still stuck with as a, I guess, a researcher who cares about these things and is thinking about how to frame them in new ways. So thinking about, say, fairness and bias and responsible technology and how we tell the story or stories of our research without replicating the Hollywood narratives, right? Without replicating the high flying techno chauvinism that has gotten us into this mess in the first place. And one thing that I asked Meredith about and that I just think a lot about is, well, what are the stories that we're telling about ourselves as researchers? Do we even tell our stories within our papers or our books or whatever? Do we treat technology as this objective thing that's over there, or do we name our identity? And then what are the ramifications of naming our identity? Do we get targeted for it? I'm curious about the stories that other researchers tell about researchers who disclose their identity or their positionality. Meredith brings up, you know, standpoint theory, which has a history in in feminism and disclosure of identity and how you can use that to upend power structures. And so I'm curious, as technologists or as people, researchers or people building tools, how we can leverage personal storytelling effectively and not in ways that just replicate existing systems of power and systems of oppression. So that's what I'm sitting with. Wow.
Speaker6:
Just that. Just.
Speaker1:
Just only that. Just what I know. We we come at this from from very different backgrounds. And I'm wondering. Yeah, what what's on your mind?
Speaker2:
Yeah. It's so funny after these interviews happen, especially usually like while we're debriefing, I'll like, think of new questions and new thoughts. And I'm like, Oh, I should have asked that during the interview. Dang it. I want to know Meredith's take on this, but I just had one of those moments while you were speaking where I was thinking about, you know, her narrative during the book and just some of the different stories that she tells. And I, I noticed that there was this sort of like duality that was emerging in the book where on the one hand, it's like we shouldn't be building some of these systems in the first place. Like technology does not have a place or should not have a place or should not have a seat at the table in some discipline, some domains, some circumstances. And then on the other hand, we have this narrative of like, okay, well, these technologies in some cases have already been made, or sometimes there's nothing we can do to stop these people from making them. Unfortunately, like that's just sort of the reality that we live in. And so in the event that these technologies are made, how can we effectively improve them? How can we effectively audit them, How can we evaluate them? And so that's sort of the tension that I'm grappling with right now.
Speaker2:
And I would love to know how Meredith thinks about this. Maybe we can get her take on this and then share it in a future episode or something. But I'm just I'm wondering, like to what extent should, um, you know, academics like us, researchers like us or even, you know, the, the, the casual technology user, to what extent should we be working within the system that we've been placed in and to what extent should we be like fighting to take that system down? And it sort of speaks to like the the topic and the theme of this show, too, right? Like this, this idea of like radical technology is to really like to to to question technology at its root and to to to critically question the systems that we've been placed in and perhaps to try to to shift them and to fundamentally change them before they have the chance to even cause harm.
Speaker6:
Yeah. One thing that you said, one question that.
Speaker1:
You asked or part of a question that you asked was about reducing the social systems to a technological perspective. And Meredith put me on the spot, you know, towards the end of the interview about like, well, what is it that you study and, and what's coming out of that research which, which I kind of freaked on.
Speaker2:
But I nobody ever asks us questions. We're never prepared. I know.
Speaker6:
Oh, my God.
Speaker1:
Is this what it feels like to be on the other side? But but that's a that's a question that I ask a lot about this death thing of what if technology isn't designed for that social thing at all right now and is now playing catch up to what, like thousands of years of entire human history of like, ritual that's been built up and whatever that like, what what can technology do at the end of life? Is it just logistical? What does it play in culture and in ritual broadly, religion, all these kind of questions come up that technology just maybe shouldn't be a part of, maybe should. And now it like there's no choice but for it to be. And so what do we do? And so I think that's the other question for me is like, well, for stuff like death or probably a lot of other topics, that there aren't social solutions necessarily. Maybe it's just like harm reduction or something for the techno chauvinism. Like what?
Speaker6:
Who is. Yeah. What are the what are the possibilities?
Speaker1:
And over the three years of the show, I think I kind of remember like three episodes in asking the same question. So I guess we're because we're, we're evolving but staying the same. Yeah. Much, much like technology. Possibly. Much like.
Speaker6:
Technology.
Speaker2:
No, it is interesting though. I mean, I think like the the language of solutions in the tech ethics space is always so fascinating to me. Like, how do you even grapple with trying to come up with a solution to something that is potentially inherently unsolvable? And the concept of coming up with the solution is just like so subjective and theoretical to like, how do you even know that you've solved something? Well, maybe you've achieved an arbitrary metric that someone set. Is that metric all encompassing and holistically, like representing the thing that we were attempting to solve in the first place. Does solution mean that everyone is happy with the outcome? Does solution mean that something that was broken is now fixed? How can you tell if it's fixed? And it gets even more complex when we're talking about like social issues where people disagree on basically everything that I just said. And so you might have some people who are like, Yes, this is fixed. I got the bigger half of the cookie, as Meredith was saying, and other people who are like, Hey, I got the smaller half of the cookie. No, this is not fixed. I'm not okay with this. And I just get so excited talking about these topics. They're so fascinating to me. That's probably a good thing, which is why I research algorithmic fairness work because, you know, there are no solutions. And and the whole concept of doing practical ethics work is is working within the constraints of a system where you know that there is no right and wrong, there is no good or bad, there's maybe better or worse. But even that is something that's so subjective that it's just it's such a complex space and such a really like rich research opportunity to really start to like interrogate. What do we even mean by solutions? What do we even mean by fair? What do we even mean by unbiased? And and I think that that's probably why we will continue asking these same questions in however many years it is as we continue to run this podcast. I don't think we'll ever stop.
Speaker6:
Well, and I think, Meredith.
Speaker1:
Are.
Speaker6:
Some of those.
Speaker1:
Co-conspirators, right? Is one of those co-conspirators with us of asking those questions? And so it's fitting that we're ending with more questions. I guess we always have more questions than.
Speaker6:
Answers, but maybe, maybe that's our job in this. I'm not sure I.
Speaker1:
I again, just want to we want to thank Meredith for coming on the show today. Please do read her book. We know that over the last episode with Casey Fiesler and Emily Bender and also this episode we, I guess we imagined for this episode we'll have a lot of new listeners. And so per Jess's plug at the beginning of this episode, please do. In order to find more information, please visit the episode page at radical ai.org.
Speaker2:
And and add besides the awesome discount that you can get from buying this book, if you do visit the episode page for this episode. If you enjoyed this episode, we invite you to subscribe, rate and review the show on iTunes or your favorite Podcatcher. You can catch our regularly scheduled episodes the last Wednesday of every month unless we have something really exciting come out like a book launch, which is what happened this time. So this is the second to last Wednesday of this month that we're releasing this episode. And you can join our conversation on Twitter at Radical iPod. You can join our conversation on LinkedIn at the Radical AI podcast. And as always, stay radical.
Sonix is the world’s most advanced automated transcription, translation, and subtitling platform. Fast, accurate, and affordable.
Automatically convert your mp3 files to text (txt file), Microsoft Word (docx file), and SubRip Subtitle (srt file) in minutes.
Sonix has many features that you'd love including world-class support, enterprise-grade admin tools, collaboration tools, automatic transcription software, and easily transcribe your Zoom meetings. Try Sonix for free today.